Template-Based Models for Genome-Wide Analysis of Next-Generation Sequencing Data at Base-Pair Resolution
JOURNAL OF THE AMERICAN STATISTICAL ASSOCIATION(2016)
摘要
We consider the problem of estimating the genome-wide distribution of nucleosome positions from paired end sequencing data. We develop a modeling approach based on nonparametric templates to control for the variability along the sequence of read counts associated with nucleosomal DNA due to enzymatic digestion and other sample preparation steps, and we develop a calibrated Bayesian method to detect local concentrations of nucleosome positions. We also introduce a set of estimands that provides rich, interpretable summaries of nucleosorne positioning. Inference is carried out via a distributed Hamiltonian Monte Carlo algorithm that can scale linearly with the length of the genome being analyzed. We provide MPI-based Python implementations of the proposed methods, stand-alone and on Amazon EC2, which can provide inferences on an entire Saccharomyces cerevisiae genome in less than 1 hr on EC2. We evaluate the accuracy and reproducibility of the inferences leveraging a factorially designed simulation study and experimental replicates. The template-based approach we develop here is also applicable to single-end sequencing data by using alternative sources of fragment length information, and to ordered and sequential data more generally. It provides a flexible and scalable alternative to mixture models, hidden Markov models, and Parzen-window methods. Supplementary materials for this article are available online.
更多查看译文
关键词
Calibrated Bayesian detection,Deconvolution,Hamiltonian Monte Carlo,Massive data,Measurement error,Nucleosomes,Parallel computation,Yeast
AI 理解论文
溯源树
样例
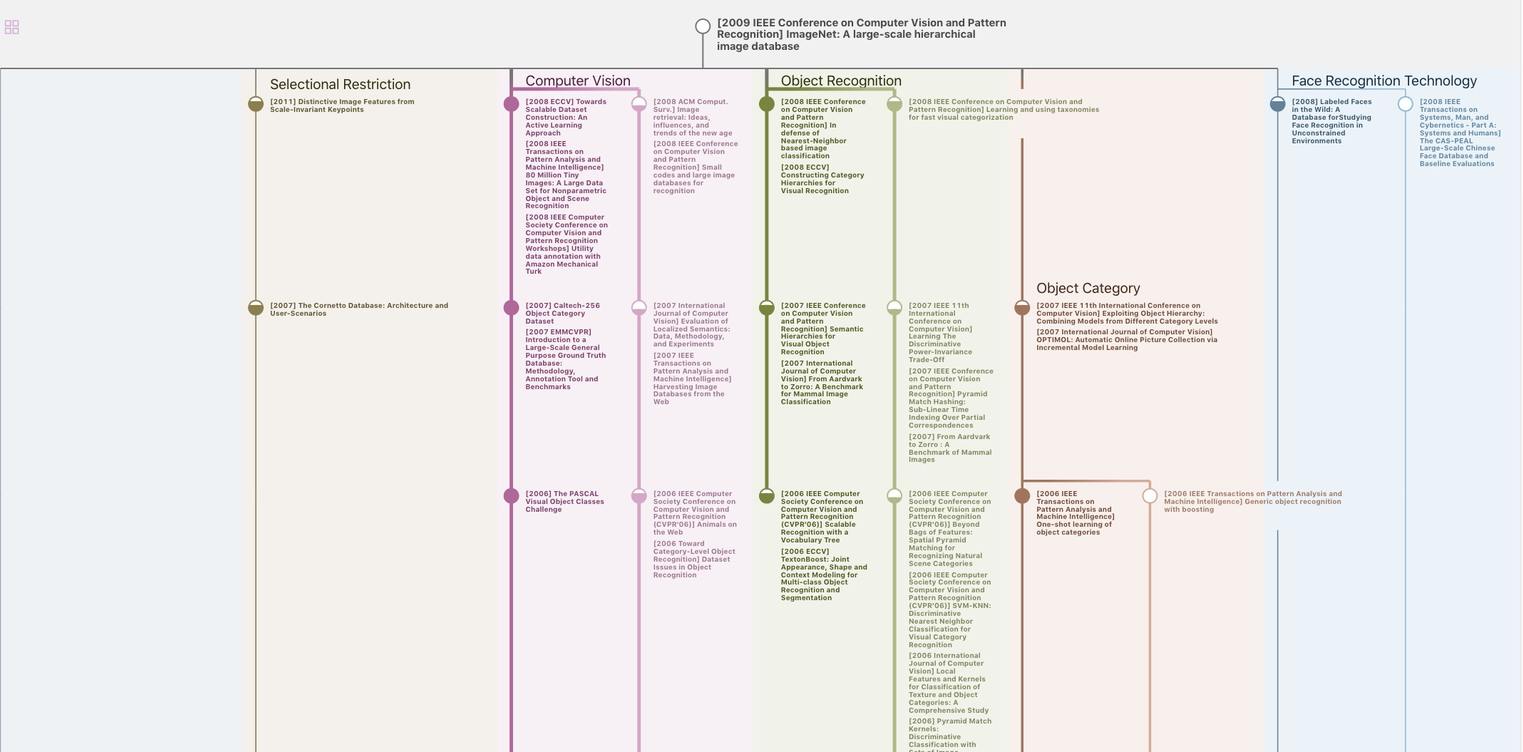
生成溯源树,研究论文发展脉络
Chat Paper
正在生成论文摘要