Myocardial Ischemia Event Detection Based On Support Vector Machine Model Using Qrs And St Segment Features
2016 COMPUTING IN CARDIOLOGY CONFERENCE (CINC), VOL 43(2016)
摘要
This study aimed to develop a nonlinear support vector machine (SVM) model to detect ischemic events based on a dataset of QRS-derived and ST indices from non-ischemic and acute ischemic episodes.The study included 67 patients undergoing elective percutaneous coronary intervention (PCI) with 12-lead continuous and signal-averaged ECG recordings before and during PCI. Fifty-four indices were initially considered from each episode. The dataset was randomly divided into training (80%) and testing (20%) subsets. The training subset was used to optimize the SVM parameters algorithm and for determining the most important statistically significant indices, by using repeated k-fold cross-validation (with N=25 repetitions and k=5). The described procedure was run on 25 randomized training/testing subsets to assess the average performance.On average, the most important indices were the QRS-vector difference and the ST segment level at J-point + 60 ms computed from the synthesized vector magnitude, and the summed high-frequency QRS components of all 12 leads at 150-250 Hz band. The performance of testing was: classification error=12.5(8.3-16.7)%, sensibility=83.3(75.0-91.7)%, specificity=91.7(83.3-91.7)%, positive predictive value=90.9(83.0-92.3)% and negative predictive value=85.7(80.0-91.7)%. The method used to construct the SVM model is robust enough and looks promising in detecting acute myocardial ischemia and myocardial infarction risk.
更多查看译文
关键词
myocardial ischemia event detection,support vector machine model,QRS segment feature,ST segment feature,QRS-derived index,ST index,nonischemic episode,acute ischemic episode,percutaneous coronary intervention,ECG recording,QRS-vector difference,acute myocardial ischemia,myocardial infarction,frequency 150 Hz to 250 Hz
AI 理解论文
溯源树
样例
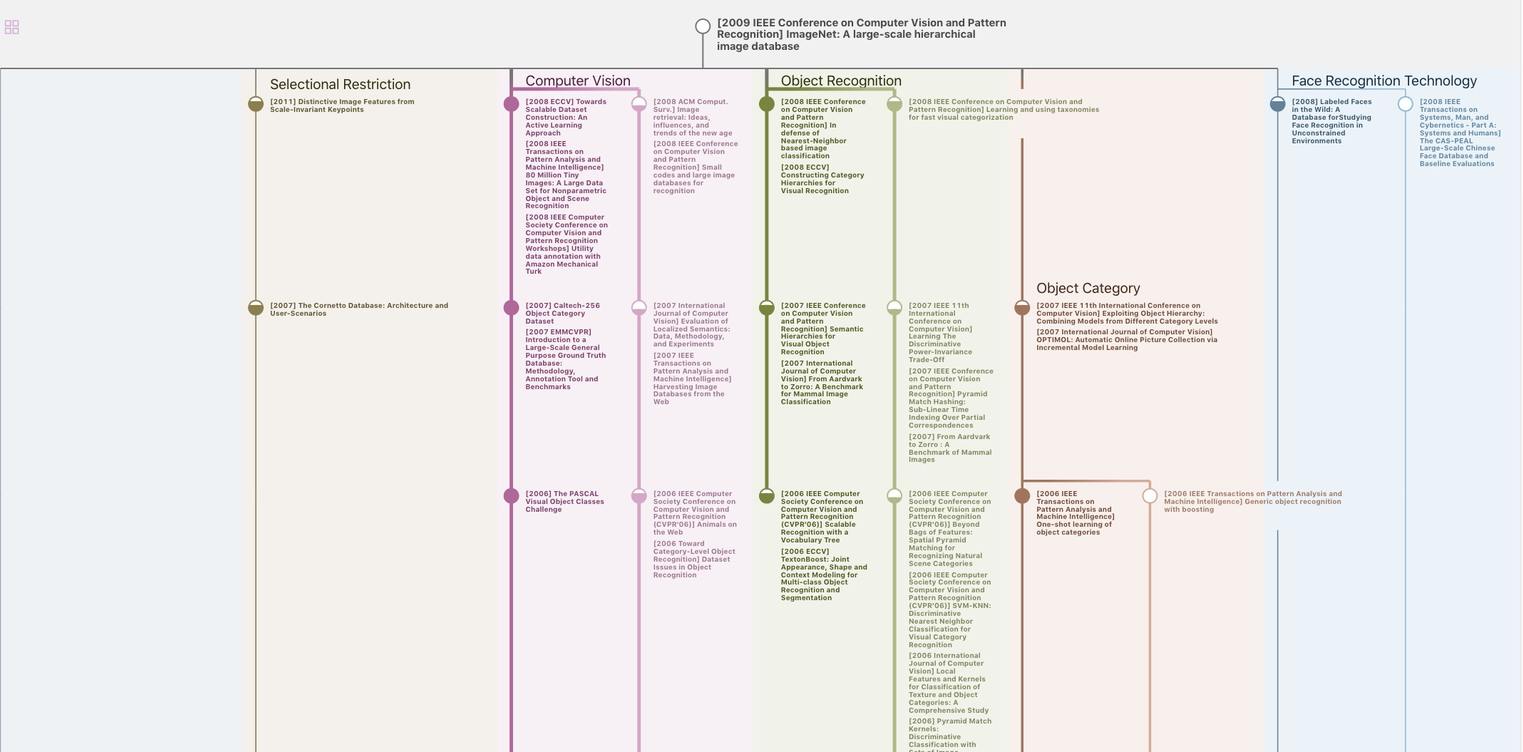
生成溯源树,研究论文发展脉络
Chat Paper
正在生成论文摘要