A systematic optimization approach for a class of statistical inference problems utilizing data augmentation
arXiv: Optimization and Control(2016)
摘要
We present an algorithm for a class of statistical inference problems. main idea is to reformulate the inference problem as an optimization procedure, based on the generation of surrogate (auxiliary) functions. This approach is motivated by the MM algorithm, combined with the systematic and iterative structure of the Expectation-Maximization algorithm. resulting algorithm can deal with hidden variables in Maximum Likelihood and Maximum a Posteriori estimation problems, Instrumental Variables, Regularized Optimization and Constrained Optimization problems. The advantage of the proposed algorithm is to provide a systematic procedure to build surrogate functions for certain kind of systems typically arising in communication and quantization applications, where hidden variables are usually involved. Numerical examples show the benefits of the proposed approach.
更多查看译文
AI 理解论文
溯源树
样例
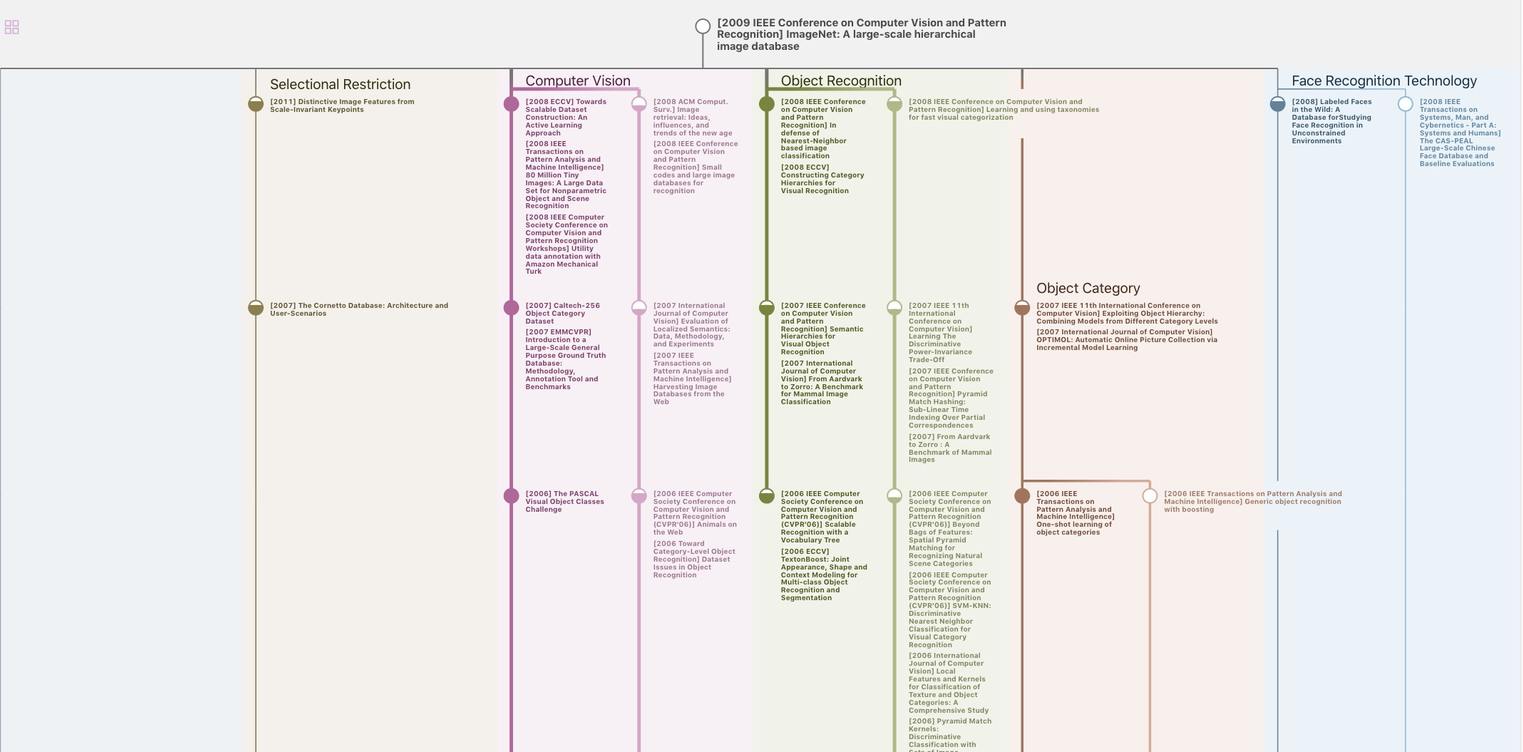
生成溯源树,研究论文发展脉络
Chat Paper
正在生成论文摘要