Data Augmentation Using Learned Transformations For One-Shot Medical Image Segmentation
2019 IEEE/CVF CONFERENCE ON COMPUTER VISION AND PATTERN RECOGNITION (CVPR 2019)(2019)
摘要
Image segmentation is an important task in many medical applications. Methods based on convolutional neural networks attain state-of-the-art accuracy; however, they typically rely on supervised training with large labeled datasets. Labeling medical images requires significant expertise and time, and typical hand-tuned approaches for data augmentation fail to capture the complex variations in such images.We present an automated data augmentation method for synthesizing labeled medical images. We demonstrate our method on the task of segmenting magnetic resonance imaging (MRI) brain scans. Our method requires only a single segmented scan, and leverages other unlabeled scans in a semi-supervised approach. We learn a model of transformations from the images, and use the model along with the labeled example to synthesize additional labeled examples. Each transformation is comprised of a spatial deformation field and an intensity change, enabling the synthesis of complex effects such as variations in anatomy and image acquisition procedures. We show that training a supervised segmenter with these new examples provides significant improvements over state-of-the-art methods for one-shot biomedical image segmentation.
更多查看译文
关键词
Medical,Biological and Cell Microscopy,Image and Video Synthesis
AI 理解论文
溯源树
样例
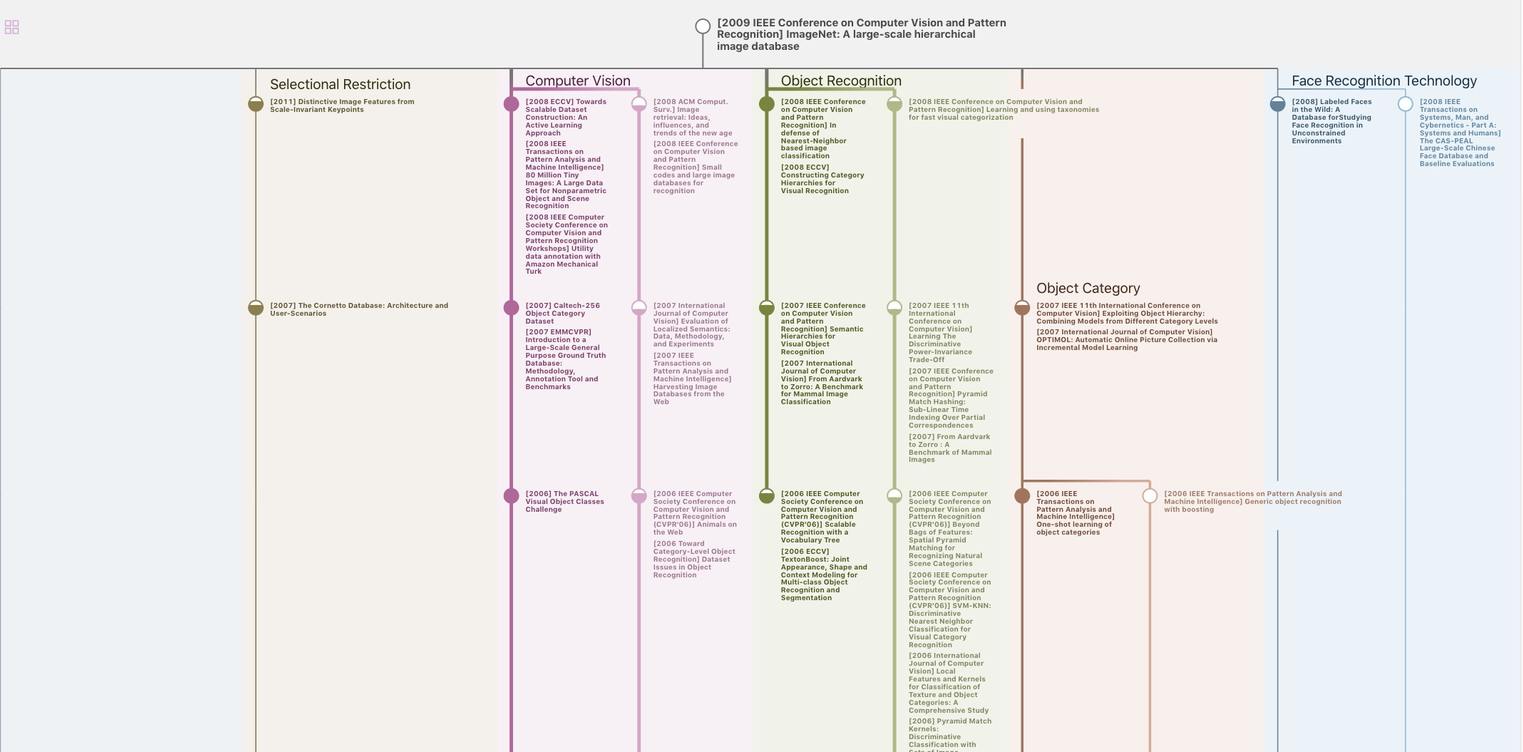
生成溯源树,研究论文发展脉络
Chat Paper
正在生成论文摘要