Molecular Subtype Classification Of Diffuse Large B-Cell Lymphoma By Immunohistochemical Algorithms And Automatic Supervised Classifiers
BLOOD(2016)
摘要
Diffuse large B-cell lymphoma (DLBCL) can be classified into germinal center-like (GCB) and non-germinal center-like (non-GCB) molecular subtype. These entities are driven by different intracellular oncogenic signaling pathways that lead to a distinct clinical outcome (Fang, Xu, u0026 Li, 2010; Lenz et al., 2008). Several immunohistochemical (IHC)-based DLBCL classification algorithms have been proposed, this considers the case when genetic expression profile (GEP) studies are not available. However, there is a major discrepancy within IHC algorithms, and when they are compared to GEP (Coutinho et al., 2013). To address these inconsistencies and determine if an automatic classifier could be used to accurately categorize DLBCL subtype, we perfomed a present a performance comparison between eight reported IHC algorithms (Colomo, Hans, Hans modified [Hans*], Nyman, Choi, Choi modified [Choi*] and Visco-Young with three [VY3] and four [VY4] antibodies) against their counterparts developed by automatic classification techniques, which consider the following structures: Bayesian Classifier (B), Bayesian Simple Classifier (BS), Naive Bayesian Classifier (BN), Artificial Neural Networks (ANN), and Support Vector Machines (SVM). The Visco-Young database (Visco et al., 2012), which contains GEP, IHC raw data corresponding to GCET1, MUM1, FOXP1, BCL6, and CD10 antibodies, and clinical information of 475 de novo DLBCL patients, was used. According to GEP, the database contained 231 GCB, and 244 non-GCB cases. Each patient in VY database was ranked by survival rate as: low survival (0 - 34 months, 237 patients), medium survival (35 - 69 months, 173 patients), or high survival (70 - 106 months, 65 patients) rate. For the implementation of automatic classifiers, the database was split into training, testing and validation data subsets (75%, 20% and 5% respectively) by random selection, but to preserve the same proportion of ranked patients, the so-called k -fold cross-validation technique was applied. The automatic classifier versions of IHC algorithms used the same raw IHC data (antibody combination) as the input, e.g. VY3 used CD10, FOXP1, and BCL6 raw IHC as well as the ANN VY3. A total of 35 automatic classifiers were trained, where Colomo and Hans use the same set of antibodies and are represented by the same automatic classifiers. The stopping criterion during the training stage for all classification algorithms was an error less than 1x10 -3 or 100 training epochs, whichever was satisfied first. The performance of the eight IHC algorithms and the automatic classifiers was evaluated by computing the accuracy (Acc), specificity (Spec), and sensitivity (Sens), according to the Receiver Operating Characteristic procedure. Five classifiers obtained the highest metrics: ANN Choi, BS Choi, and BS Choi* with 94.2% Acc, 93.1% Spec, and 95.2% Sens, followed by SVM Choi and SVM VY4 with 94.2% Acc, 91.4% Spec, and 96.8% Sens. Choi was the IHC algorithm with better metrics (92.5% Acc, 84.5% Spec, and 100% Sens), which ranked 11 out of 43 models tested, followed by VY3 and VY4 (ranked 22 and 23, respectively). Survival of GCB and non-GCB groups identified by these models were compared using Kaplan-Meier curves, and the significance was calculated using log-rank test. For the best five automatic classifiers and the Choi IHC algorithm, GCB overall survival was better than non-GCB cases ( p To statistically compare the models with GEP, all automatic classifiers and IHC algorithms results were analyzed by Cohen9s kappa (κ) for agreement analysis and Pearson9s chi-squared test. Only Choi IHC algorithm had a very good agreement when compared with GEP (κ = 0.85, p p p Harnessing all of the available immunohistochemical data in order to increase the DLBCL classification accuracy when compared with decision three pre-existing algorithms, we conclude that 4 antibody-based BS Choi* automatic classifier provided the best metrics and represents an affordable and time-saving alternative for DLBCL molecular subtype identification. Disclosures No relevant conflicts of interest to declare.
