Accelerated Learning Of Discriminative Spatio-Temporal Features For Action Recognition
2016 INTERNATIONAL CONFERENCE ON SIGNAL PROCESSING AND COMMUNICATIONS (SPCOM)(2016)
摘要
Recently, paradigm has shifted from hand-designed local feature learning to unsupervised learning in order to extract features from raw data. In action recognition, good results are achieved using deep learning techniques such as stacking and convolution to extend the idea of independent subspace analysis (ISA). Albeit performance is good, it takes significant amount of time on big datasets due to high computational complexity and sequential implementation. We propose two methods for speeding up feature learning using ISA. We also propose input data modification which increases the classification performance. One method of faster feature learning is parallelization - we use the scalable programming model, MapReduce to parametrize ISA algorithm by distributing datasets into equal disjoint sets. The second method for increasing speed is by using spatio-temporal interest point detectors to extract "important" blocks from video. The latter not only enhances the speed but also improves the classification accuracy. We modified input as the gradient of video and achieved a better classification accuracy on all the datasets that were tested. We also created a dataset of water activities and used the ISA network for feature extraction. We achieved speed up by a factor of 4 and 2.4 in first and second method respectively.
更多查看译文
关键词
accelerated learning,discriminative spatio-temporal features,action recognition,unsupervised learning,deep learning techniques,independent subspace analysis,computational complexity,sequential implementation,feature learning,scalable programming model,spatio-temporal interest point detectors,feature extraction
AI 理解论文
溯源树
样例
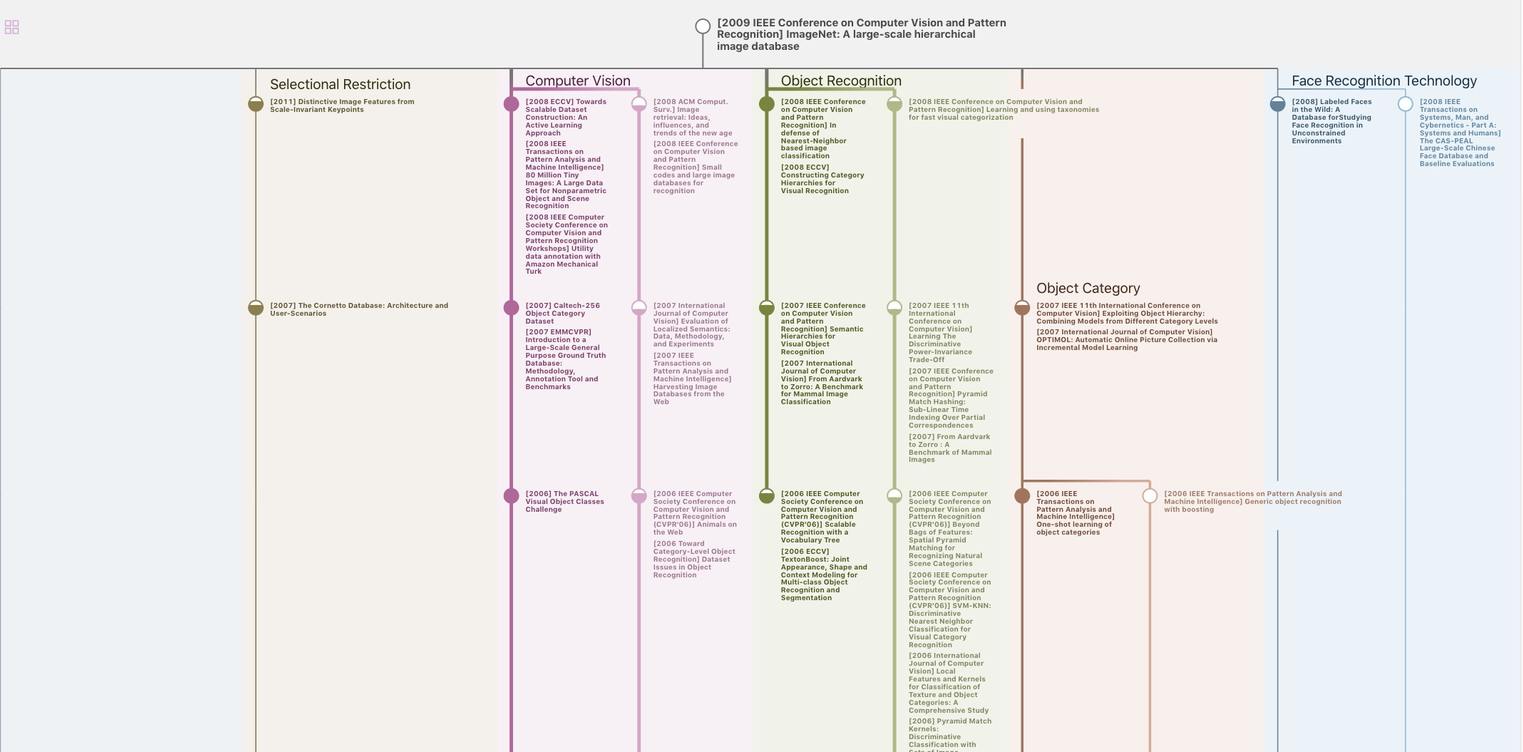
生成溯源树,研究论文发展脉络
Chat Paper
正在生成论文摘要