Memetic Algorithm with Normalized RBF ANN for Approximation of Objective Function and Secondary RBF ANN for Error Mapping
Procedia Engineering(2017)
摘要
Memetic algorithms (MAs) based on genetic algorithm (GAs) often require many evaluations of objective function. In applications like structural optimization a single evaluation of objective function can take from mere seconds to few hours or even days. Using artificial neural networks (ANN) to approximate the objective function can save computational time. To achieve required precision, certain number of training points has to be supplied. The time required to initialize and train the Radial Basis Function Artificial Neural Network (RBF ANN) depends on the number of training points and dimensionality, so it takes longer for more training points and more dimensions. More dimensions require more training points and so it is feasible to use the ANN approximation only for lower number of dimensions.
更多查看译文
关键词
memetic algorithms,radial basis function artificial neural networks,objective function approximation,structural optimization ,
AI 理解论文
溯源树
样例
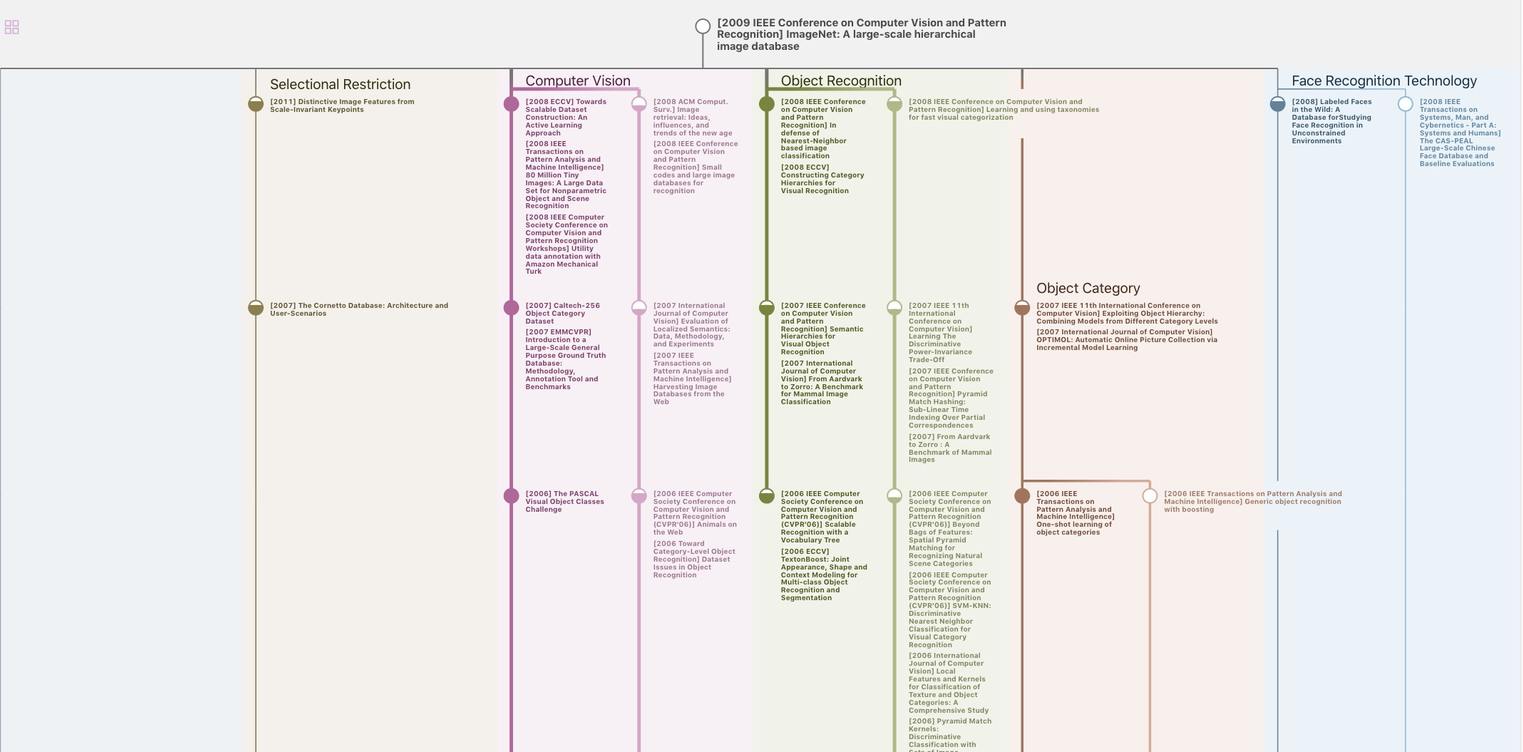
生成溯源树,研究论文发展脉络
Chat Paper
正在生成论文摘要