Structure-Based Prediction Of Protease Multispecificity Using Computational Protein Design
BIOPHYSICAL JOURNAL(2016)
摘要
The substrate specificity of protease enzymes constitutes the molecular basis of their diverse and complex biological roles. Rapid and accurate prediction of extended substrate specificity would also enable the design of custom enzymes capable of selectively cleaving biotechnologically or therapeutically relevant proteins. However, current in silico approaches for protease specificity prediction rely on, and are therefore limited by, machine learning of sequence patterns in known experimental data. Here, we describe a general structure-based approach for predicting peptidase substrates. We develop a structure-guided sequence sampling technique using self-consistent mean field theory for rapid specificity profile prediction and implement it in the Rosetta software. We show that computationally calculated specificity profiles closely match experimentally determined ones. Further, we construct atomic resolution models of thousands of substrate-enzyme complexes for each of six model proteases belonging to the four major protease mechanistic classes, and develop a discriminatory scoring function using enzyme design modules from Rosetta and Amber-MMPBSA. We rank putative peptide substrates in order of their interaction energies with a modeled near attack conformation of the enzyme active site. We show that the energetic patterns obtained from these simulations can be used to robustly classify known cleaved and uncleaved peptides, and these patterns have greater discriminatory power compared to purely sequence-based statistical inference. Combining sequence and energetic patterns for a given protease using machine-learning algorithms further improves classification performance, and analysis of atomic resolution models affords physical insight into the structural basis for the observed specificities. We tested the predictive capability of the approach by designing and experimentally characterizing the cleavage of four previously unidentified, but less rapidly cleaved, novel substrates for the Hepatitis C virus NS3/4 protease using an in vivo assay.
更多查看译文
AI 理解论文
溯源树
样例
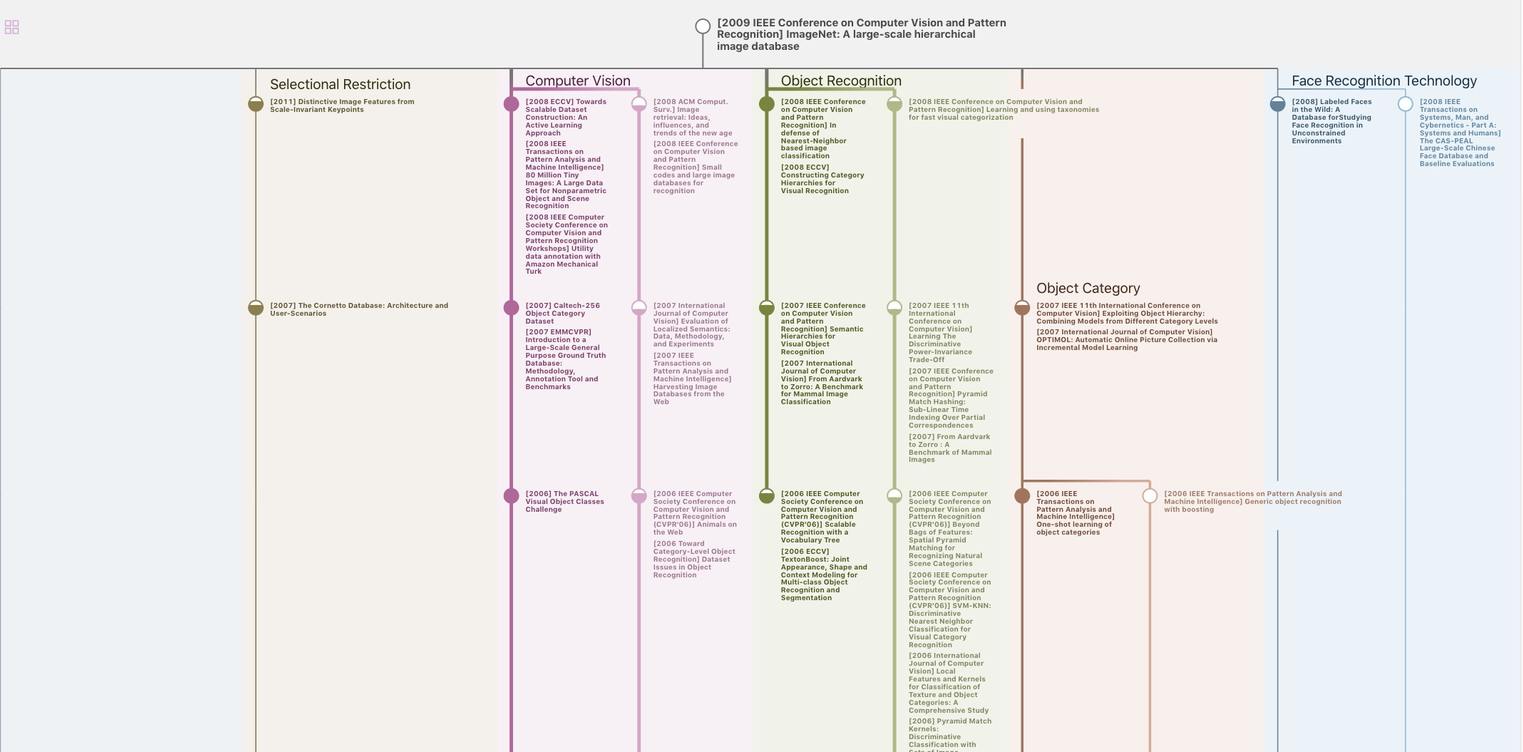
生成溯源树,研究论文发展脉络
Chat Paper
正在生成论文摘要