Classification of magnetic resonance brain images using bi-dimensional empirical mode decomposition and autoregressive model
Biomedical Engineering Letters(2016)
摘要
Purpose Automated classification of brain magnetic resonance (MR) images has been an extensively researched topic in biomedical image processing. In this work, we propose a new approach for classifying normal and abnormal brain MR images using bi-dimensional empirical mode decomposition (BEMD) and autoregressive (AR) model Methods In our approach, brain MR image is decomposed into four intrinsic mode functions (IMFs) using BEMD and AR coefficients from multiple IMFs are concatenated to form a feature vector. Finally a binary classifier, least-squares support vector machine (LS-SVM), is employed to discriminate between normal and abnormal brain MR images. Results The proposed technique achieves 100% classification accuracy using second-order AR model with linear and radial basis function (RBF) as kernels in LS-SVM. Conclusions Experimental results confirm that the performance of the proposed method is quite comparable with the existing results. Specifically, the presented approach outperforms one-dimensional empirical mode decomposition (1D-EMD) based classification of brain MR images.
更多查看译文
关键词
Magnetic resonance imaging (MRI), Bi-dimensional empirical mode decomposition (BEMD), Intrinsic mode function (IMF), Autoregressive (AR) model
AI 理解论文
溯源树
样例
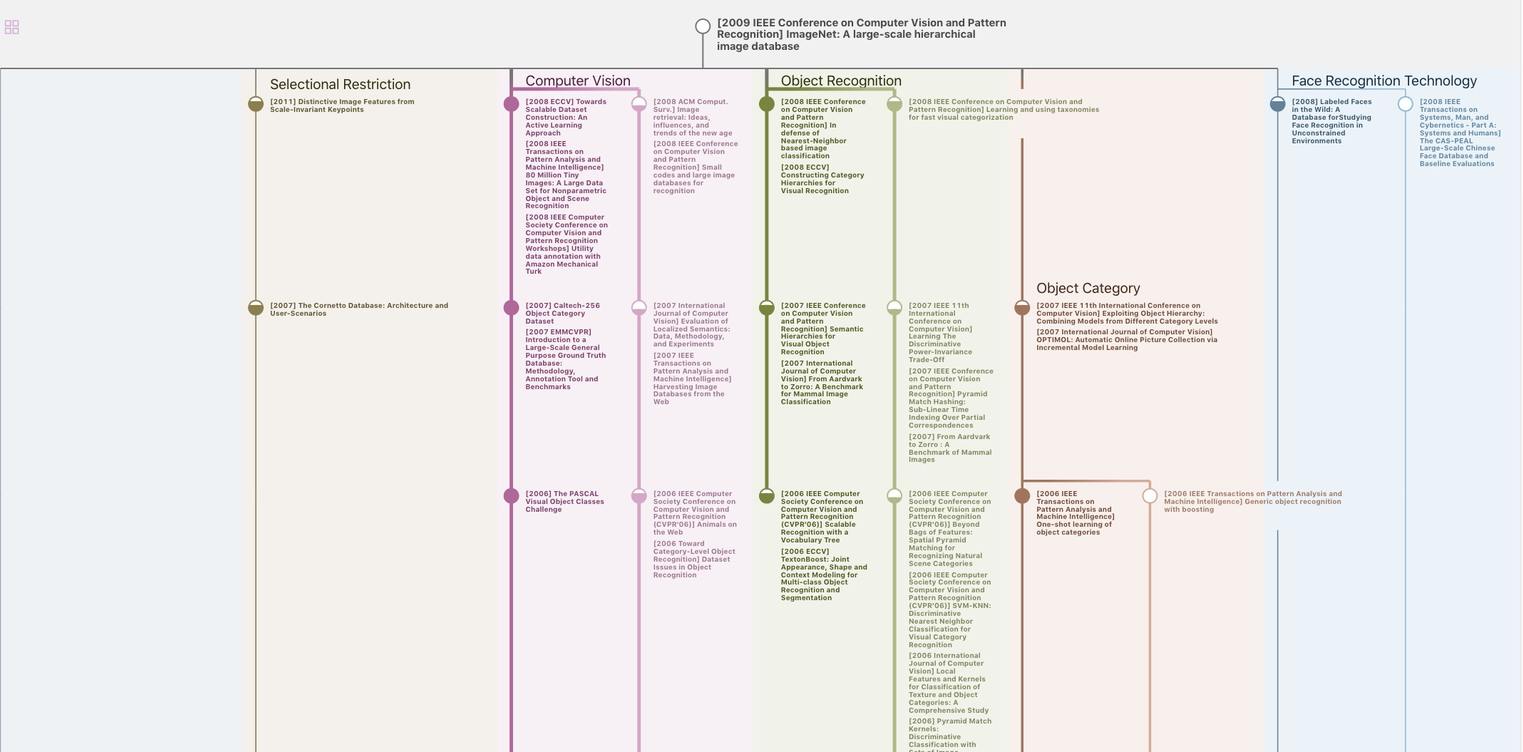
生成溯源树,研究论文发展脉络
Chat Paper
正在生成论文摘要