Sparse interpretable estimators for cyclic arrival rates
arXiv: Machine Learning(2016)
摘要
Exploiting the fact that most arrival processes exhibit cyclic (though not necessarily periodic) behaviour, we propose a simple and computationally efficient procedure for estimating the intensity of a nonhomogeneous Poisson process. The estimator is interpretable because it is a simple sum of p sinusoids, although p and the frequency, amplitude, and phase of each wave are not known and need to be estimated. This results in a flexible specification that is suitable for use in modeling as well as in high resolution simulations. Our estimation procedure sits in between classic periodogram methods and atomic/total variation norm thresholding. A novel aspect of our approach is the use of window functions with fast decaying spectral tails, which we show improves frequency resolution as well as prevent strong frequency signals from masking weaker ones nearby. Interestingly the prolate spheriodal window that is usually considered optimal in signal processing is suboptimal for frequency recovery. Under suitable conditions, finite sample performance guarantees for our procedure can be derived that resolve open questions and expand existing results in spectral estimation literature. Preliminary simulations suggest that our procedure can potentially be extended to Cox processes which are used to model overdispersion, although a formal analysis is left for future research. We apply our procedure to arrivals data from an emergency department at an academic hospital in the United States to examine differences in arrival patterns by illness severity.
更多查看译文
AI 理解论文
溯源树
样例
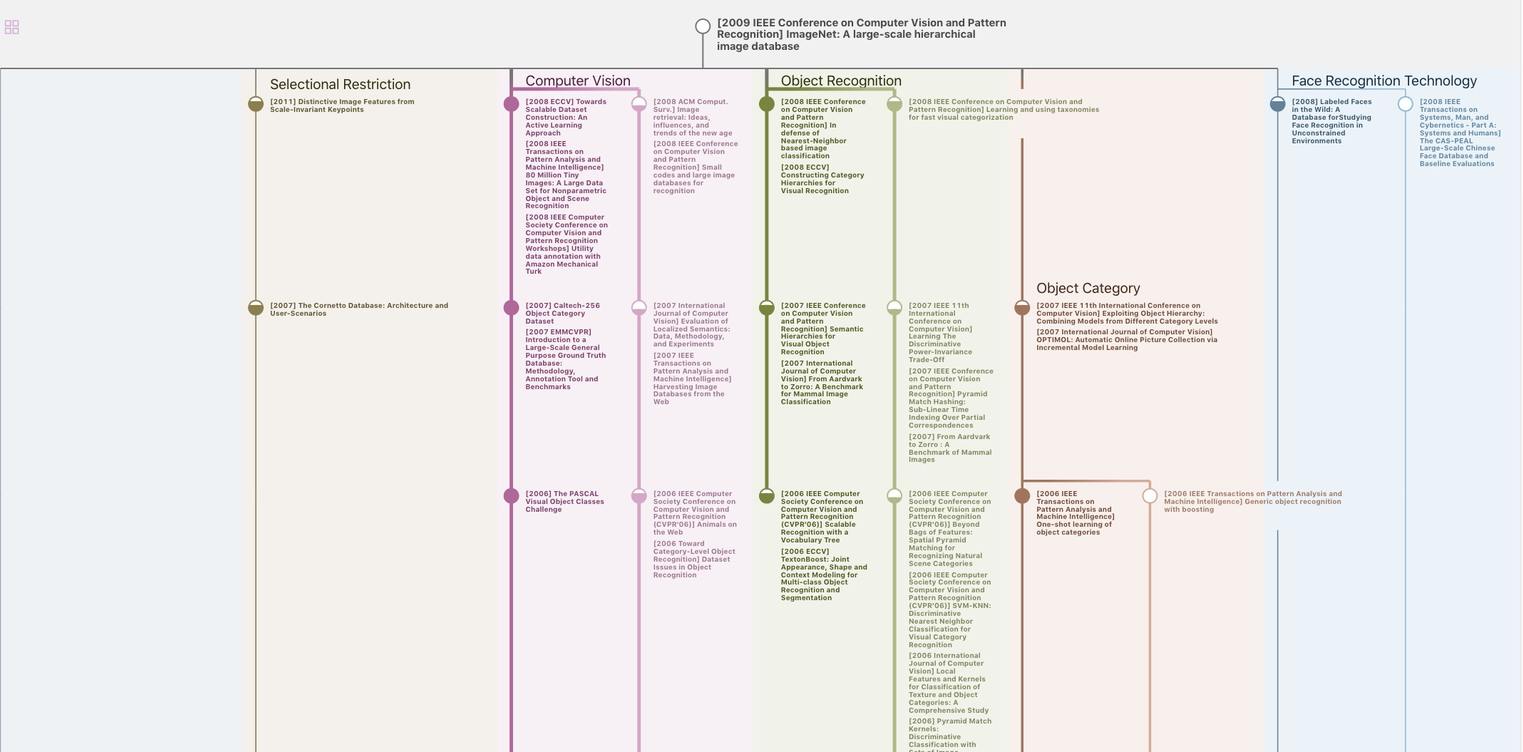
生成溯源树,研究论文发展脉络
Chat Paper
正在生成论文摘要