METAPHOR: a machine-learning-based method for the probability density estimation of photometric redshifts
MONTHLY NOTICES OF THE ROYAL ASTRONOMICAL SOCIETY(2017)
摘要
A variety of fundamental astrophysical science topics require the determination of very accurate photometric redshifts (photo-z). A wide plethora of methods have been developed, based either on template models fitting or on empirical explorations of the photometric parameter space. Machine-learning-based techniques are not explicitly dependent on the physical priors and able to produce accurate photo-z estimations within the photometric ranges derived from the spectroscopic training set. These estimates, however, are not easy to characterize in terms of a photo-z probability density function (PDF), due to the fact that the analytical relation mapping the photometric parameters on to the redshift space is virtually unknown. We present METAPHOR (Machine-learning Estimation Tool for Accurate PHOtometric Redshifts), a method designed to provide a reliable PDF of the error distribution for empirical techniques. The method is implemented as a modular workflow, whose internal engine for photo-z estimation makes use of the MLPQNA neural network (Multi Layer Perceptron with Quasi Newton learning rule), with the possibility to easily replace the specific machine-learning model chosen to predict photo-z. We present a summary of results on SDSS-DR9 galaxy data, used also to perform a direct comparison with PDFs obtained by the L-E P-HARE spectral energy distribution template fitting. We show that METAPHOR is capable to estimate the precision and reliability of photometric redshifts obtained with three different self-adaptive techniques, i.e. MLPQNA, Random Forest and the standard K-Nearest Neighbors models.
更多查看译文
关键词
techniques: photometric,galaxies: distances and redshifts,galaxies: photometry
AI 理解论文
溯源树
样例
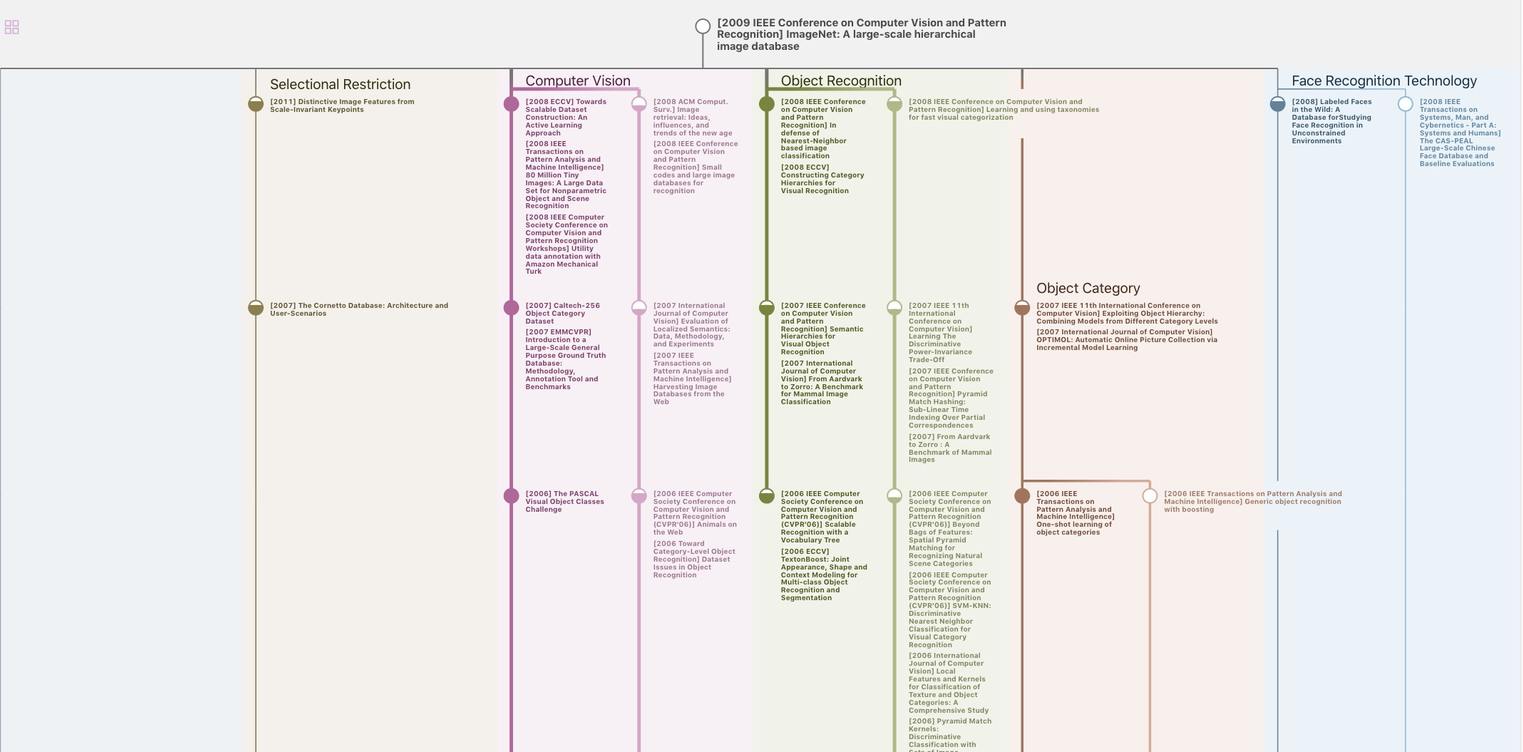
生成溯源树,研究论文发展脉络
Chat Paper
正在生成论文摘要