Fast community detection based on sector edge aggregation metric model in hyperbolic space
Physica A: Statistical Mechanics and its Applications(2016)
摘要
By studying the edge aggregation characteristic of nodes in hyperbolic space, Sector Edge Aggregation Metric (SEAM) model is proposed and theoretically proved in this paper. In hyperbolic disk SEAM model determines the minimum angular range of a sector which possesses the maximal edge aggregation of nodes. The set of nodes within such sector has dense internal links, which corresponds with the characteristic of community structure. Based on SEAM model, we propose a fast community detection algorithm called Greedy Optimization Modularity Algorithm (GOMA) which employs greedy optimization strategy and hyperbolic coordinates. GOMA firstly divides initial communities according to the quantitative results of sector edge aggregation given by SEAM and the nodes’ hyperbolic coordinates, then based on greedy optimization strategy, only merges the two angular neighboring communities in hyperbolic disk to optimize the network modularity function, and consequently obtains high-quality community detection. The strategies of initial community partition and merger in hyperbolic space greatly improve the speed of searching the most optimal modularity. Experimental results indicate that GOMA is able to detect out high-quality community structure in synthetic and real networks, and performs better when applied to the large-scale and dense networks with strong clustering.
更多查看译文
关键词
Edge aggregation metric,Community detection,Hyperbolic space,Complex network
AI 理解论文
溯源树
样例
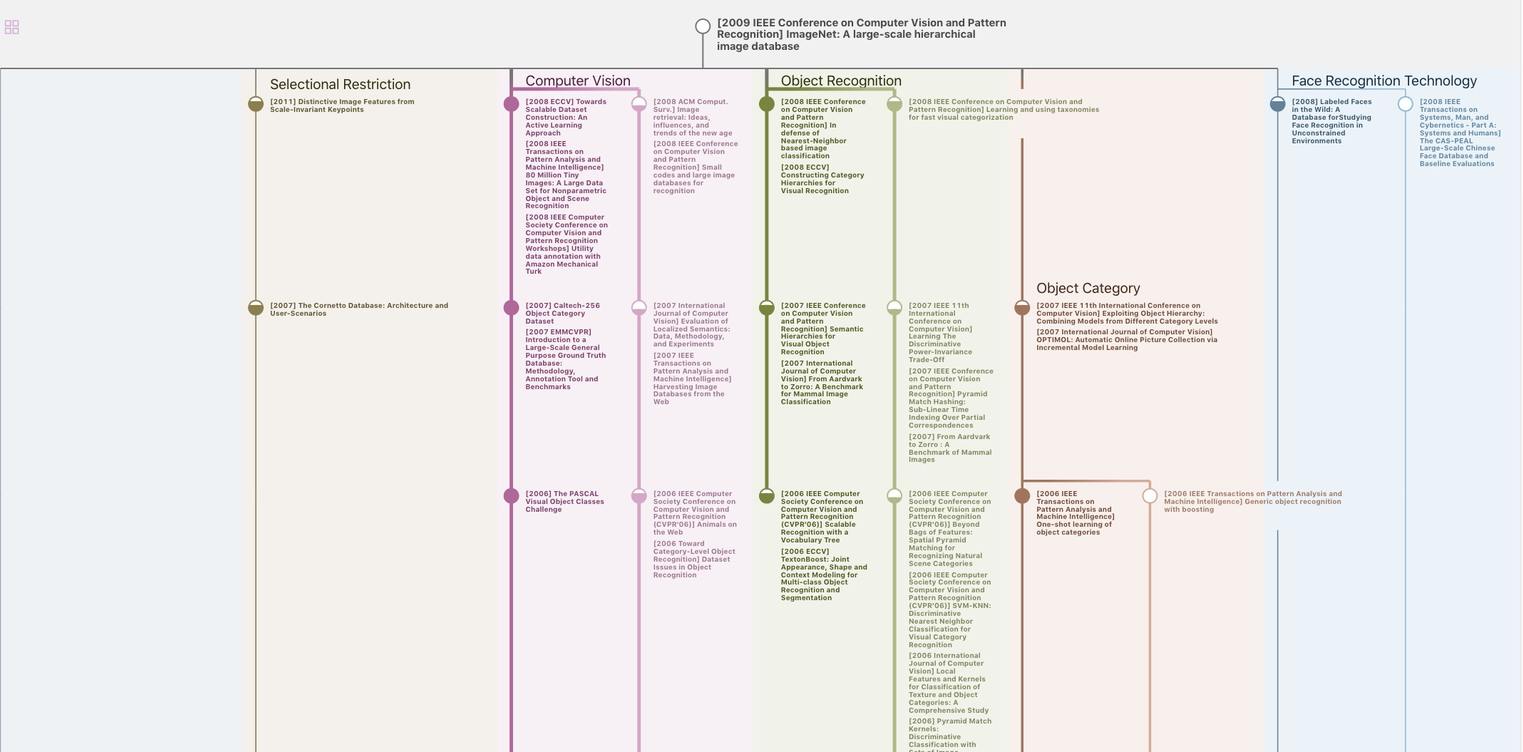
生成溯源树,研究论文发展脉络
Chat Paper
正在生成论文摘要