Learning with $\ell^{0}$-Graph: $\ell^{0}$-Induced Sparse Subspace Clustering
arXiv: Learning(2015)
摘要
Sparse subspace clustering methods, such as Sparse Subspace Clustering (SSC) cite{ElhamifarV13} and $ell^{1}$-graph cite{YanW09,ChengYYFH10}, are effective in partitioning the data that lie in a union of subspaces. Most of those methods use $ell^{1}$-norm or $ell^{2}$-norm with thresholding to impose the sparsity of the constructed sparse similarity graph, and certain assumptions, e.g. independence or disjointness, on the subspaces are required to obtain the subspace-sparse representation, which is the key to their success. Such assumptions are not guaranteed to hold in practice and they limit the application of sparse subspace clustering on subspaces with general location. In this paper, we propose a new sparse subspace clustering method named $ell^{0}$-graph. In contrast to the required assumptions on subspaces for most existing sparse subspace clustering methods, it is proved that subspace-sparse representation can be obtained by $ell^{0}$-graph for arbitrary distinct underlying subspaces almost surely under the mild i.i.d. assumption on the data generation. We develop a proximal method to obtain the sub-optimal solution to the optimization problem of $ell^{0}$-graph with proved guarantee of convergence. Moreover, we propose a regularized $ell^{0}$-graph that encourages nearby data to have similar neighbors so that the similarity graph is more aligned within each cluster and the graph connectivity issue is alleviated. Extensive experimental results on various data sets demonstrate the superiority of $ell^{0}$-graph compared to other competing clustering methods, as well as the effectiveness of regularized $ell^{0}$-graph.
更多查看译文
AI 理解论文
溯源树
样例
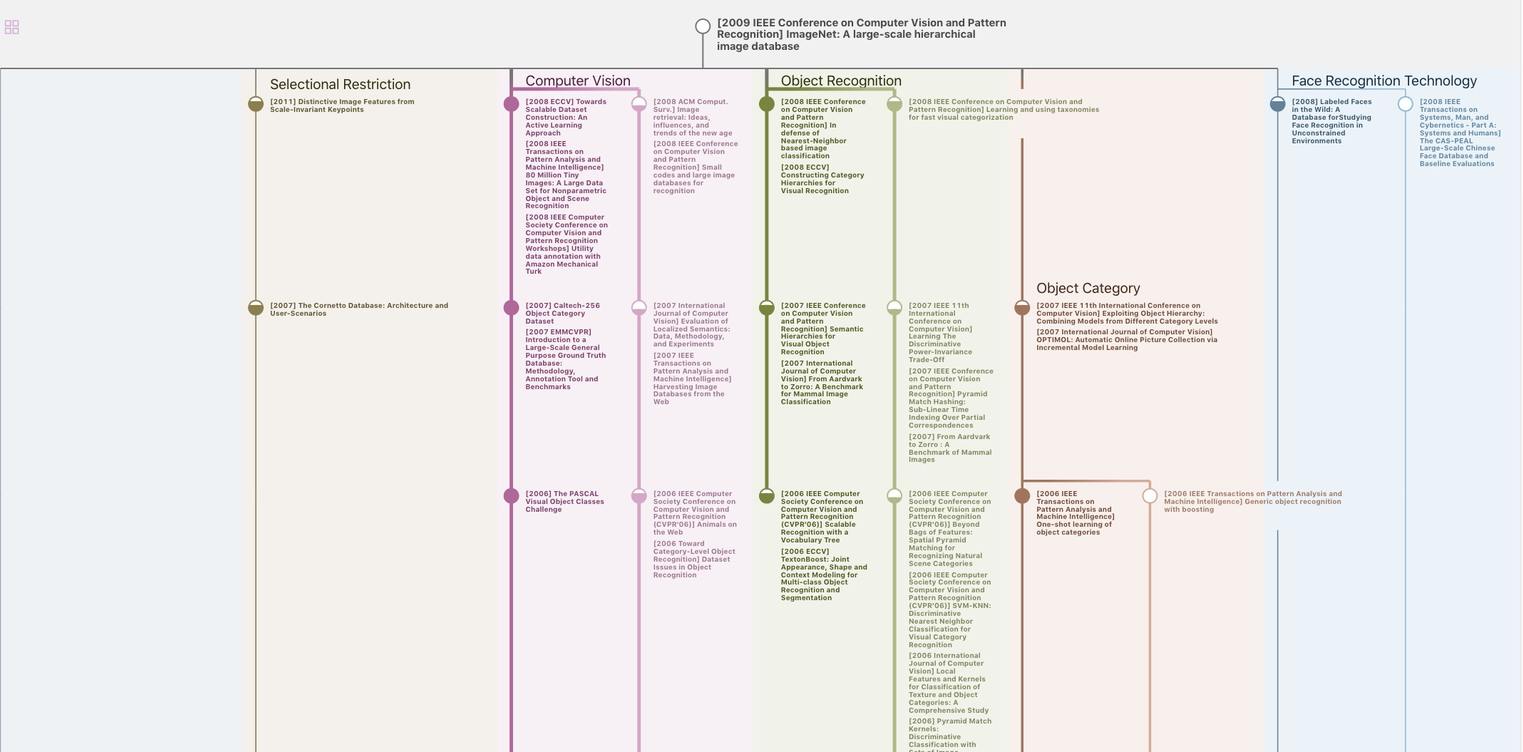
生成溯源树,研究论文发展脉络
Chat Paper
正在生成论文摘要