Benchmarking Factor Selection And Sensitivity: A Case Study With Nursing Courses
STUDIES IN HIGHER EDUCATION(2018)
摘要
There is an increasing requirement in higher education (HE) worldwide to deliver excellence. Benchmarking is widely used for this purpose, but methodological approaches to the creation of benchmark metrics vary greatly. Approaches require selection of factors for inclusion and subsequent calculation of benchmarks for comparison. We describe an approach using machine learning to select input factors based on their value to predict completion rates of nursing courses. Data from over 36,000 students, from nine institutions over three years were included and weighted averages provided a dynamic baseline for year on year and within year comparisons between institutions. Anonymised outcomes highlight the variation in benchmarked performances between institutions and we demonstrate the value of accompanying sensitivity analyses. Our methods are appropriate worldwide, for many forms of data and at multiple scales of enquiry. We discuss our results in the context of HE management, highlighting the value of scrutinising benchmark calculations.
更多查看译文
关键词
Metric benchmarks, machine learning, RandomForest, factor selection, sensitivity
AI 理解论文
溯源树
样例
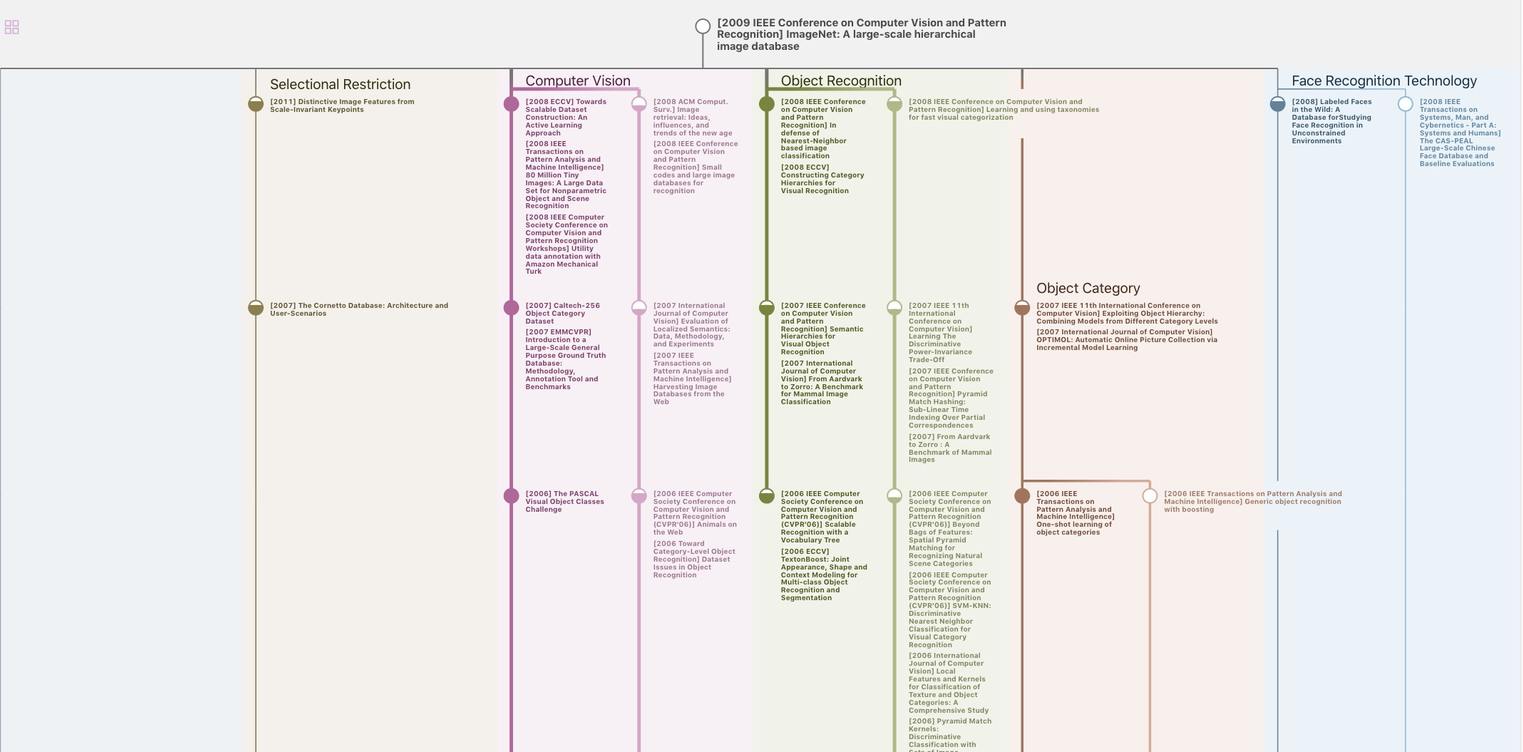
生成溯源树,研究论文发展脉络
Chat Paper
正在生成论文摘要