A Tighter Bound on the Uniqueness of Sparsely-Used Dictionaries
2016 SECOND INTERNATIONAL CONFERENCE ON COMPUTATIONAL INTELLIGENCE & COMMUNICATION TECHNOLOGY (CICT)(2016)
摘要
In this paper, we consider the sparsely used dictionary learning problem and focus on the case that the dictionary is square with arbitrary entries and the coefficient matrix is a sparse with random entries, which is first proposed and studied by Wang and Spielman et al.[1], [2]. We improve the theoretical results with a tighter bounded uniqueness theorem, which says that O(n) samples are sufficient to uniquely determine the decomposition with probability one when given assumption is satisfied, and the proof is given.
更多查看译文
关键词
Dictionary Learning, Uniqueness, Probalistic model, Tighter bound
AI 理解论文
溯源树
样例
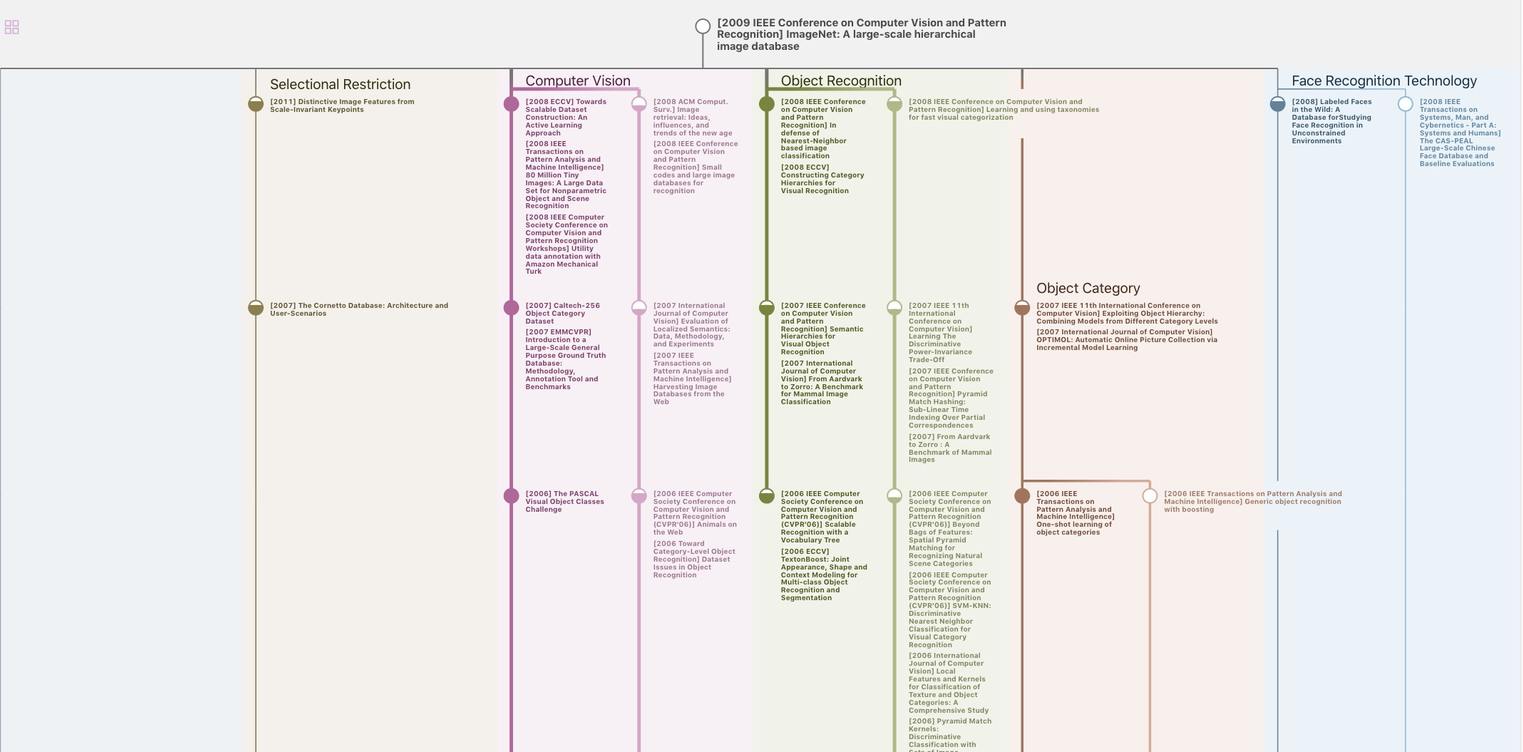
生成溯源树,研究论文发展脉络
Chat Paper
正在生成论文摘要