Hierarchically correlated equilibrium Q-learning for multi-area decentralized collaborative reactive power optimization
CSEE Journal of Power and Energy Systems(2016)
摘要
A hierarchically correlated equilibrium Q-learning (HCEQ) algorithm for reactive power optimization that considers carbon emission on the grid-side as an optimization objective, is proposed here. Based on the multi-area decentralized collaborative framework, the controllable variables in each region are divided into several optimization layers, which is an effective method for solving the limitations posed by dimensionality. The HCEQ provides constant information on the interaction between the state-action value function matrices, as well as on the cooperative game equilibrium among agents in each region. After acquiring the optimal value function matrix in the pre-learning process, HCEQ is able to quickly achieve an optimal solution online. Simulation of the IEEE 57-bus system is performed, which demonstrates that the proposed algorithm can effectively solve multi-area decentralized collaborative reactive power optimization, with the desired global search capabilities and convergence speed.
更多查看译文
关键词
Reactive power,Optimization,Power grids,Generators,Carbon dioxide,Collaboration,Linear programming
AI 理解论文
溯源树
样例
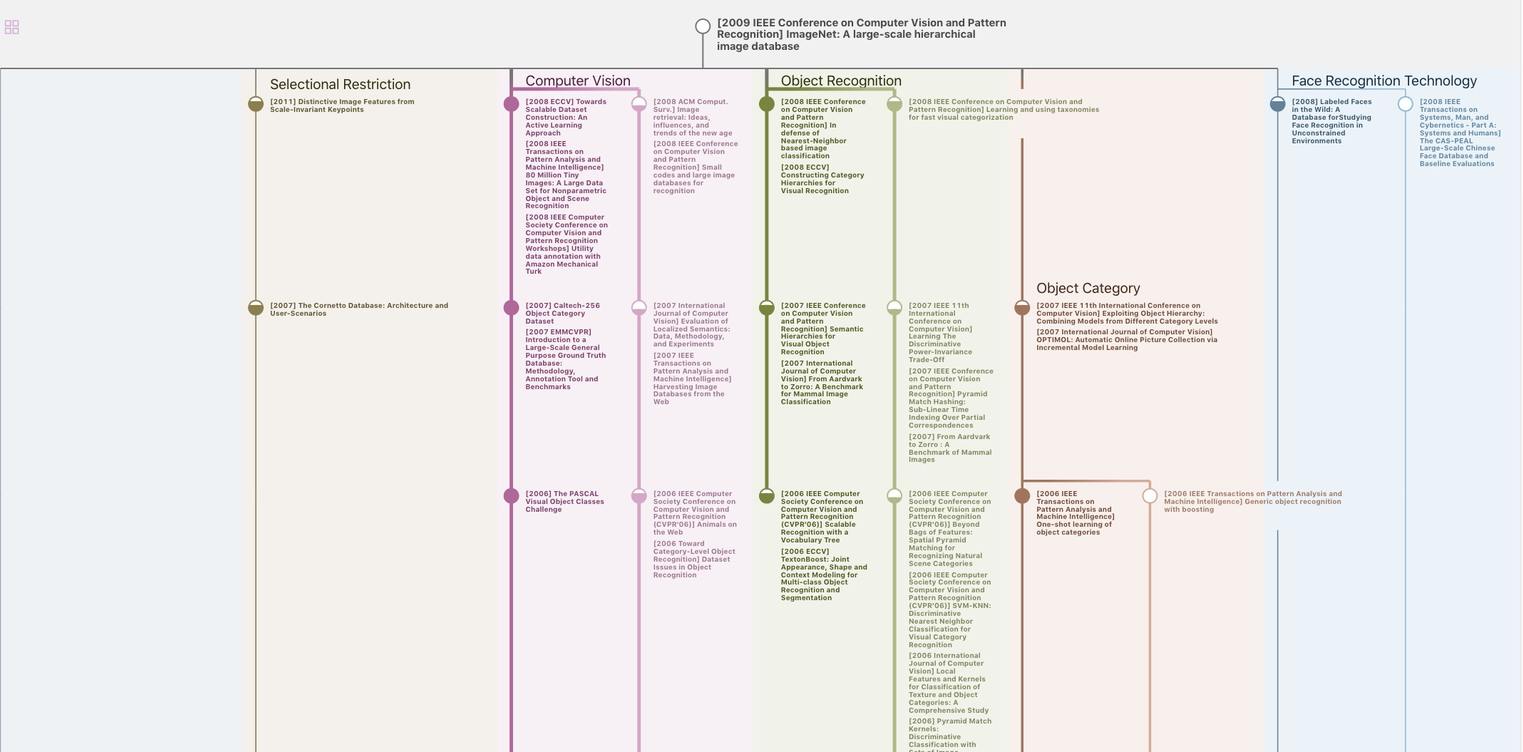
生成溯源树,研究论文发展脉络
Chat Paper
正在生成论文摘要