Novel RRAM-enabled 1T1R synapse capable of low-power STDP via burst-mode communication and real-time unsupervised machine learning
2016 IEEE Symposium on VLSI Technology(2016)
摘要
We present a new electronic synapse for neuromorphic computing consisting of a 1T1R structure based on HfO
2
RRAM technology, and capable of STDP and pattern learning. Power consumption is reduced by adopting short POST spike and burst-mode integration. MNIST classification shows promising learning and classification efficiency. These results support RRAM as an enabling technology for low-power neuromorphic hardware.
更多查看译文
关键词
electronic synapse,neuromorphic computing,1T1R structure,HfO2 RRAM technology,STDP,pattern learning,power consumption,short POST spike,burst-mode integration,MNIST classification,low-power neuromorphic hardware,burst-mode communication,real-time unsupervised machine learning,HfO2
AI 理解论文
溯源树
样例
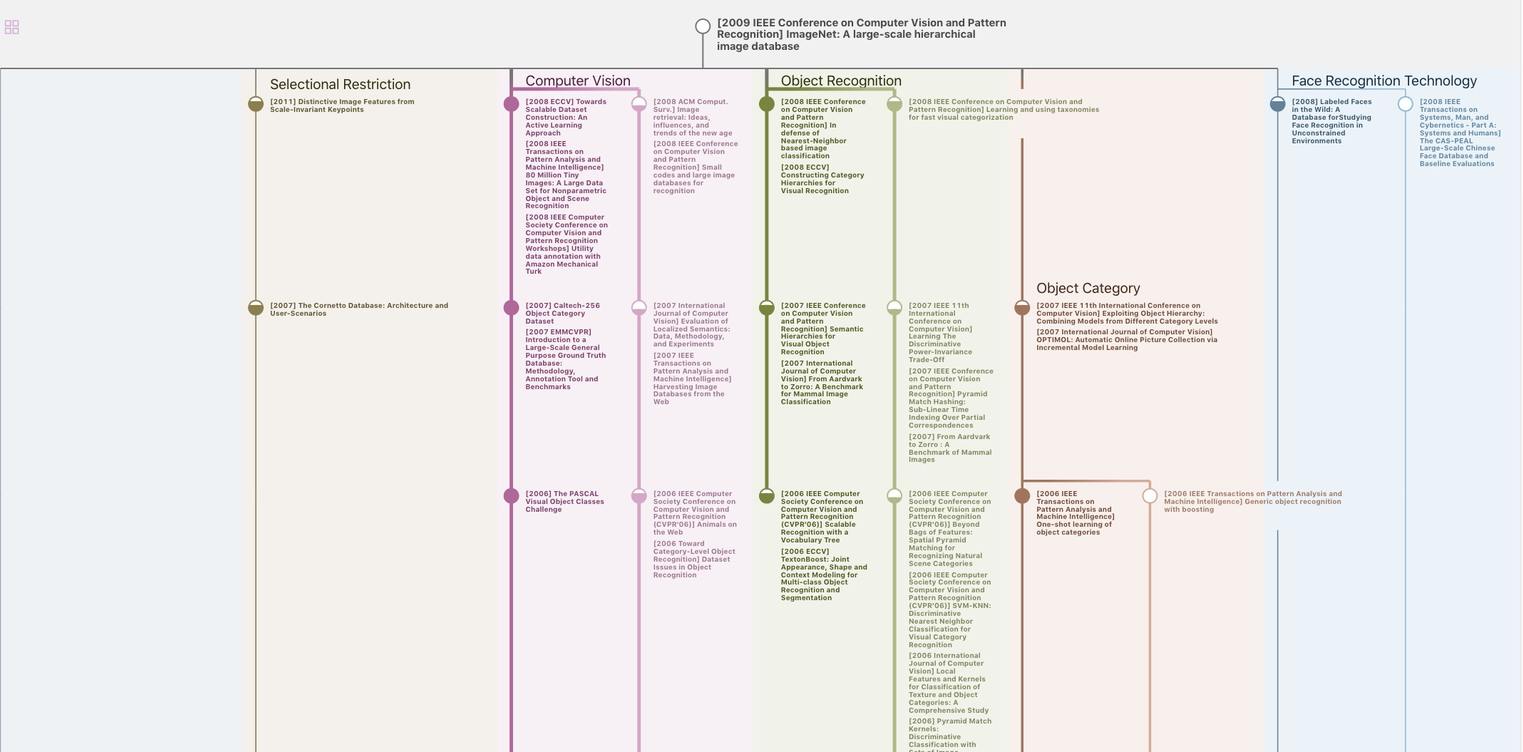
生成溯源树,研究论文发展脉络
Chat Paper
正在生成论文摘要