A Model Based Framework For Land Cover Classification In High Resolution Satellite Images
27TH SESSION OF THE CIE, VOL. 1, PTS 1 AND 2(2011)
摘要
This paper presents a parametric framework for the solution of land cover classification problem in high resolution IKONOS and QuickBird satellite images. In the first step, we merge the multispectral images of low spatial resolution with a monospectral image of higher spatial resolution in order to improve the resolution of multispectral images. This image fusion is based on the search of an optimal 3D hybrid colour space. The second step consists of the parametric segmentation of these high resolution images. This segmentation is based on the 2D multichannel QPAR linear prediction model. A multivariate Gaussian mixture model approximation is used to model the probability distribution of the multichannel linear prediction errors for an initial class label field estimation. These coarsely classified results are then improved by using a spatial regularization algorithm. Experimental results show the pertinence and the robustness of the approach. A comparison of the land cover classification results in different colour spaces is also presented.
更多查看译文
关键词
Land cover classification, Satellite image fusion, Satellite image segmentation, MGMM, Colour space comparison
AI 理解论文
溯源树
样例
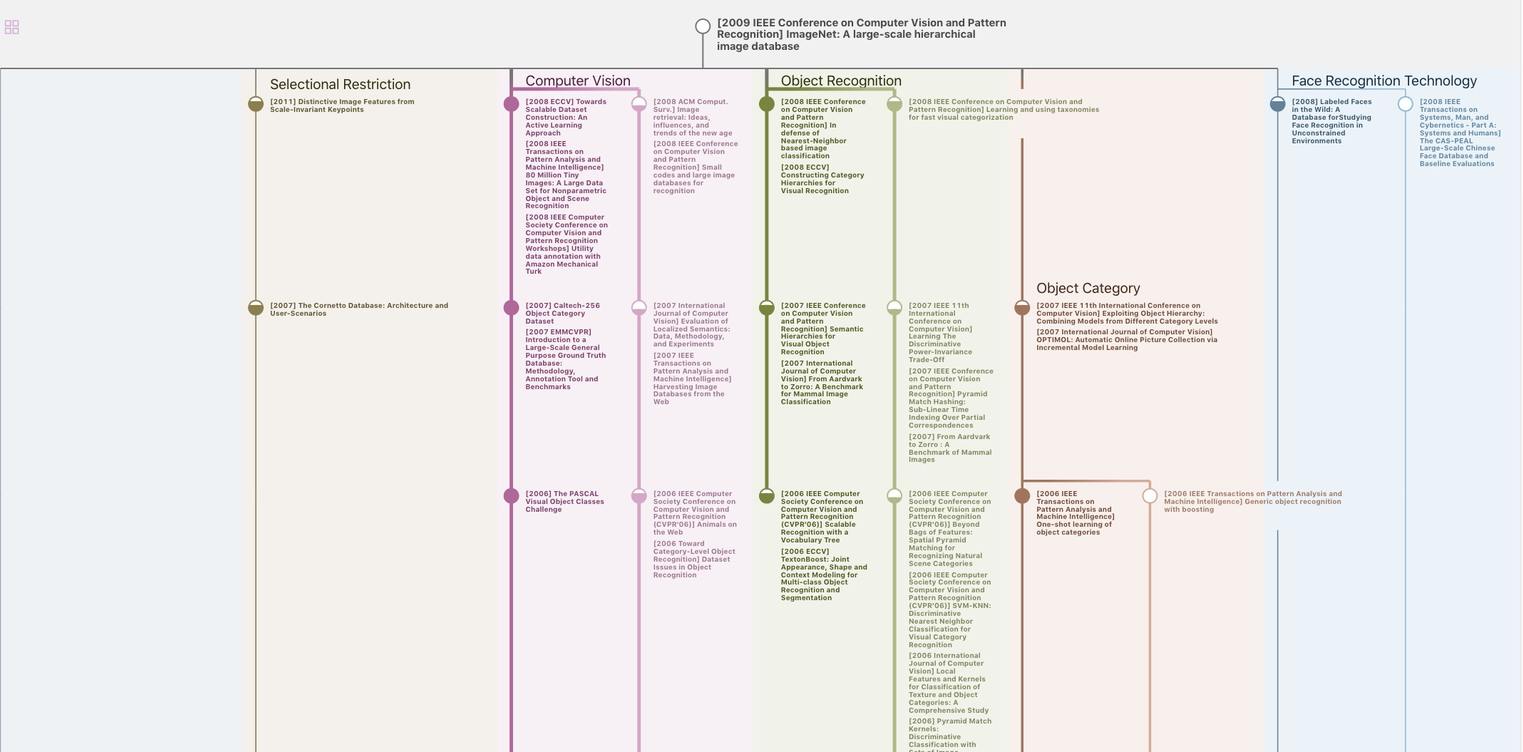
生成溯源树,研究论文发展脉络
Chat Paper
正在生成论文摘要