Geometric foundations for scaling-rotation statistics on symmetric positive definite matrices: Minimal smooth scaling-rotation curves in low dimensions
ELECTRONIC JOURNAL OF STATISTICS(2017)
摘要
We investigate a geometric computational framework, called the "scaling-rotation framework", on Sym(+) (p), the set of p x p symmetric positive-definite (SPD) matrices. The purpose of our study is to lay geometric foundations for statistical analysis of SPD matrices, in situations in which eigenstructure is of fundamental importance, for example diffusion-tensor imaging (DTI). Eigen-decomposition, upon which the scaling-rotation framework is based, determines both a stratification of Sym(+) (p), defined by eigenvalue multiplicities, and fibers of the "eigen-composition" map SO(p) x Diag(+) (p) -> Sym(+) (p). This leads to the notion of scaling-rotation distance [Jung et al. (2015)], a measure of the minimal amount of scaling and rotation needed to transform an SPD matrix, X, into another, Y, by a smooth curve in Sym(+) (p). Our main goal in this paper is the systematic characterization and analysis of minimal smooth scaling-rotation (MSSR) curves, images in Sym(+) (p) of minimal-length geodesics connecting two fibers in the "upstairs" space SO(p) x Diag(+) (p). The length of such a geodesic connecting the fibers over X and Y is what we define to be the scaling-rotation distance from X to Y. For the important low dimensional case p = 3 (the home of DTI), we find new explicit formulas for MSSR curves and for the scaling-rotation distance, and identify the set M(X, Y) of MSSR curves from X to Y in all "nontrivial" cases. The quaternionic representation of SO(3) is used in these computations. We also provide closed-form expressions for scaling-rotation distance and MSSR curves for the case p = 2.
更多查看译文
关键词
Eigen-decomposition,geodesics,stratified spaces,statistics on manifolds,scaling-rotation distance,symmetric group
AI 理解论文
溯源树
样例
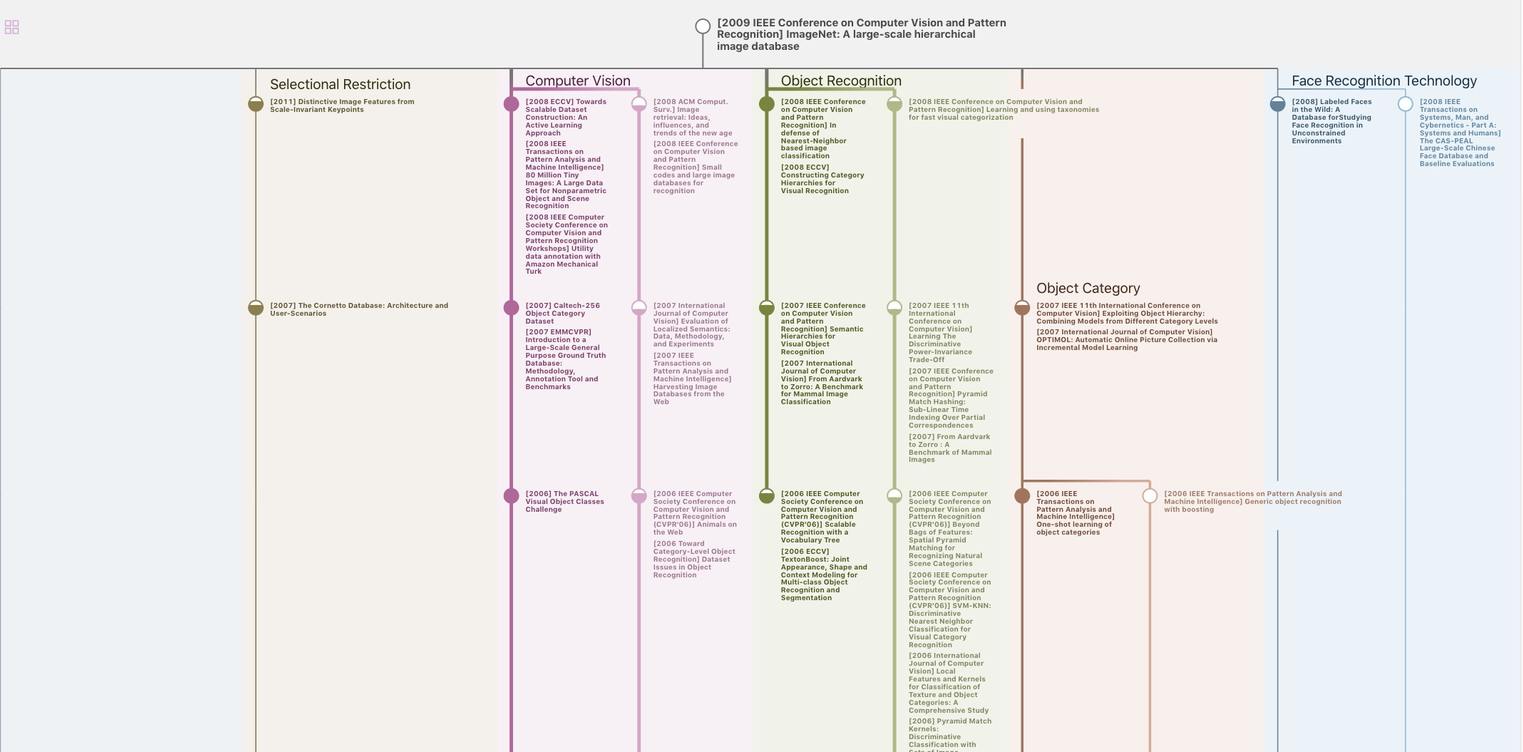
生成溯源树,研究论文发展脉络
Chat Paper
正在生成论文摘要