Developing Atmospheric Retrieval Methods for Direct Imaging Spectroscopy of Gas Giants in Reflected Light I: Methane Abundances and Basic Cloud Properties
ASTRONOMICAL JOURNAL(2016)
摘要
Upcoming space-based coronagraphic instruments in the next decade will perform reflected light spectroscopy and photometry of cool directly imaged extrasolar giant planets. We are developing a new atmospheric retrieval methodology to help assess the science return and inform the instrument design for such future missions, and ultimately interpret the resulting observations. Our retrieval technique employs a geometric albedo model coupled with both a Markov chain Monte Carlo Ensemble Sampler (emcee) and a multimodal nested sampling algorithm (MultiNest) to map the posterior distribution. This combination makes the global evidence calculation more robust for any given model and highlights possible discrepancies in the likelihood maps. As a proof of concept, our current atmospheric model contains one or two cloud layers, methane as a major absorber, and a H-2-He background gas. This 6-to-9 parameter model is appropriate for Jupiter-like planets and can be easily expanded in the future. In addition to deriving the marginal likelihood distribution and confidence intervals for the model parameters, we perform model selection to determine the significance of methane and cloud detection as a function of expected signal-to-noise ratio in the presence of spectral noise correlations. After internal validation, the method is applied to realistic spectra of Jupiter, Saturn, and HD 99492c, a model observing target. We find that the presence or absence of clouds and methane can be determined with high confidence, while parameter uncertainties are model dependent and correlated. Such general methods will also be applicable to the interpretation of direct imaging spectra of cloudy terrestrial planets.
更多查看译文
关键词
methods: statistical,planets and satellites: atmospheres,planets and satellites: composition,techniques: spectroscopic
AI 理解论文
溯源树
样例
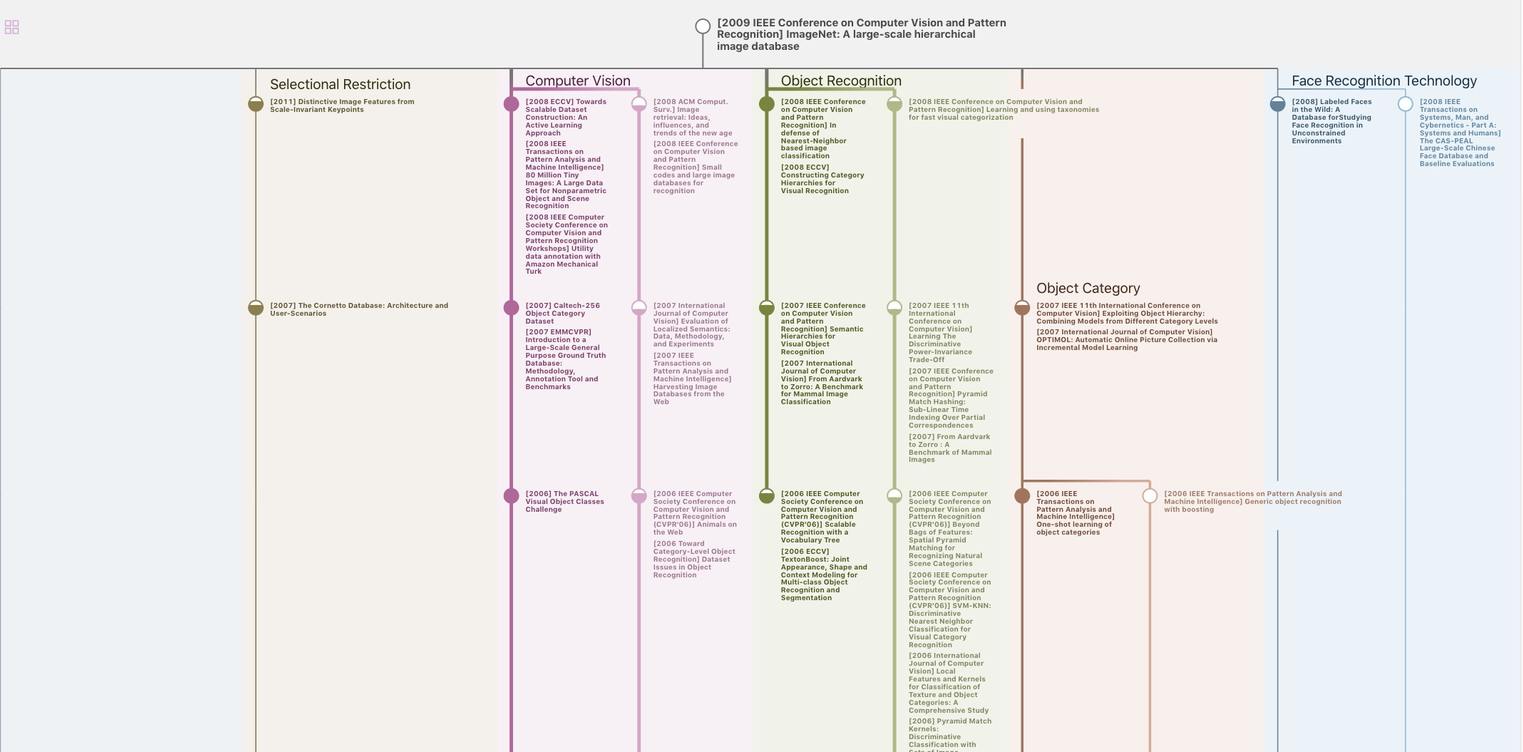
生成溯源树,研究论文发展脉络
Chat Paper
正在生成论文摘要