Abstract A018: High-value T cell epitope selection for mutanome-directed cancer immunotherapy using an innovative cancer neo-epitope classification system
CANCER IMMUNOLOGY RESEARCH(2016)
摘要
T cell epitopes bearing tumor-specific mutations discovered using whole-exomic and transcriptomic sequencing of tumor-normal pairs stimulate T cell-mediated processes that lead to tumor regression. Although neo-epitope prediction using computational methods rapidly identifies epitope candidates in the mutanome, a large proportion of neo-epitopes prove to be non-immunogenic. Innovative computational tools validated for infectious disease targets can be applied to enhance design of personalized cancer immunotherapies by classification of predicted epitopes by potential for mounting a tumor-specific response. Tumor-specific epitopes with potential to stimulate naive T cells and epitopes that may cross-react with antigen-experienced T cells raised in infection are valuable targets. Epitopes with potential to activate regulatory T cells trained on self and commensal antigens are counter-indicated. We developed the JanusMatrix algorithm that parses query sequences into MHC-facing and T cell receptor (TCR)-facing sequences and screens sequence databases to identify MHC ligands that share TCR faces with host-related proteins. A database of human protein sequences is available to identify tumor-specific epitopes that may reduce anti-tumor activity by sequences that activate regulatory T cells (Tregs) trained in the thymus on self-antigens. Similarly, tumor-specific epitope candidates are screened using databases composed of antigens derived from human commensals or pathogens to identify epitopes that, respectively, may detrimentally or beneficially cross-react with T cells raised over the course of an individual9s immune history. Neo-epitope candidates are ranked according to MHC binding potential, TCR face homology. We conducted retrospective analyses of cancer vaccine efficacy studies performed in mice [Kreiter et al . 2015 Nature 520, 692–696] showing that mutanome-directed vaccines effective at preventing tumor growth contained higher numbers of class I and II MHC neo-epitopes, as identified by EpiVax9s iVAX platform, and had lower potential to cross-react with other murine proteins. An evaluation of mutanomes derived from non-small cell lung cancer patients [Rizvi et al. 2014 Science 348, 124-128] confirmed these findings. Improved clinical outcomes were observed in patients with mutanomes enriched in class I MHC neo-epitopes with TCR faces distinct from other human-derived epitopes. These results highlight the benefits of using in silico prediction tools for the selection of neo-epitopes and how they can improve the design and efficacy of future cancer vaccines. While retrospective in nature, the suite of tools used for these analyses have been extensively validated in prospective vaccine studies for infectious disease. Removal of Treg epitopes, identified by JanusMatrix, has led to the development of more immunogenic vaccine antigens. EpiVax9s H7N9 influenza Treg epitope-depleted vaccine is scheduled for Phase I clinical trial. Direct application of JanusMatrix to the neo-epitope-driven cancer vaccine discovery and design pipeline will focus candidate selection on higher-value sequences than what conventional T cell epitope mapping algorithms generate. Neo-epitopes with low Treg activation potential may then be used to support development of personalized therapies including vaccination and in vitro expansion of tumor infiltrating lymphocytes for adoptive cell transfer. Citation Format: Lenny Moise, Guilhem Richard, Frances Terry, William Martin, Anne De Groot. High-value T cell epitope selection for mutanome-directed cancer immunotherapy using an innovative cancer neo-epitope classification system [abstract]. In: Proceedings of the Second CRI-CIMT-EATI-AACR International Cancer Immunotherapy Conference: Translating Science into Survival; 2016 Sept 25-28; New York, NY. Philadelphia (PA): AACR; Cancer Immunol Res 2016;4(11 Suppl):Abstract nr A018.
更多查看译文
AI 理解论文
溯源树
样例
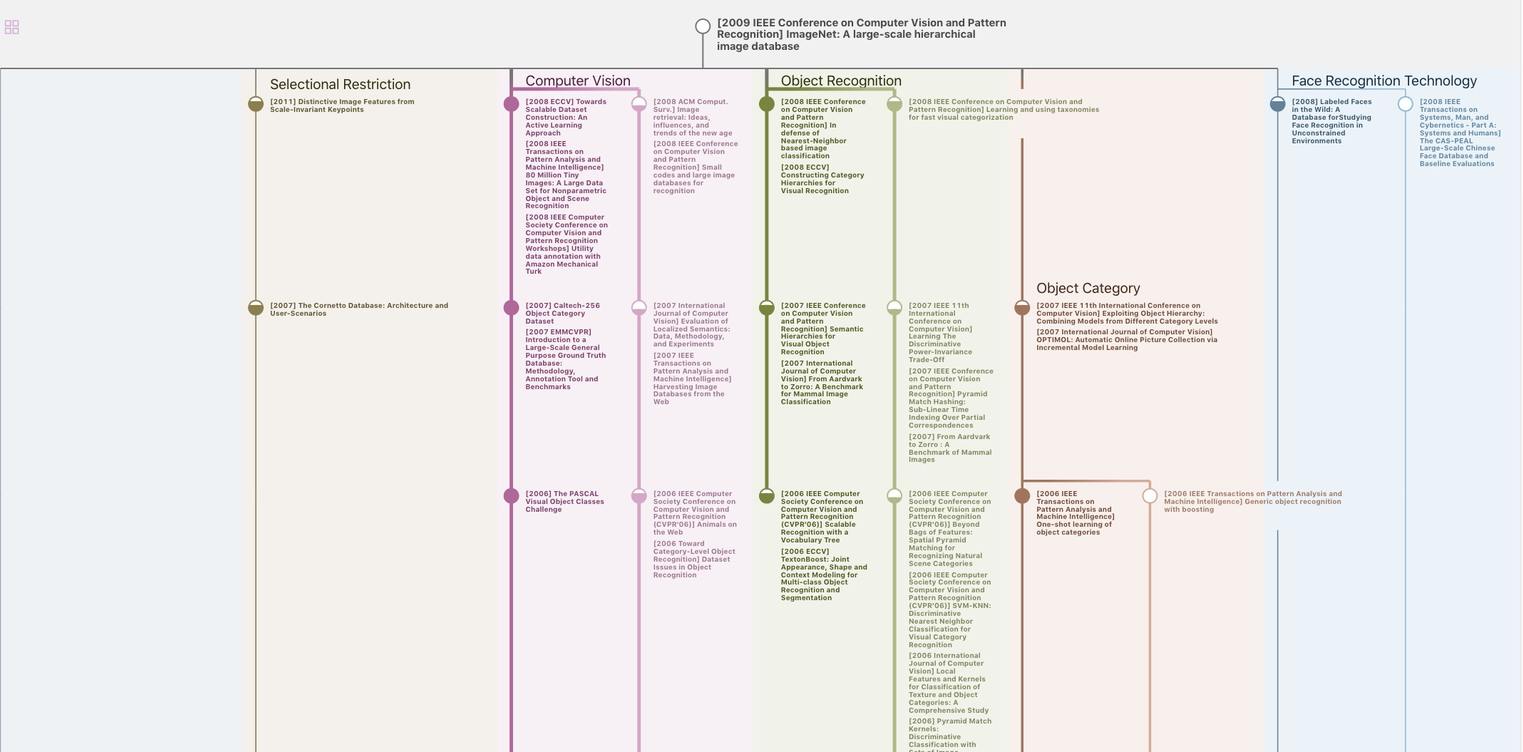
生成溯源树,研究论文发展脉络
Chat Paper
正在生成论文摘要