An Efficient Non-Intrusive Uncertainty Propagation Method for Stochastic Multi-Physics Models
arXiv: Numerical Analysis(2014)
摘要
Multi-physics models governed by coupled partial differential equation (PDE) systems, are naturally suited for partitioned, or modular numerical solution strategies. Although widely used in tackling deterministic coupled models, several challenges arise in extending the benefits of modularization to uncertainty propagation. On one hand, Monte-Carlo (MC) based methods are prohibitively expensive as the cost of each deterministic PDE solve is usually quite large, while on the other hand, even if each module contains a moderate number of uncertain parameters, implementing spectral methods on the combined high-dimensional parameter space can be prohibitively expensive. In this work, we present a reduced non-intrusive spectral projection (NISP) based uncertainty propagation method which separates and modularizes the uncertainty propagation task in each subproblem using block Gauss-Seidel (BGS) techniques. The overall computational costs in the proposed method are also mitigated by constructing reduced approximations of the input data entering each module. These reduced approximations and the corresponding quadrature rules are constructed via simple linear algebra transformations. We describe these components of the proposed algorithm assuming a generalized polynomial chaos (gPC) model of the stochastic solutions. We demonstrate our proposed method and its computational gains over the standard NISP method using numerical examples.
更多查看译文
AI 理解论文
溯源树
样例
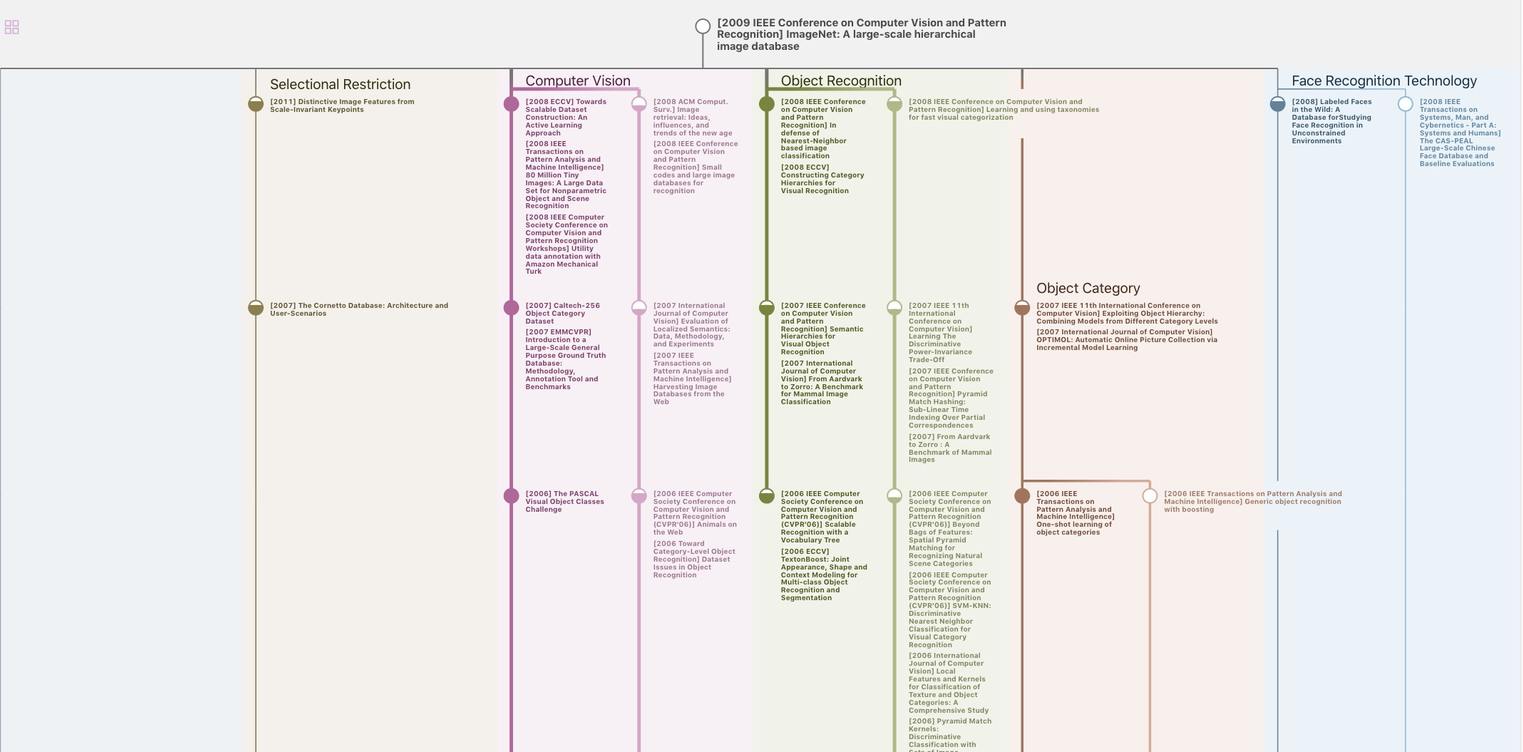
生成溯源树,研究论文发展脉络
Chat Paper
正在生成论文摘要