Predictive Scheduling For Electric Vehicles Considering Uncertainty Of Load And User Behaviors
2016 IEEE/PES TRANSMISSION AND DISTRIBUTION CONFERENCE AND EXPOSITION (T&D)(2016)
摘要
Un-coordinated Electric Vehicle (EV) charging can create unexpected load in local distribution grid, which may degrade the power quality and system reliability. The uncertainty of EV load, user behaviors and other baseload in distribution grid, is one of challenges that impedes optimal control for EV charging problem. Previous researches did not fully solve this problem due to lack of real-world EV charging data and proper stochastic model to describe these behaviors. In this paper, we propose a new predictive EV scheduling algorithm (PESA) inspired by Model Predictive Control (MPC), which includes a dynamic load estimation module and a predictive optimization module. The user-related EV load and base load are dynamically estimated based on the historical data. At each time interval, the predictive optimization program will be computed for optimal schedules given the estimated parameters. Only the first element from the algorithm outputs will be implemented according to MPC paradigm. Current-multiplexing function in each Electric Vehicle Supply Equipment (EVSE) is considered and accordingly a virtual load is modeled to handle the uncertainties of future EV energy demands. This system is validated by the real-world EV charging data collected on UCLA campus and the experimental results indicate that our proposed model not only reduces load variation up to 40% but also maintains a high level of robustness. Finally, IEC 61850 standard is utilized to standardize the data models involved, which brings significance to more reliable and large-scale implementation.
更多查看译文
关键词
EV Scheduling, Predictive Control, IEC 61850, Renewable Energy Integration
AI 理解论文
溯源树
样例
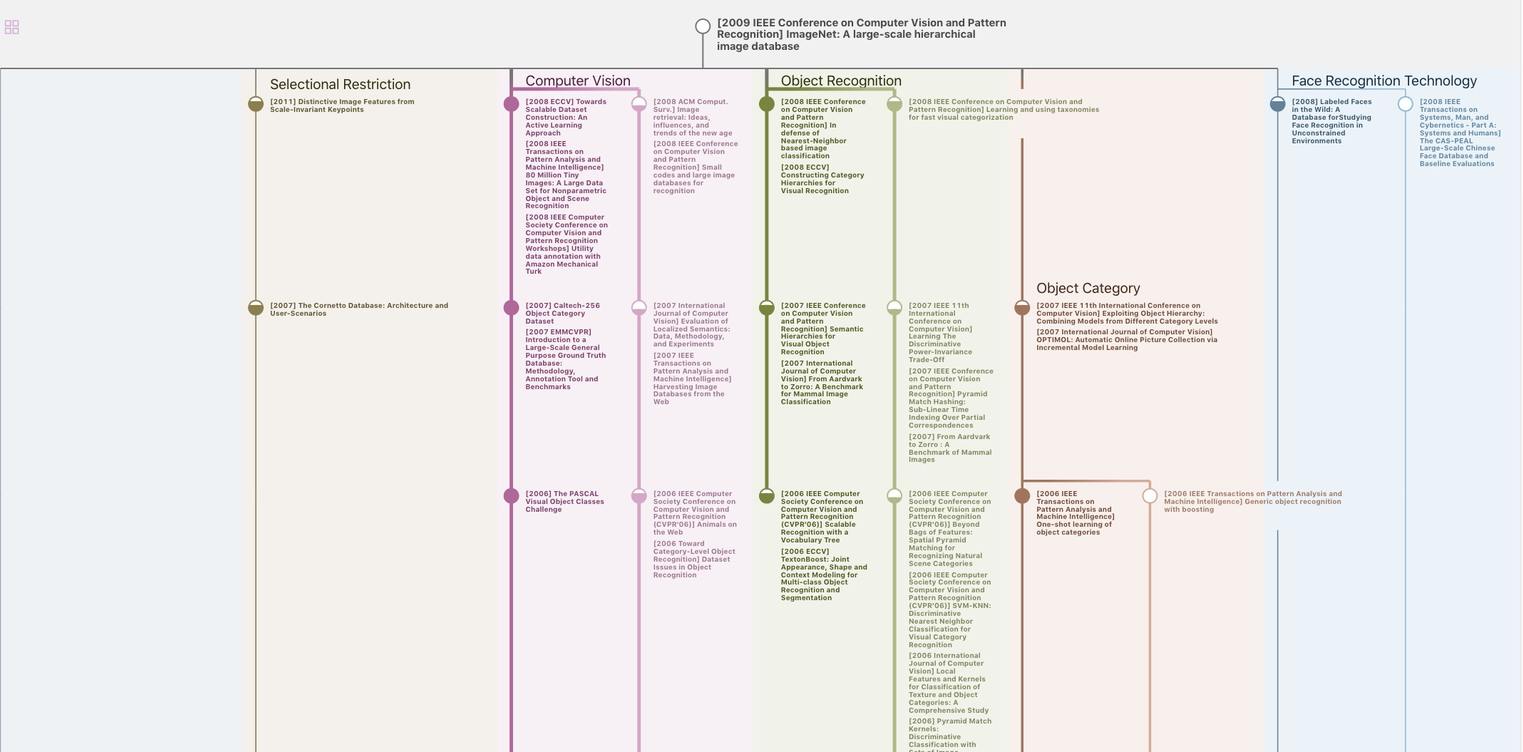
生成溯源树,研究论文发展脉络
Chat Paper
正在生成论文摘要