Adjusting for Dropout Variance in Batch Normalization and Weight Initialization
arXiv: Learning(2017)
摘要
We show how to adjust for the variance introduced by dropout with corrections to weight initialization and Batch Normalization, yielding higher accuracy. Though dropout can preserve the expected input to a neuron between train and test, the variance of the input differs. We thus propose a new weight initialization by correcting for the influence of dropout rates and an arbitrary nonlinearityu0027s influence on variance through simple corrective scalars. Since Batch Normalization trained with dropout estimates the variance of a layeru0027s incoming distribution with some inputs dropped, the variance also differs between train and test. After training a network with Batch Normalization and dropout, we simply update Batch Normalizationu0027s variance moving averages with dropout off and obtain state of the art on CIFAR-10 and CIFAR-100 without data augmentation.
更多查看译文
AI 理解论文
溯源树
样例
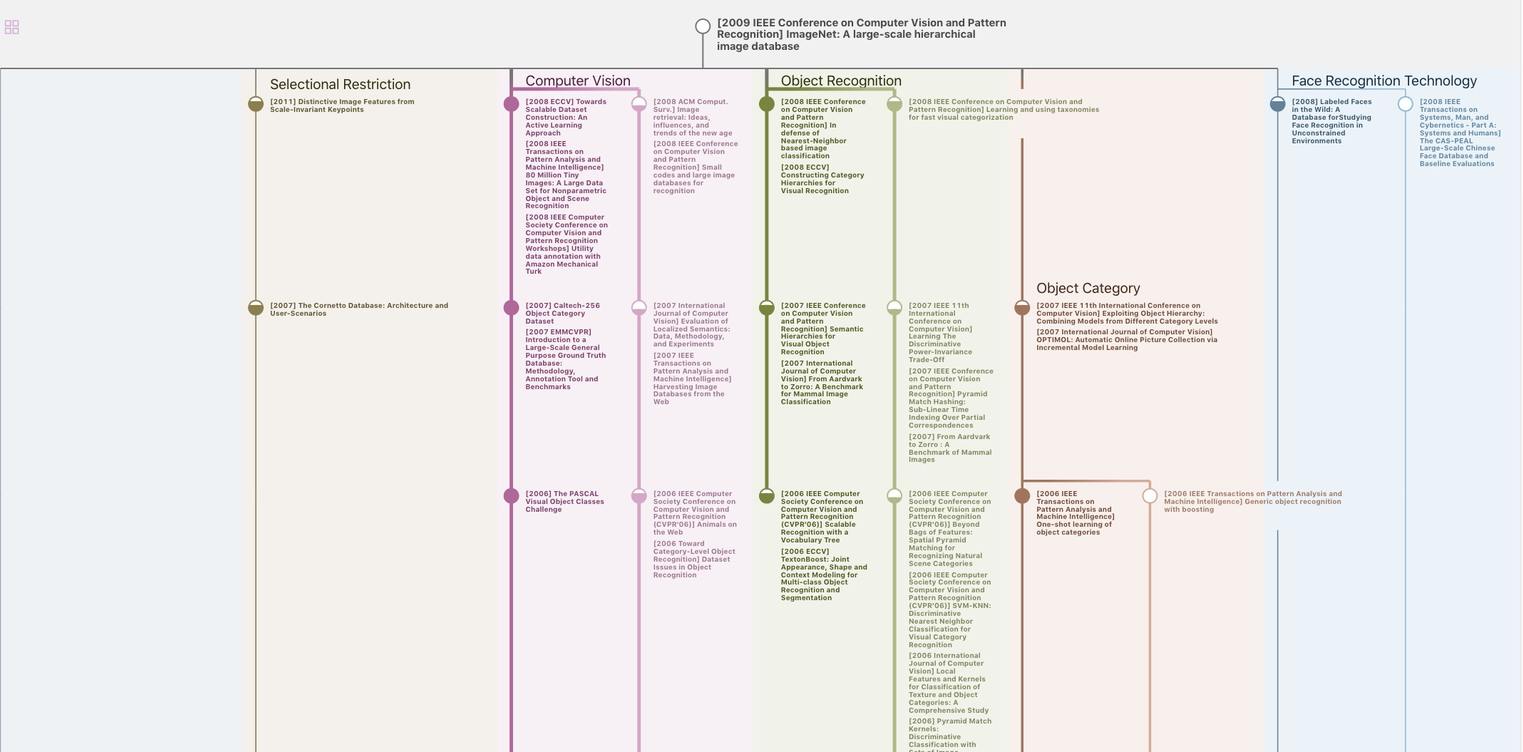
生成溯源树,研究论文发展脉络
Chat Paper
正在生成论文摘要