Implementing and Automating Fixed-Form Variational Posterior Approximation through Stochastic Linear Regression
arXiv: Computation(2014)
摘要
We recently proposed a general algorithm for approximating nonstandard Bayesian posterior distributions by minimization of their Kullback-Leibler divergence with respect to a more convenient approximating distribution. In this note we offer details on how to efficiently implement this algorithm in practice. We also suggest default choices for the form of the posterior approximation, the number of iterations, the step size, and other user choices. By using these defaults it becomes possible to construct good posterior approximations for hierarchical models completely automatically.
更多查看译文
AI 理解论文
溯源树
样例
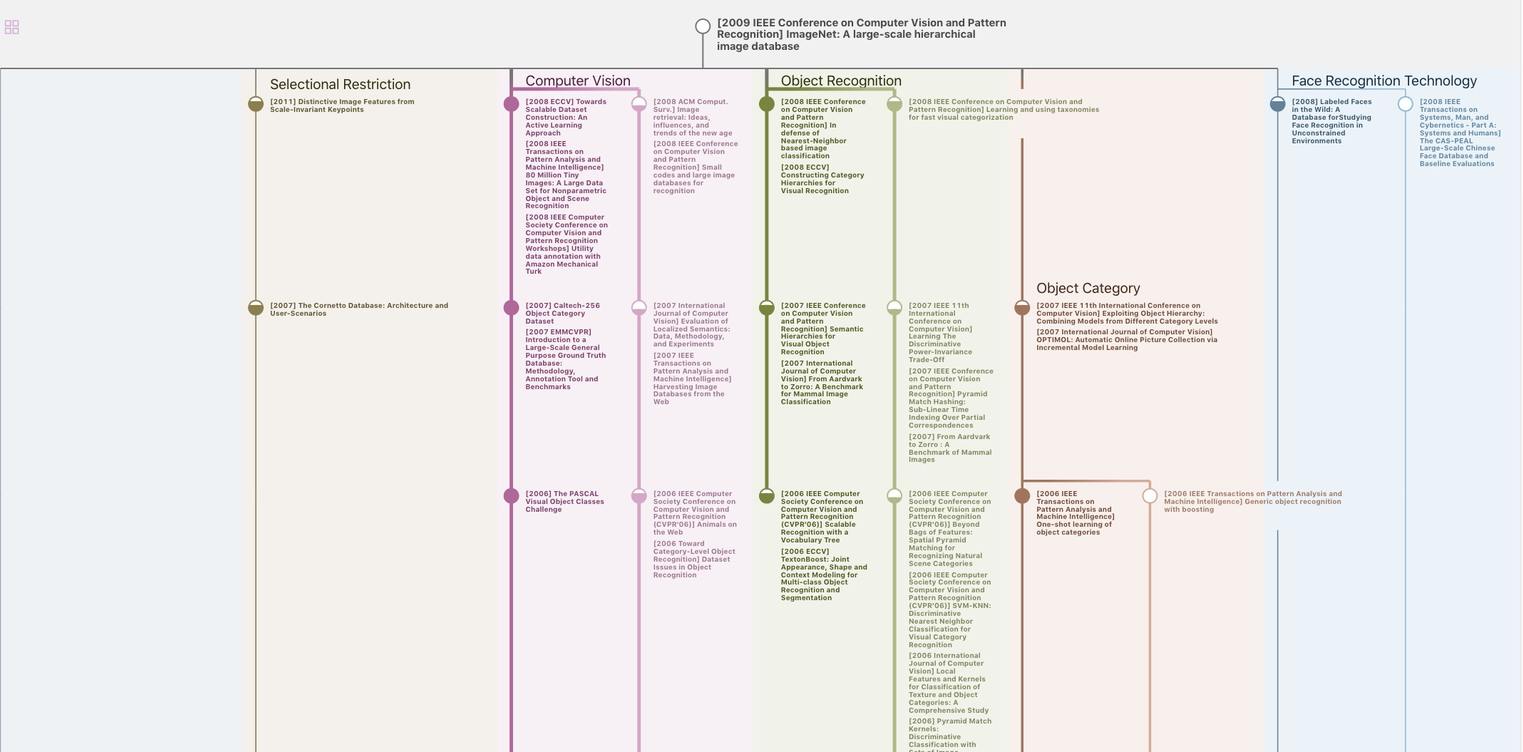
生成溯源树,研究论文发展脉络
Chat Paper
正在生成论文摘要