A dynamic programming algorithm for optimizing baseball strategies
MI Preprint Series(2019)
摘要
In this paper, baseball is formulated as a finite Markov game with approximately 6.45 million states. We give an effective dynamic programming algorithm which computes Markov perfect equilibria and the value functions of the game for both teams in 2 second per game. Optimal decision making can be found depending on the situation—for example, for the batting team, whether batting for a hit, stealing a base or sacrifice bunting will maximize their win percentage, or for the fielding team, whether to pitch to or intentionally walk a batter, yields optimal results. In addition, our algorithm makes it possible to compute the optimal batting order, in consideration of strategy optimization such as a sacrifice bunt or a stolen base. The authors believe that this baseball model is also useful as a benchmark instance for evaluating the performances of (multi-agent) Reinforcement Learning methods.
更多查看译文
关键词
baseball strategies,dynamic programming algorithm
AI 理解论文
溯源树
样例
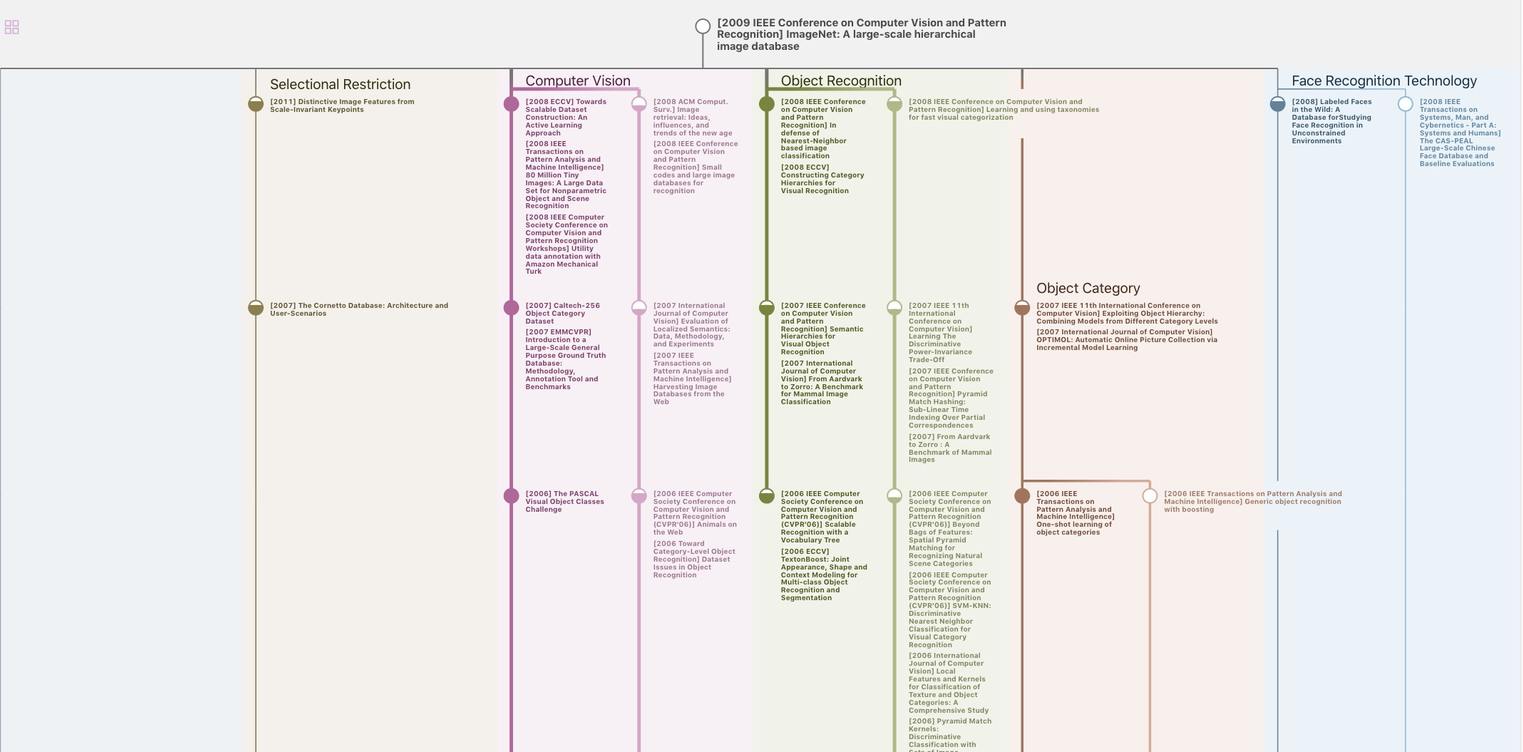
生成溯源树,研究论文发展脉络
Chat Paper
正在生成论文摘要