Rofi - The Use Of Repeated Optimization For Feature Interpretation
2016 International Conference on Computational Science and Computational Intelligence (CSCI)(2016)
摘要
High-throughput molecular profiling technologies have the capability to capture both global and targeted scale data for multiple biomolecules. In the case of biomarker discovery, building predictive models via machine learning has become a common strategy for evaluating these large complex datasets. In biology however, identification of a collection of features (e.g., metabolites) that work well in combination is vital to the identification of candidate biomarkers of interest for validation or to gain insight into the biological mechanisms associated with the outcome of interest. Thus, gaining insight into the value of the feature in the model is important for inferring relationships between the features and the phenotype. We present an approach that uses repeated optimization to enable improved feature interpretation (ROFI). ROFI is shown to return better or comparable results to existing methods for true negatives. Furthermore, application to a real world dataset associated with type 1 diabetes confirms that ROFI identifies metabolites with a clear connection to the disease in an easy to interpret format.
更多查看译文
关键词
biomarker discovery,feature selection,feature interpretation,optimization
AI 理解论文
溯源树
样例
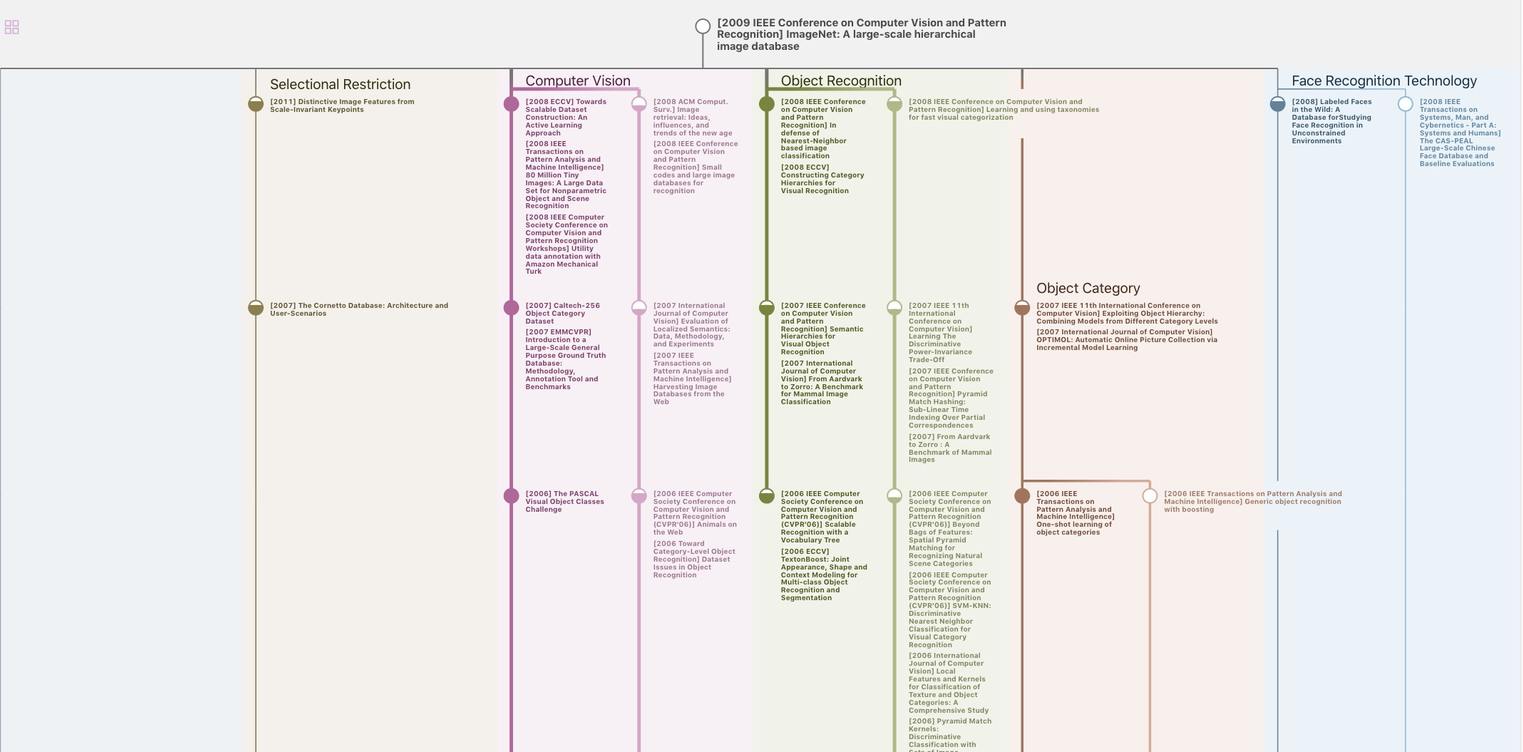
生成溯源树,研究论文发展脉络
Chat Paper
正在生成论文摘要