Cellular Automata Modeling of Land-Use/Land-Cover Dynamics: Questioning the Reliability of Data Sources and Classification Methods
ANNALS OF THE AMERICAN ASSOCIATION OF GEOGRAPHERS(2016)
摘要
Based on four time intervals within a forty-year period of observation, we construct land-use/land-cover (LULC) maps and estimate the transition probabilities between six LULC states. The maps and transition probability matrices (TPMs) were built based on the high-resolution aerial photos and 30-m multispectral Landsat images for the same years. We considered the TPM constructed from manual classification of the aerial photos as a reference and compared it to the TPM constructed from the Landsat image classified with several methods: mean-shift segmentation followed by random forest classification and three pixel-based methods popular in cellular automata (CA) studies: K-means, iterative self-organizing data analysis techniques (ISO-DATA), and maximum likelihood. For each classification method, the TPMs were constructed and compared to the TPMs for the aerial photos. We prove that the goodness-of-fit of maps obtained with the three pixel-based methods was insufficient for estimating the LULC TPM. The LULC maps obtained with the object-based classification fit well to those based on the aerial photos, but the estimates of TPM were yet qualitatively different. This article raises doubts regarding the adequacy of Landsat data and standard classification methods for establishing LULC CA model rules and calls for the careful reexamination of the entire land-use CA framework. We appeal for a new view of the CA modeling methodology: It should be based on a long-term series of carefully validated LULC maps that portray different types of land-use dynamics and land planning systems over long and representative periods of population and economic growth.
更多查看译文
关键词
cellular automata,Landsat images,land-use/land-cover changes,Markov transition probabilities matrices,validation of remote sensing classification methods
AI 理解论文
溯源树
样例
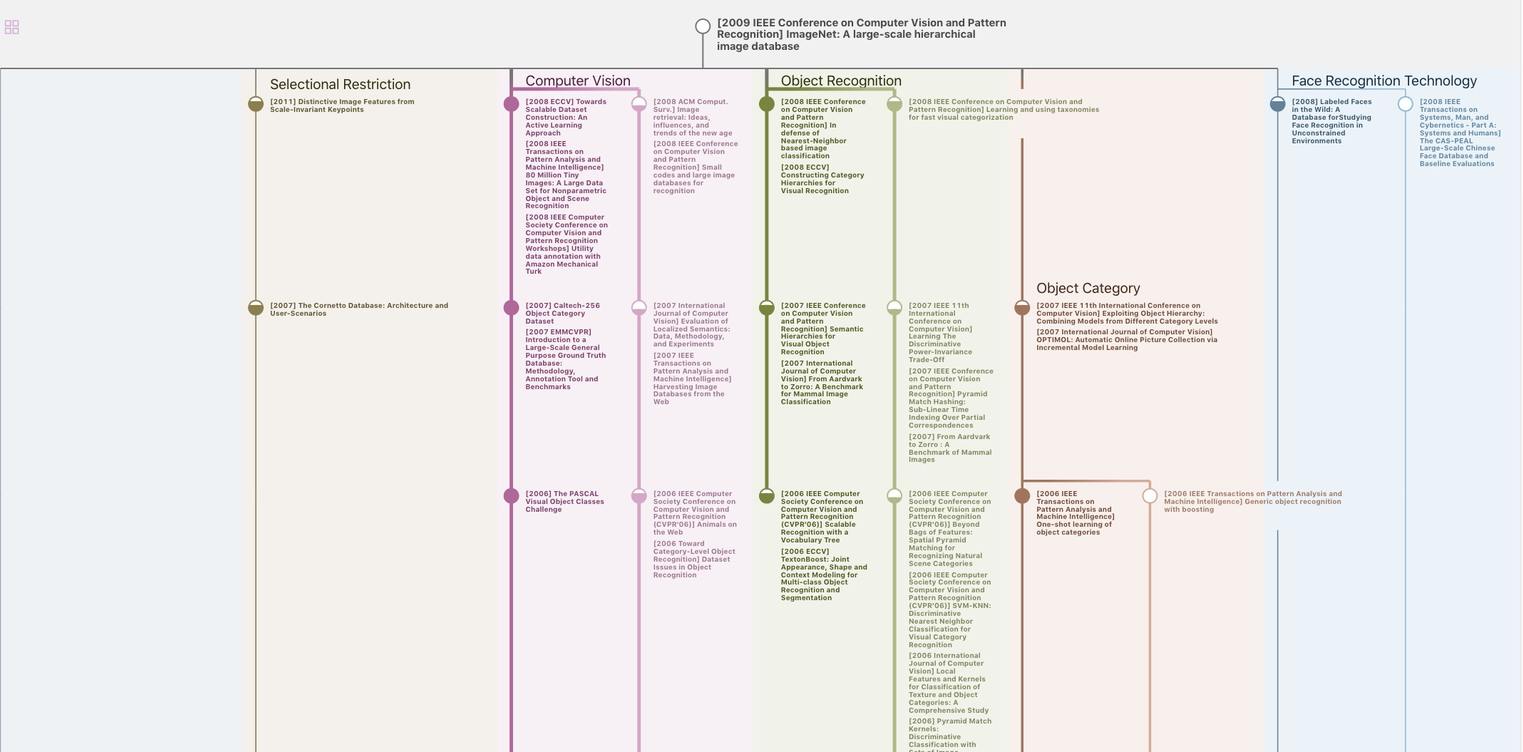
生成溯源树,研究论文发展脉络
Chat Paper
正在生成论文摘要