A Low-Power Multi-Physiological Monitoring Processor For Stress Detection
2016 IEEE SENSORS(2016)
摘要
Personal monitoring systems can offer effective solutions for human health and performance. These systems require sampling and significant processing on multiple streams of physiological signals. The processing typically consists of feature extraction, data fusion, and classification stages that require a large number of digital signal processing and machine learning kernels. In order to be functional, however, the processing architecture needs to be low-power and have a low-area footprint. In this paper we present such a design for a personalized stress monitoring system with a flexible, multi-modal design. Various physiological and behavioral features were explored to maximize detection accuracy with both SVM and KNN machine learning classifiers. Among 17 different features from 5 sensors, heart rate and accelerometer features were found to have the highest classification accuracy to detect stress in the given dataset. While KNN classifier accuracy outperforms by 2%, it requires significantly larger memory and computation compared to the SVM classifier. Therefore, we chose the SVM classifier for hardware implementation. The post-layout implementation results in 130 nm CMOS technology show that the SVM processor occupies 0.2 mm(2) and dissipates 20.2 mW at 125 MHz. The proposed processor takes 800 ns to classify each input and consumes 16.2 nJ. The overall classification accuracy of this system is 96%.
更多查看译文
关键词
Multi-modal Monitoring,Machine Learning Classifiers,Stress Monitoring,Wearable,ASIC
AI 理解论文
溯源树
样例
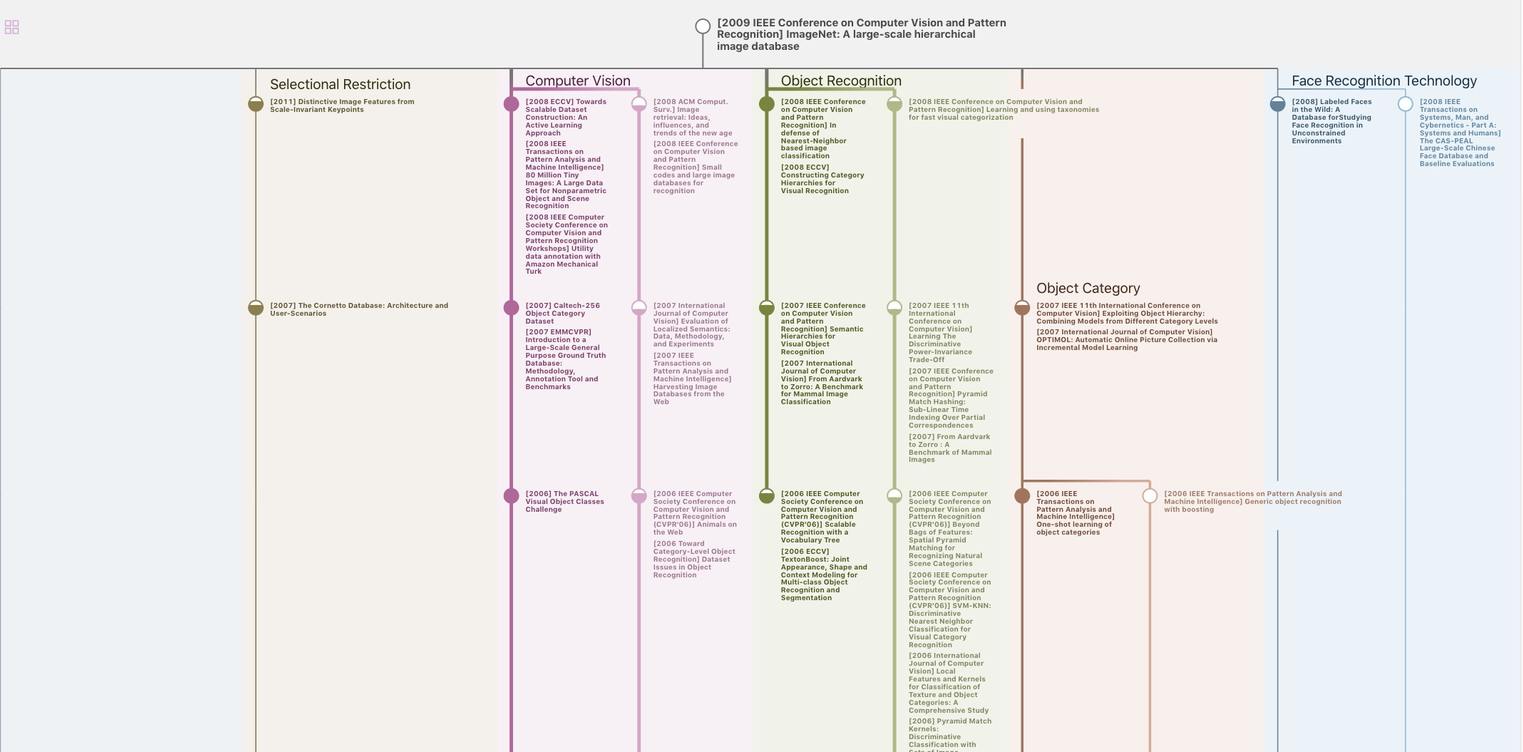
生成溯源树,研究论文发展脉络
Chat Paper
正在生成论文摘要