Best practices for safety improvement through high-volume institutional incident learning: lessons learned from 2 years
Journal of Radiation Oncology(2016)
摘要
Objective Incident learning systems (ILSs) are a key component of improving patient safety in radiation oncology, but the practicalities of ILS implementation can present major challenges. We describe the implementation and best practices derived from 2 years of experience with institutional incident learning, with details on root cause analysis (RCA), a list of key process improvements, and operational aspects of ILS use. Methods and materials The structure of the ILS is consistent with recommendations from the American Association of Physicists in Medicine (AAPM). Workflow is analyzed for incident reports from initial reporting to analysis and feedback to the reporter, including staffing required. A system for incident categorization is shown, as well as sample events selected for root cause analysis, and suggestions for providing feedback to users of ILS. Results In the first 2 years of the ILS implementation from 2012 to 2014, 1897 near-miss incidents were reported. There is widespread participation in the ILS program across all professional groups inside the department, with at least 75 % of clinical staff having filed at least one report. Total workload for the ILS program is estimated to be approximately one full-time employee, shared by approximately eight team members. Fifteen events were selected for RCA during this period. Eighteen major process improvement projects are described, ranging from issues related to process standardization, automation, staffing, new organization structures, and equipment purchase. Conclusions A unique high reporting volume institutional ILS has successfully resulted in numerous improvements in process, safety, and quality. Details for implementation and best practices for incident learning have been presented to allow adaptation in other practices.
更多查看译文
关键词
Patient safety, Incident learning, Quality improvement, Radiation oncology
AI 理解论文
溯源树
样例
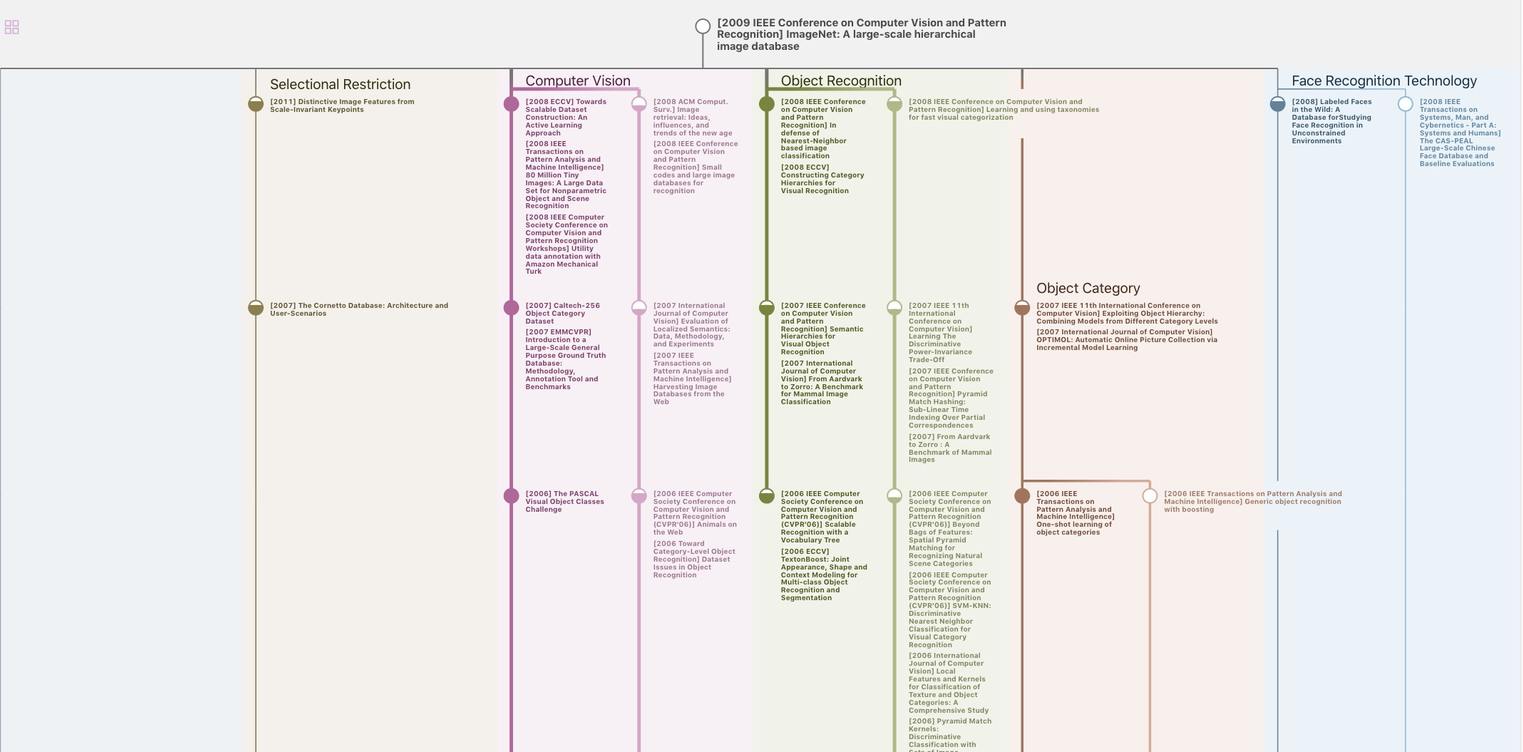
生成溯源树,研究论文发展脉络
Chat Paper
正在生成论文摘要