Multilinear Filtering Based on a Hierarchical Structure of Covariance Matrices
Schedae Informaticae(2015)
摘要
We propose a novel model of multilinear filtering based on a hierar- chical structure of covariance matrices - each matrix being extracted from the input tensor in accordance to a specific set-theoretic model of data generaliza- tion, such as derivation of expectation values. The experimental analysis results presented in this paper confirm that the investigated approaches to tensor-based data representation and processing outperform the standard collaborative filter- ing approach in the u0027cold-startu0027 personalized recommendation scenario (of very sparse input data). Furthermore, it has been shown that the proposed method is superior to standard tensor-based frameworks such as N-way Random Indexing (NRI) and Higher-Order Singular Value Decomposition (HOSVD) in terms of both the AUROC measure and computation time.
更多查看译文
AI 理解论文
溯源树
样例
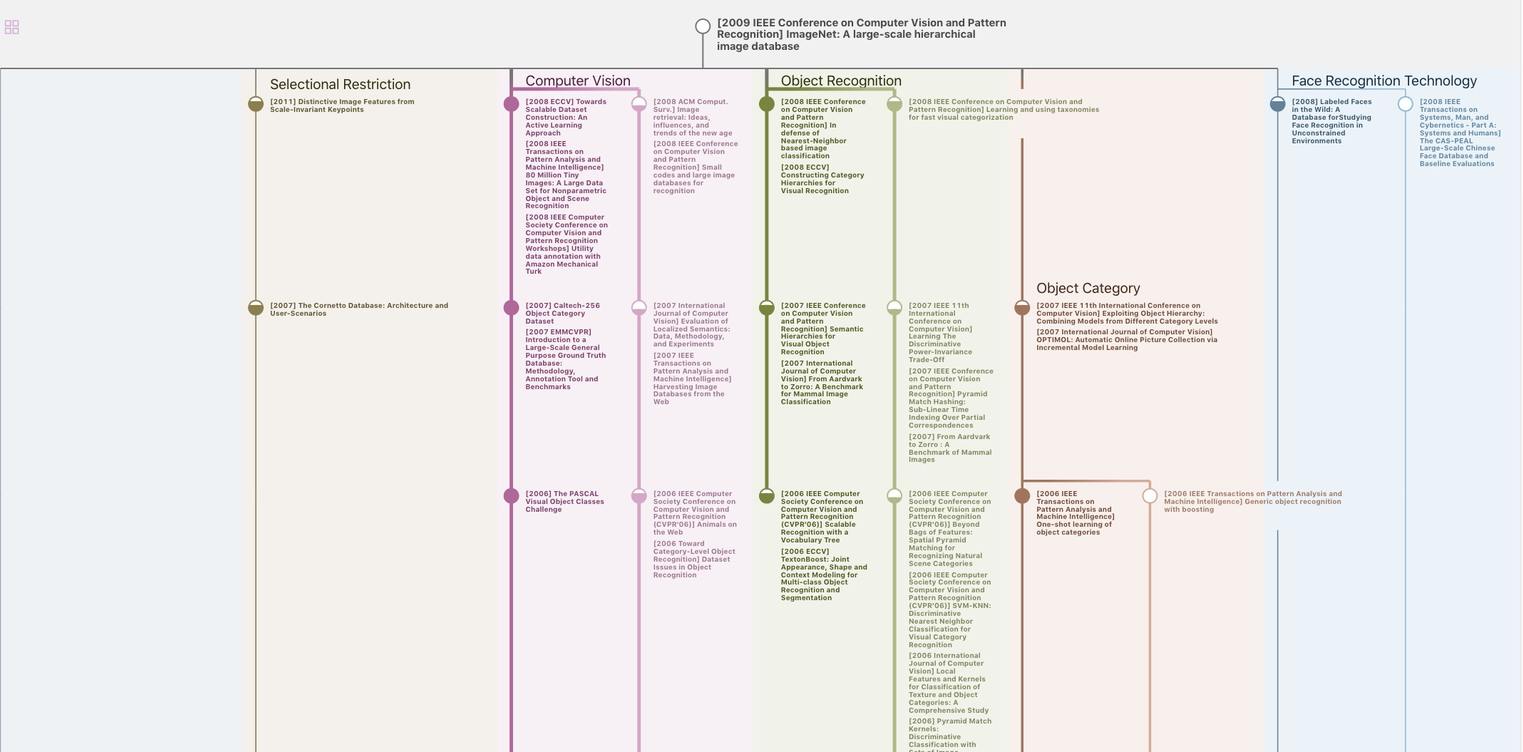
生成溯源树,研究论文发展脉络
Chat Paper
正在生成论文摘要