Mixture of Clustered Bayesian Neural Networks for Modeling Friction Processes at the Nanoscale
JOURNAL OF CHEMICAL THEORY AND COMPUTATION(2017)
摘要
Friction and wear are the source of every mechanical device failure, and lubricants are essential for the operation of the devices. These physical phenomena have a complex nature so that no model capable of accurately predicting the behavior of lubricants exists. Thus, lubricants cannot be designed from scratch but have to be screened through expensive trial-error tests. In this study we propose a machine learning (ML) method that infers the relationship between chemical composition of lubricants and their performance from a database. Because no such database of desirable size and completeness is publicly available, we compiled one from molecular dynamics (MD) simulations of toy-model fluids nanoconfined between shearing surfaces. The fluid-friction relation is modeled by a Bayesian neural network (BNN), trained to reproduce the results for a training set of fluids. Due to the inhomogeneous data distribution it was necessary to carefully pick fluids for training and validation from the database with advanced clustering algorithms, rather than using the standard random selection. Different BNNs were then trained on the data clusters and their predictions combined into a mixture of experts. The model provides a prediction of lubricants performance as well as an error bar, at a fraction of the cost of MD. Because most values agree with the actual MD simulations within the estimated error sigma, we conclude that the model is satisfactory. This method addresses the challenges brought by noisy, badly distributed, high-dimensional data that are likely to appear in reality as well, and it can be extended to real fluids, if a database could be provided.
更多查看译文
AI 理解论文
溯源树
样例
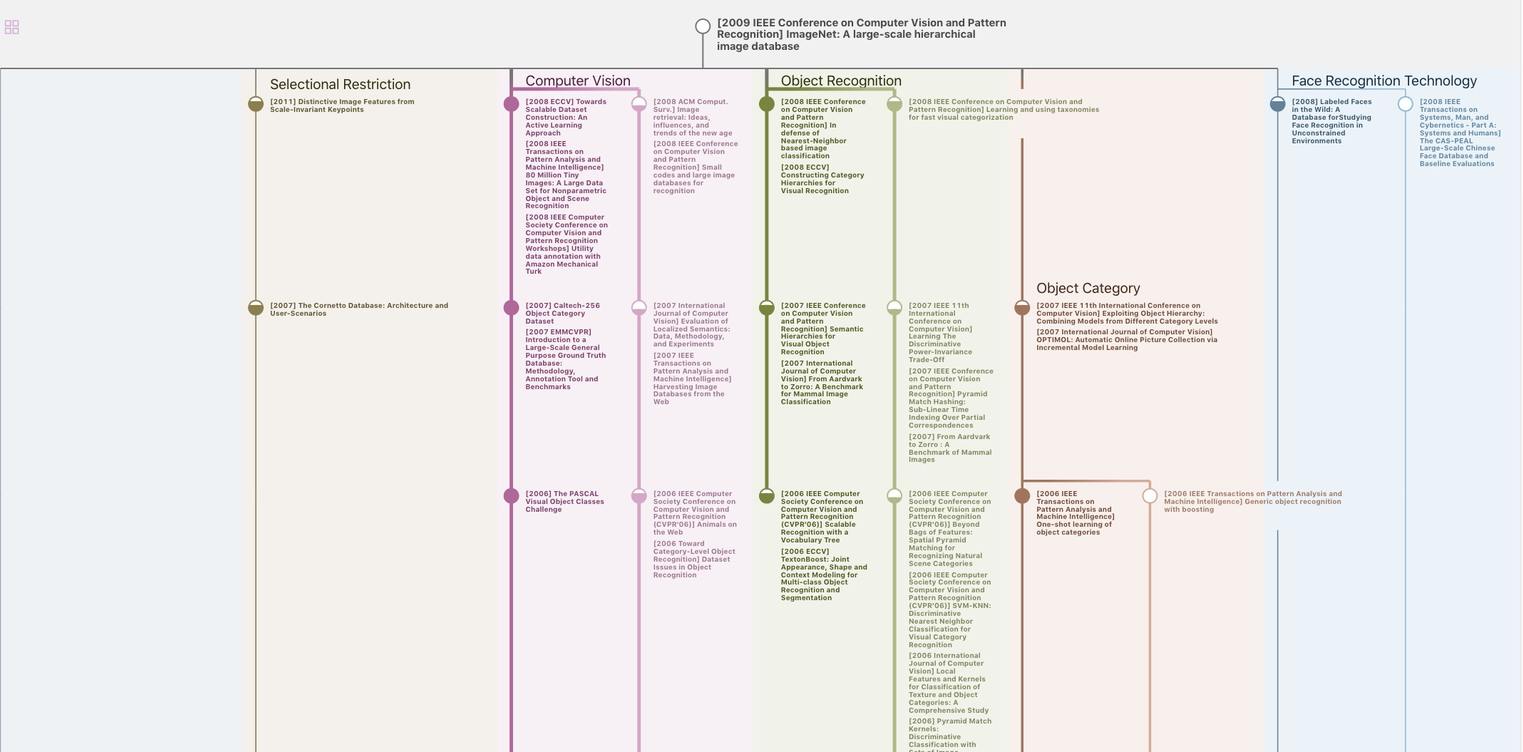
生成溯源树,研究论文发展脉络
Chat Paper
正在生成论文摘要