On the Performance of $k$ -Anonymity Against Inference Attacks With Background Information
IEEE Internet of Things Journal(2019)
摘要
Internet of Things (IoT) applications bring in a great convenience for human’s life, but users’ data privacy concern is the major barrier toward the development of IoT.
${k}$
-anonymity is a method to protect users’ data privacy, but it is presently known to suffer from inference attacks. Thus far, existing work only relies on a number of experimental examples to validate
${k}$
-anonymity’s performance against inference attacks, and thereby lacks of a theoretical guarantee. To tackle this issue, in this paper we propose the first theoretical foundation that gives a nonasymptotic bound on the performance of
${k}$
-anonymity against inference attacks, taking into consideration of adversaries’ background information. The main idea is to first quantify adversaries’ background information, and from the point of the view of adversaries, classify users’ data into four kinds: 1) independent with unknown data values; 2) local dependent with unknown data values; 3) independent with certain known data values; and 4) local dependent with certain known data values. We then move one step further, theoretically proving the bound on the performance of
${k}$
-anonymity corresponding to each of the four kinds of users’ data through cooperating with the noiseless privacy. We argue that such a theoretical foundation links
${k}$
-anonymity with noiseless privacy, theoretically proving
${k}$
-anonymity provides noiseless privacy. Additionally, this paper theoretically explains why
${k}$
-anonymity is vulnerable to inference attacks using the modified Stein method. Simulations on real check-in dataset from the location-based social network have validated our results. We believe that this paper can bridge the gap between design and evaluation, enabling a designer to construct a more practical
${k}$
-anonymity technique in real-life scenarios to resist inference attacks.
更多查看译文
关键词
Data privacy,Internet of Things,Sensitivity,Syntactics,Privacy,Electronic mail,Industries
AI 理解论文
溯源树
样例
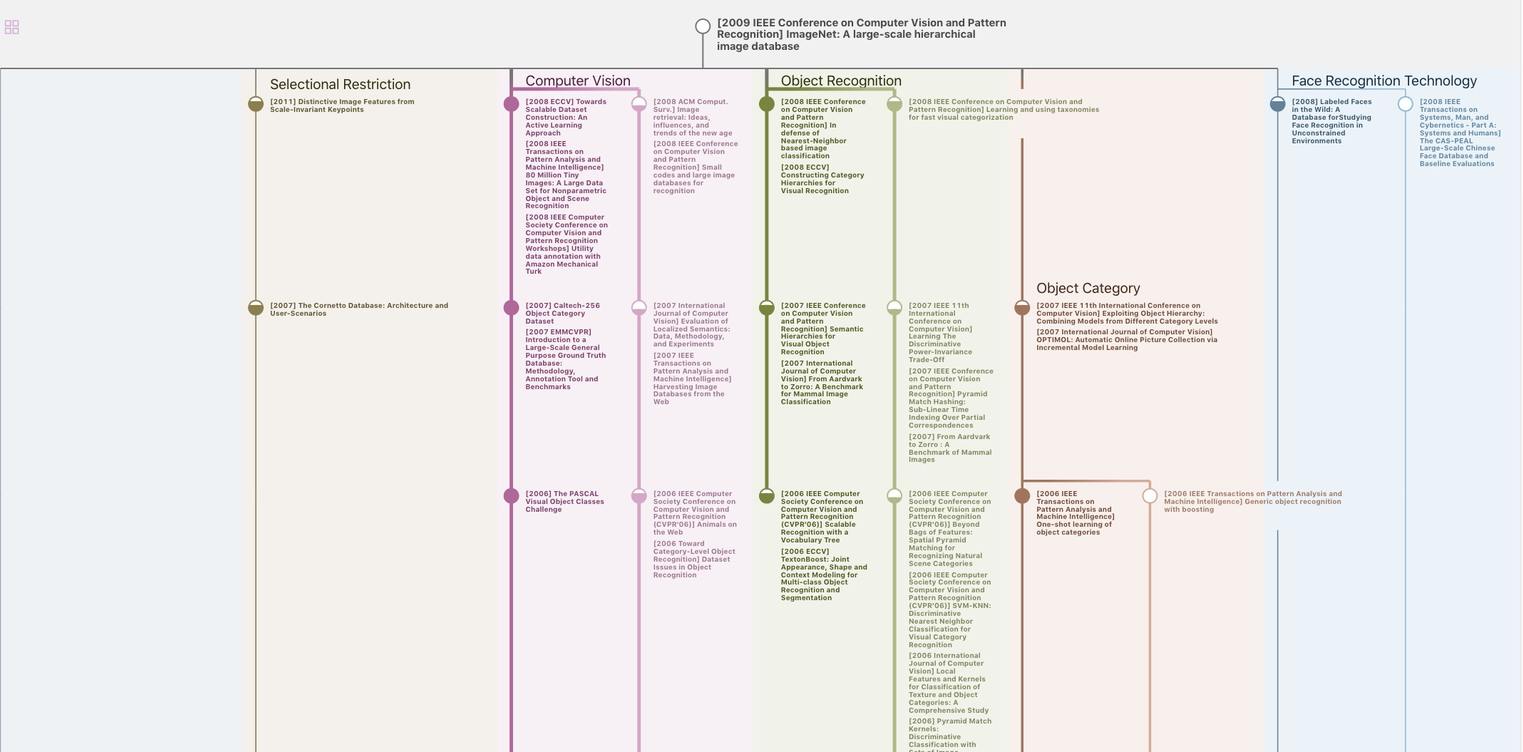
生成溯源树,研究论文发展脉络
Chat Paper
正在生成论文摘要