Rapid learning-based video stereolization using graphic processing unit acceleration
JOURNAL OF ELECTRONIC IMAGING(2016)
摘要
Video stereolization has received much attention in recent years due to the lack of stereoscopic three-dimensional (3-D) contents. Although video stereolization can enrich stereoscopic 3-D contents, it is hard to achieve automatic two-dimensional-to-3-D conversion with less computational cost. We proposed rapid learning-based video stereolization using a graphic processing unit (GPU) acceleration. We first generated an initial depth map based on learning from examples. Then, we refined the depth map using saliency and cross-bilateral filtering to make object boundaries clear. Finally, we performed depth-image-based-rendering to generate stereoscopic 3-D views. To accelerate the computation of video stereolization, we provided a parallelizable hybrid GPU-central processing unit (CPU) solution to be suitable for running on GPU. Experimental results demonstrate that the proposed method is nearly 180 times faster than CPU-based processing and achieves a good performance comparable to the-state-of-the-art ones. (C) 2016 SPIE and IS&T
更多查看译文
关键词
two-dimensional-to-three-dimensional conversion,depth-image-based-rendering,graphic processing unit acceleration,learning from examples,visual saliency,video stereolization
AI 理解论文
溯源树
样例
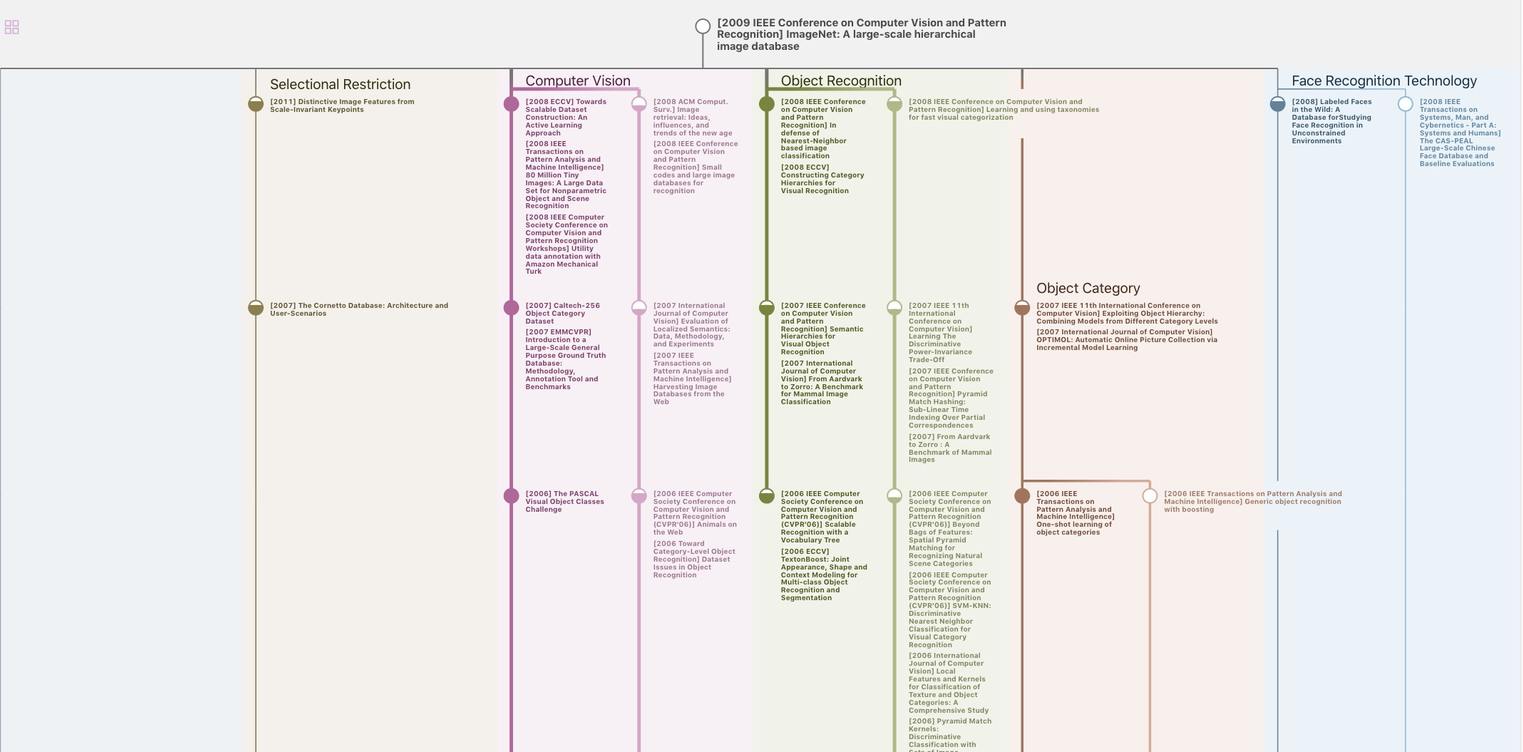
生成溯源树,研究论文发展脉络
Chat Paper
正在生成论文摘要