Automatic Classification Of Toco-Signals: An Approach Towards Comprehensive Monitoring Of Labour Progress
2016 INTERNATIONAL CONFERENCE ON SIGNAL PROCESSING AND COMMUNICATIONS (SPCOM)(2016)
摘要
Childbirth is an intricate process which is marked by an increased cervical dilation rate caused due to steady increments in the frequency and strength of uterine contractions. The contractions may be characterized by its strength, duration and frequency (count) - which are monitored through Tocography. However, the procedure is prone to subjectivity and an automated approach for the classification of the contractions is needed. In this paper, we use three different Weighted K-Nearest Neighbor classifiers and Decision Trees to classify the contractions into three types: Mild, Moderate and Strong. Further, we note the fact that our training data consists of fewer samples of Contractions as compared to those of Non-contractions - resulting in "Class Imbalance". Hence, we use the Synthetic Minority Oversampling Technique (SMOTE) in conjunction with the K-NN classifier and Decision Trees to alleviate the problems of the same.The ground truth for Tocography signals was established by a doctor having an experience of 36 years in Obstetrics and Gynaecology. The annotations are in three categories: Mild (33 samples), Moderate (64 samples) and Strong (96 samples), amounting to a total of 193 contractions whereas the number of Non-contraction samples was 1217. Decision Trees using SMOTE performed the best with accuracies of 95%, 98.25% and 100% for the aforementioned categories, respectively. The sensitivities achieved for the same are 96.67%, 96.52% and 100% whereas the specificities amount to 93.33%, 100% and 100%, respectively. Our method may be used to monitor the labour progress efficiently.
更多查看译文
关键词
Uterine contractions,Subjectivity,K-Nearest Neighbour,Decision Trees,SMOTE
AI 理解论文
溯源树
样例
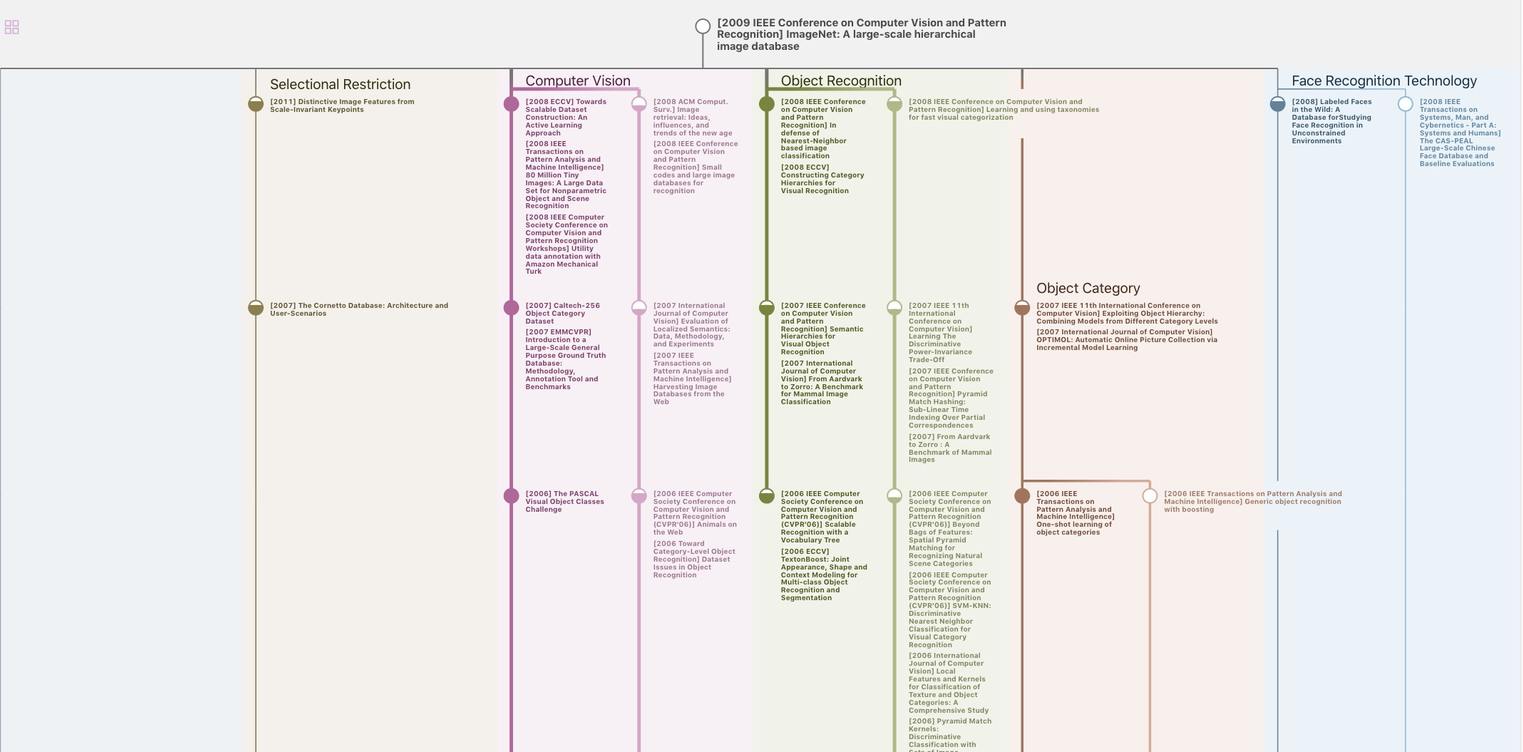
生成溯源树,研究论文发展脉络
Chat Paper
正在生成论文摘要