co-BPM: a Bayesian Model for Divergence Estimation
arXiv: Computation(2014)
摘要
Divergence is not only an important mathematical concept in information theory, but also applied to machine learning problems such as low-dimensional embedding, manifold learning, clustering, classification, and anomaly detection. We proposed a bayesian model---co-BPM---to characterize the discrepancy of two sample sets, i.e., to estimate the divergence of their underlying distributions. In order to avoid the pitfalls of plug-in methods that estimate each density independently, our bayesian model attempts to learn a coupled binary partition of the sample space that best captures the landscapes of both distributions, then make direct inference on their divergences. The prior is constructed by leveraging the sequential buildup of the coupled binary partitions and the posterior is sampled via our specialized MCMC. Our model provides a unified way to estimate various types of divergences and enjoys convincing accuracy. We demonstrate its effectiveness through simulations, comparisons with the \emph{state-of-the-art} and a real data example.
更多查看译文
关键词
Statistics,Sample space,Nonlinear dimensionality reduction,Mathematics,Markov chain Monte Carlo,Information theory,Inference,Cluster analysis,Bayesian probability,Bayesian inference,Algorithm
AI 理解论文
溯源树
样例
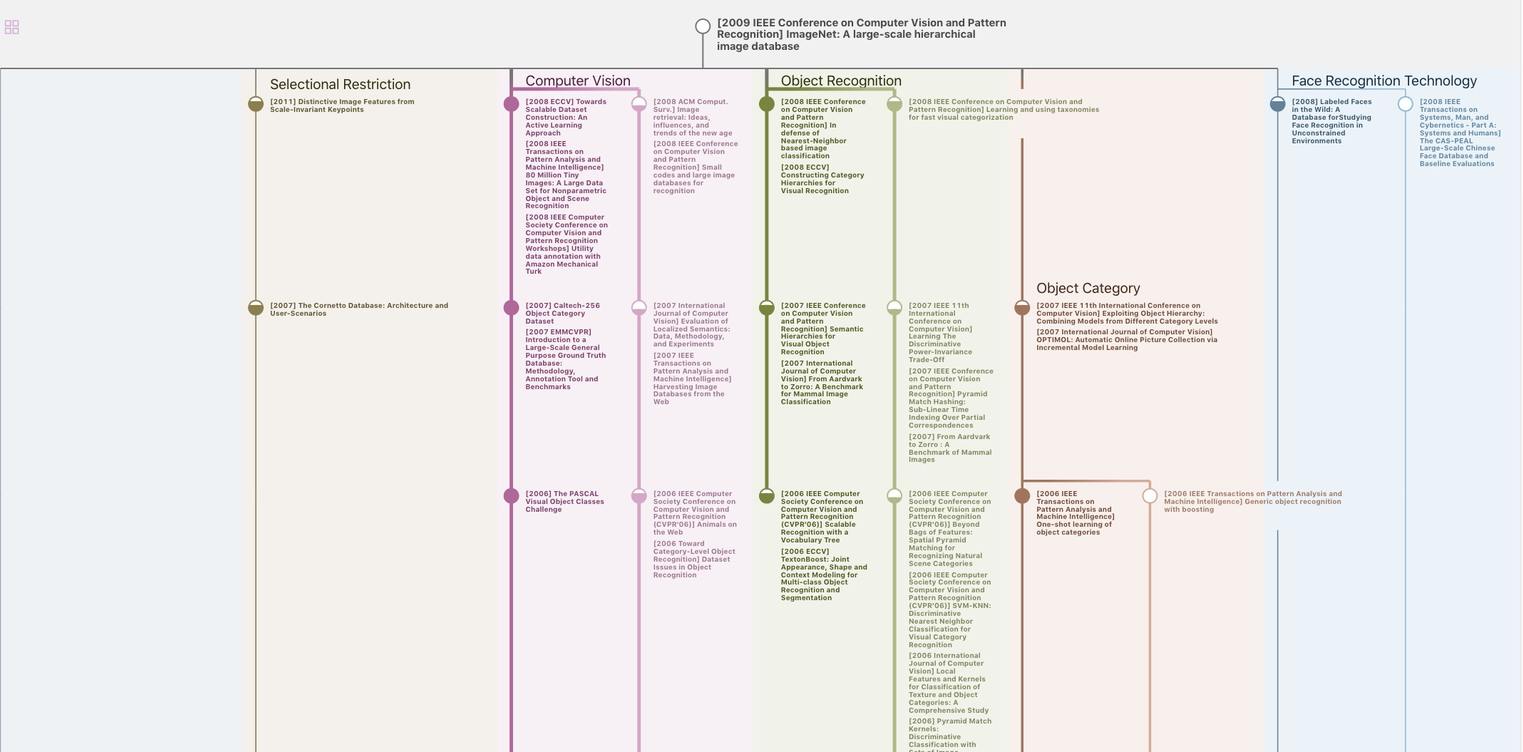
生成溯源树,研究论文发展脉络
Chat Paper
正在生成论文摘要