更多查看译文
关键词
molecular subtype classification,lymphoma,immunohistochemical algorithms,b-cell
AI 理解论文
溯源树
样例
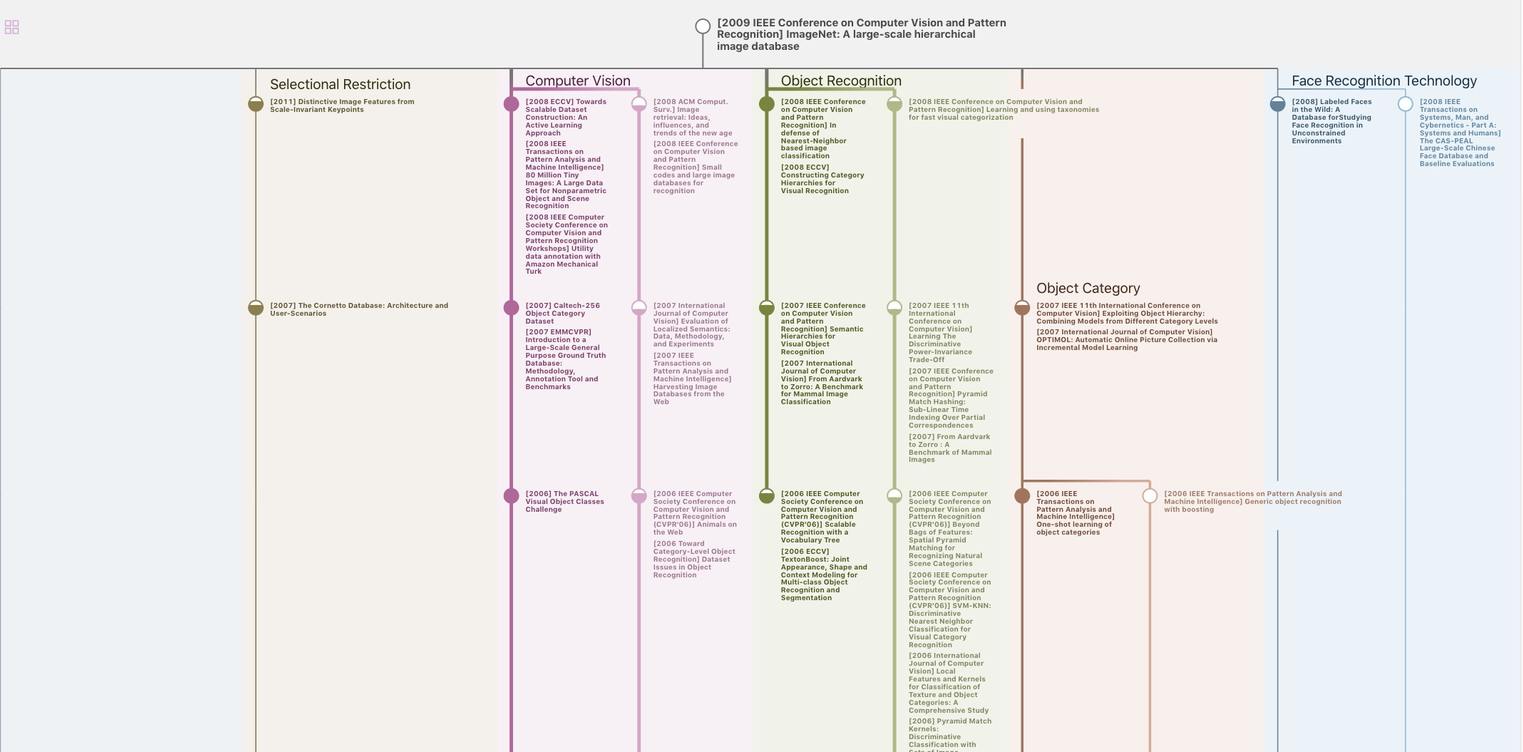
生成溯源树,研究论文发展脉络
Chat Paper
正在生成论文摘要