Asymptotic equivalence between the default Bayes factors and the ordinary Bayes factors with intrinsic priors
Journal of the Korean Statistical Society(2016)
摘要
In Bayesian model selection or testing problems, default priors are typically improper; that is, the resulting Bayes factor is not well defined. To circumvent this problem, two methodologies, namely, intrinsic and fractional Bayes factors are proposed and developed. Further, these two Bayes factors are asymptotically equivalent to the ordinary Bayes factors computed with proper priors called intrinsic priors. However, it seems that there are some necessary conditions to satisfy asymptotic equivalence. Such conditions are derived and justified in this article and illustrative examples are provided. Simulations are performed to demonstrate the results.
更多查看译文
关键词
primary,secondary
AI 理解论文
溯源树
样例
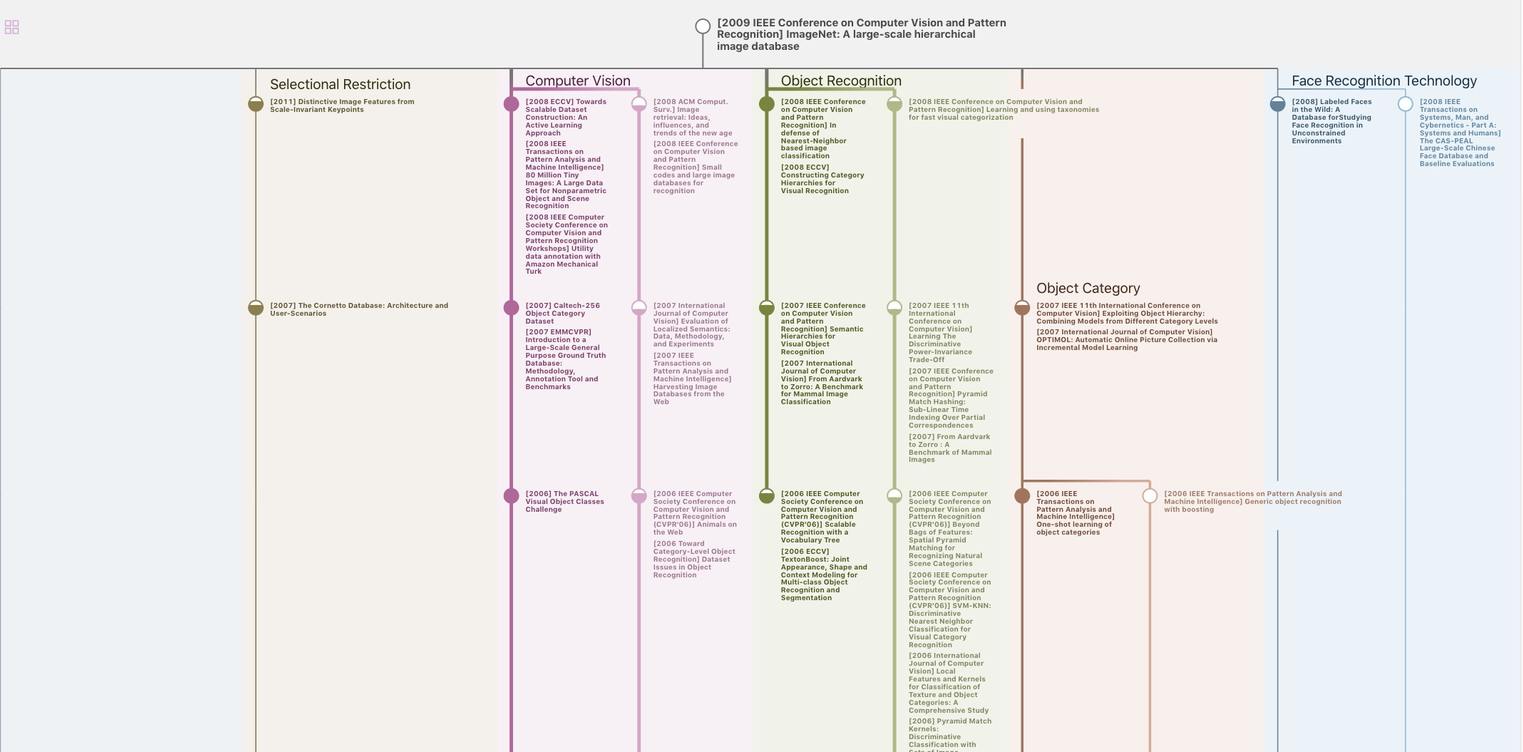
生成溯源树,研究论文发展脉络
Chat Paper
正在生成论文摘要