A Monte Carlo approach to quantifying discrepancies between intractable posterior distributions
JOURNAL OF STATISTICAL COMPUTATION AND SIMULATION(2017)
摘要
The computational demand required to perform inference using Markov chain Monte Carlo methods often obstructs a Bayesian analysis. This may be a result of large datasets, complex dependence structures, or expensive computer models. In these instances, the posterior distribution is replaced by a computationally tractable approximation, and inference is based on this working model. However, the error that is introduced by this practice is not well studied. In this paper, we propose a methodology that allows one to examine the impact on statistical inference by quantifying the discrepancy between the intractable and working posterior distributions. This work provides a structure to analyse model approximations with regard to the reliability of inference and computational efficiency. We illustrate our approach through a spatial analysis of yearly total precipitation anomalies where covariance tapering approximations are used to alleviate the computational demand associated with inverting a large, dense covariance matrix.
更多查看译文
关键词
Model error,Kullback-Leibler,divergence,covariance tapering,approximation
AI 理解论文
溯源树
样例
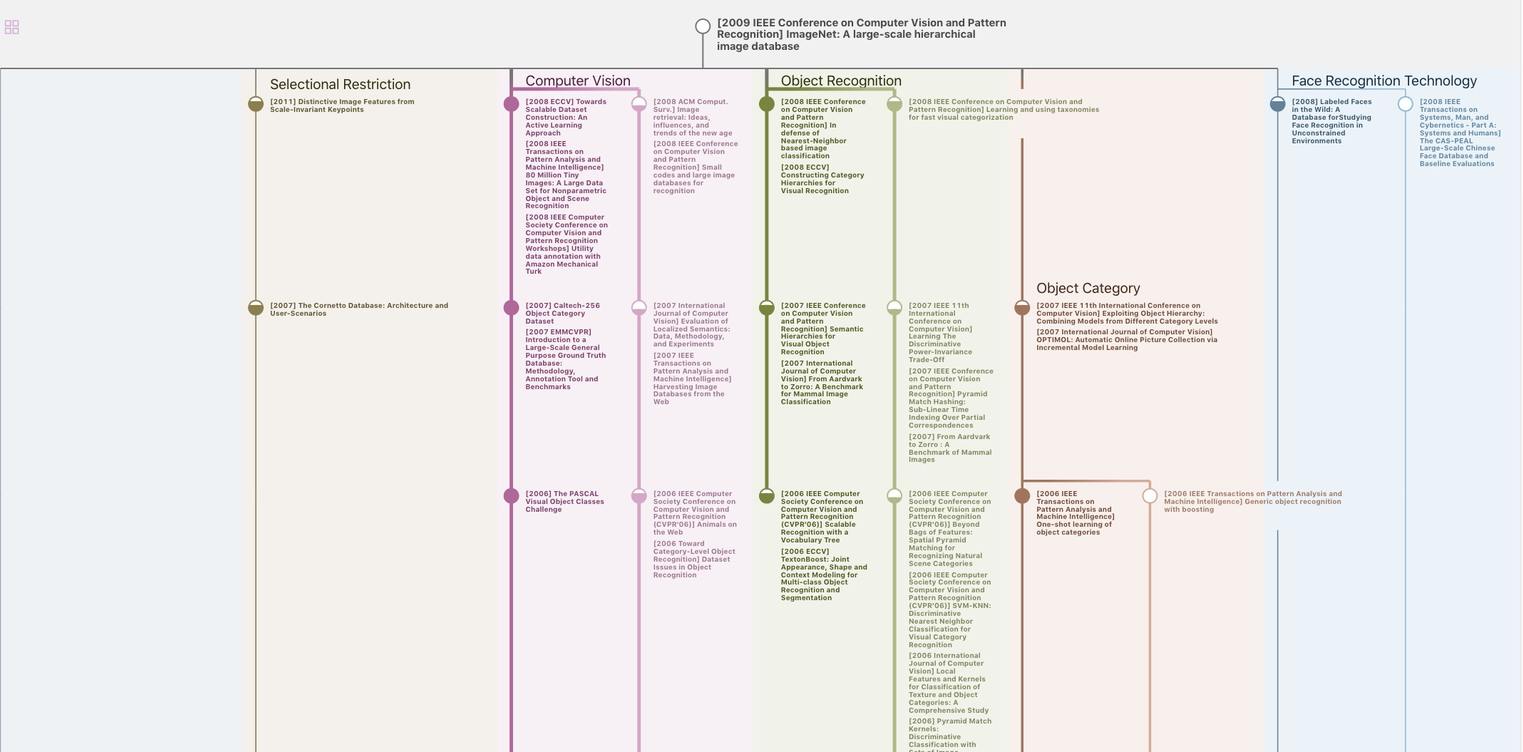
生成溯源树,研究论文发展脉络
Chat Paper
正在生成论文摘要