A new fault detection index based on Mahalanobis distance and kernel method
The International Journal of Advanced Manufacturing Technology(2017)
摘要
This paper suggests an extension of the PCMD index proposed for sensor fault diagnosis in linear systems to the nonlinear case. The proposed index is entitled KPCMD, and it is based on Mahalanobis distance and moving window kernel principal component analysis (MWKPCA) technique. The principle of this index is to detect dissimilarity between a reference KPCA model that represents normal operation of the system and a current KPCA model that represents current system behavior. The proposed KPCMD index compute Mahalanobis distance between principal components corresponding to the reference KPCA model and those corresponding to the current KPCA model which are obtained online using MWKPCA. The proposed KPCMD indices have been applied successfully for monitoring of numerical example as well a continuous stirred tank reactor (CSTR).
更多查看译文
关键词
Kernel principal component analysis (KPCA),KPCMD,SPE,Fault detection
AI 理解论文
溯源树
样例
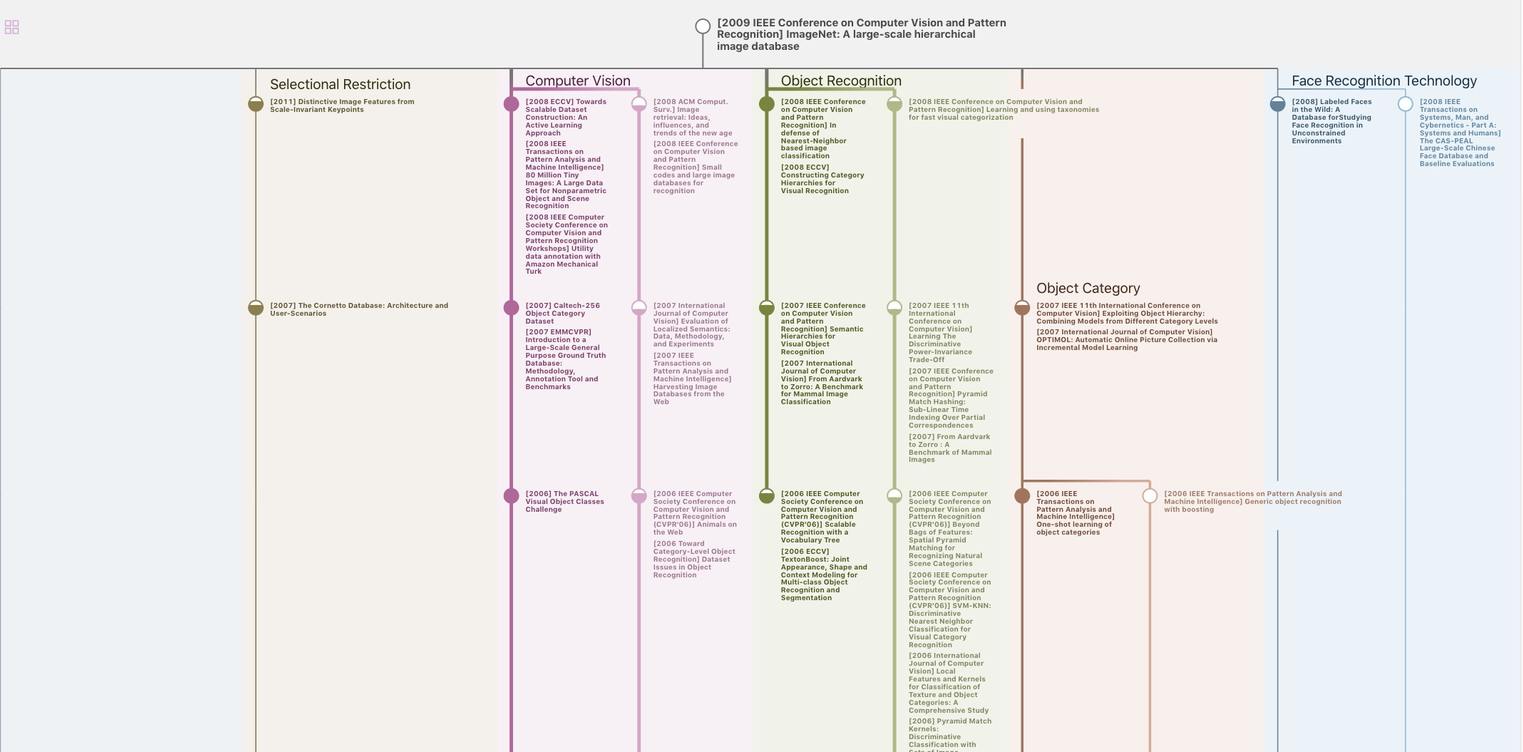
生成溯源树,研究论文发展脉络
Chat Paper
正在生成论文摘要