Improved quantum-behaved particle swarm optimization with local search strategy:
JOURNAL OF ALGORITHMS & COMPUTATIONAL TECHNOLOGY(2017)
摘要
Quantum-behaved particle swarm optimization, which was motivated by analysis of particle swarm optimization and quantum system, has shown compared performance in finding the optimal solutions for many optimization problems to other evolutionary algorithms. To address the problem of premature, a local search strategy is proposed to improve the performance of quantum-behaved particle swarm optimization. In proposed local search strategy, a super particle is presented which is a collection body of randomly selected particles' dimension information in the swarm. The selected probability of particles in swarm is different and determined by their fitness values. To minimization problems, the fitness value of one particle is smaller; the selected probability is more and will contribute more information in constructing the super particle. In addition, in order to investigate the influence on algorithm performance with different local search space, four methods of computing the local search radius are applied in local search strategy and propose four variants of local search quantum-behaved particle swarm optimization. Empirical studies on a suite of well-known benchmark functions are undertaken in order to make an overall performance comparison among the proposed methods and other quantum-behaved particle swarm optimization. The simulation results show that the proposed quantum-behaved particle swarm optimization variants have better advantages over the original quantum-behaved particle swarm optimization.
更多查看译文
关键词
Particle swarm optimization,quantum-behaved particle swarm optimization,local search,search radius
AI 理解论文
溯源树
样例
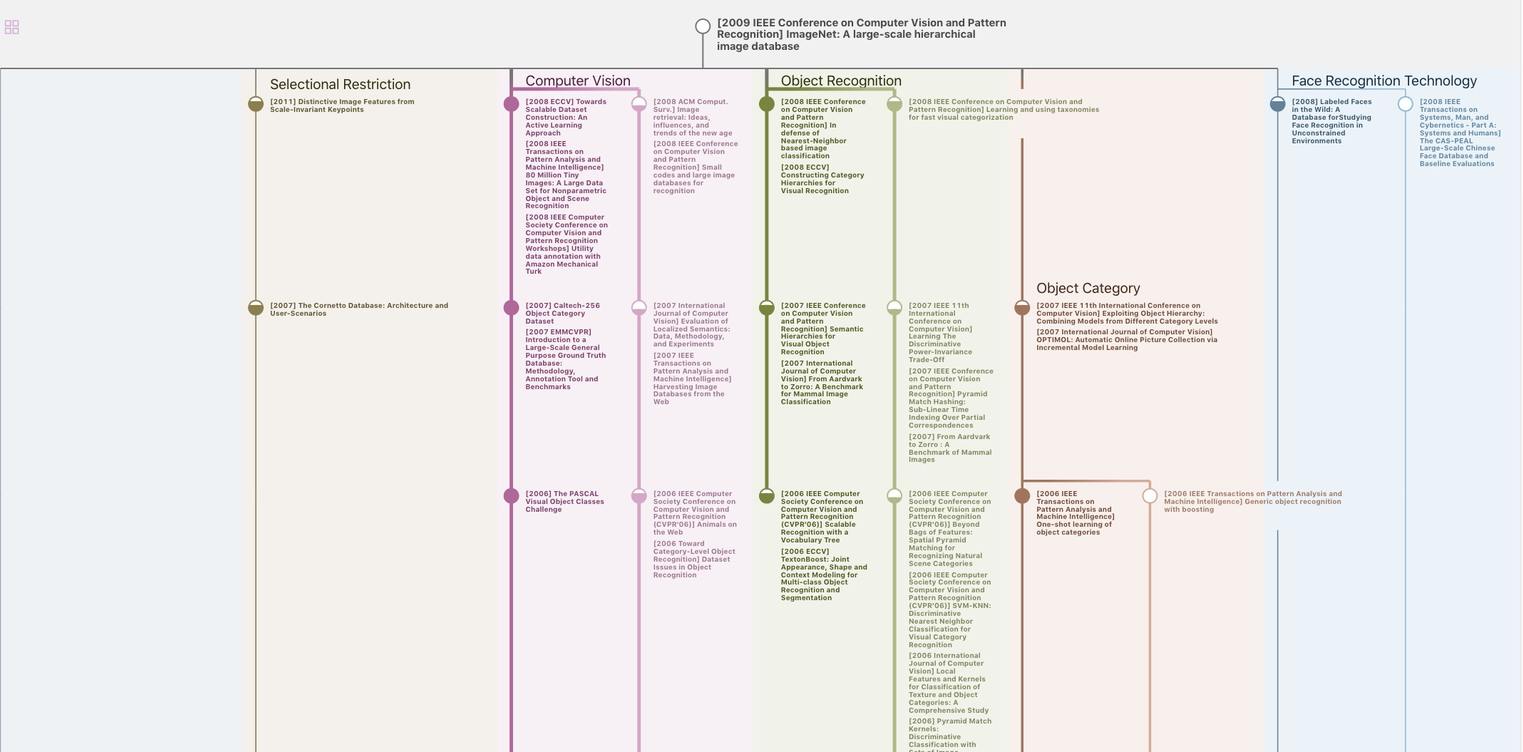
生成溯源树,研究论文发展脉络
Chat Paper
正在生成论文摘要