Flight Considerations and Hyperspectral Image Classifications for Dryland Vegetation Management from a Fixed-wing UAS
Environmental Management and Sustainable Development(2016)
摘要
Unmanned Aerial Systems (UAS)-based hyperspectral remote sensing capabilities developed by the Idaho National Lab and Boise Center Aerospace Lab were tested via demonstration flights that explored the influence of altitude on geometric error, image mosaicking, and dryland vegetation classification. The motivation for this study was to better understand the challenges associated with UAS-based hyperspectral data for distinguishing native grasses such as Sandberg bluegrass ( Poa secunda ) from invasives such as burr buttercup ( Ranunculus testiculatus) in a shrubland environment. The test flights successfully acquired usable flightline data capable of supporting classifiable composite images. Unsupervised classification results support vegetation management objectives that rely on mapping shrub cover and distribution patterns. However, supervised classifications performed poorly despite spectral separability in the image-derived endmember pixels. In many cases, the supervised classifications accentuated noise or features in the mosaic that were artifacts of color balancing and feathering in areas of flightline overlap. Future UAS flight missions that optimize flight planning; minimize illumination differences between flightlines; and leverage ground reference data and time series analysis should be able to effectively distinguish native grasses such as Sandberg bluegrass from burr buttercup.
更多查看译文
AI 理解论文
溯源树
样例
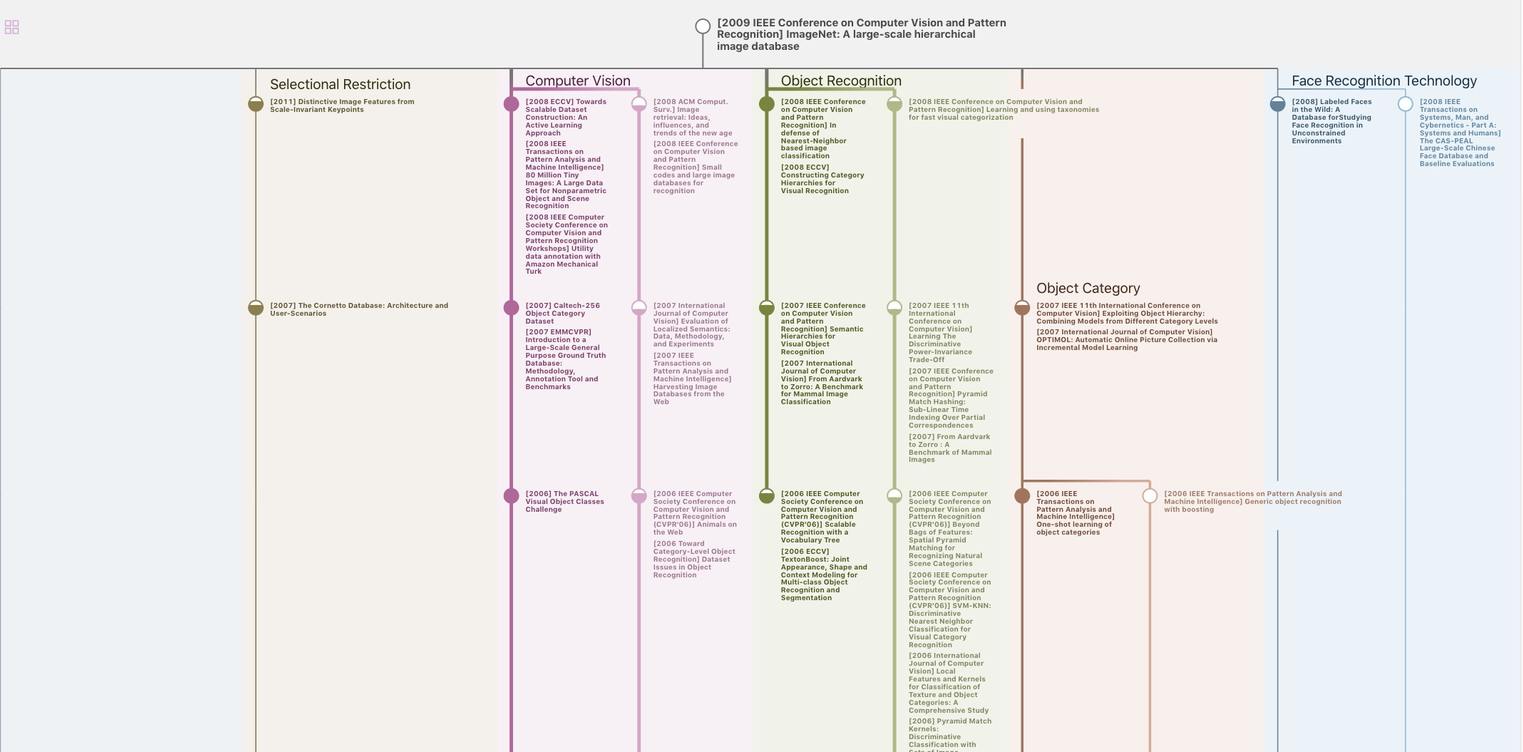
生成溯源树,研究论文发展脉络
Chat Paper
正在生成论文摘要