Constrained likelihood for reconstructing a directed acyclic Gaussian graph.
BIOMETRIKA(2019)
摘要
Directed acyclic graphs are widely used to describe directional pairwise relations. Such relations are estimated by reconstructing a directed acyclic graph's structure, which is challenging when the ordering of nodes of the graph is unknown. In such a situation, existing methods such as the neighbourhood and search-and-score methods have high estimation errors or computational complexities, especially when a local or sequential approach is used to enumerate edge directions by testing or optimizing a criterion locally, as a local method may break down even for moderately sized graphs. We propose a novel approach to simultaneously identifying all estimable directed edges and model parameters, using constrained maximum likelihood with nonconvex constraints. We develop a constraint reduction method that constructs a set of active constraints from super-exponentially many constraints. This, coupled with an alternating direction method of multipliers and a difference convex method, permits efficient computation for large-graph learning. We show that the proposed method consistently reconstructs identifiable directions of the true graph and achieves the optimal performance in terms of parameter estimation. Numerically, the method compares favourably with competitors. A protein network is analysed to demonstrate that the proposed method can make a difference in identifying the network's structure.
更多查看译文
关键词
Acyclicity,Constraint reduction,Directional relation,Nonconvex minimization
AI 理解论文
溯源树
样例
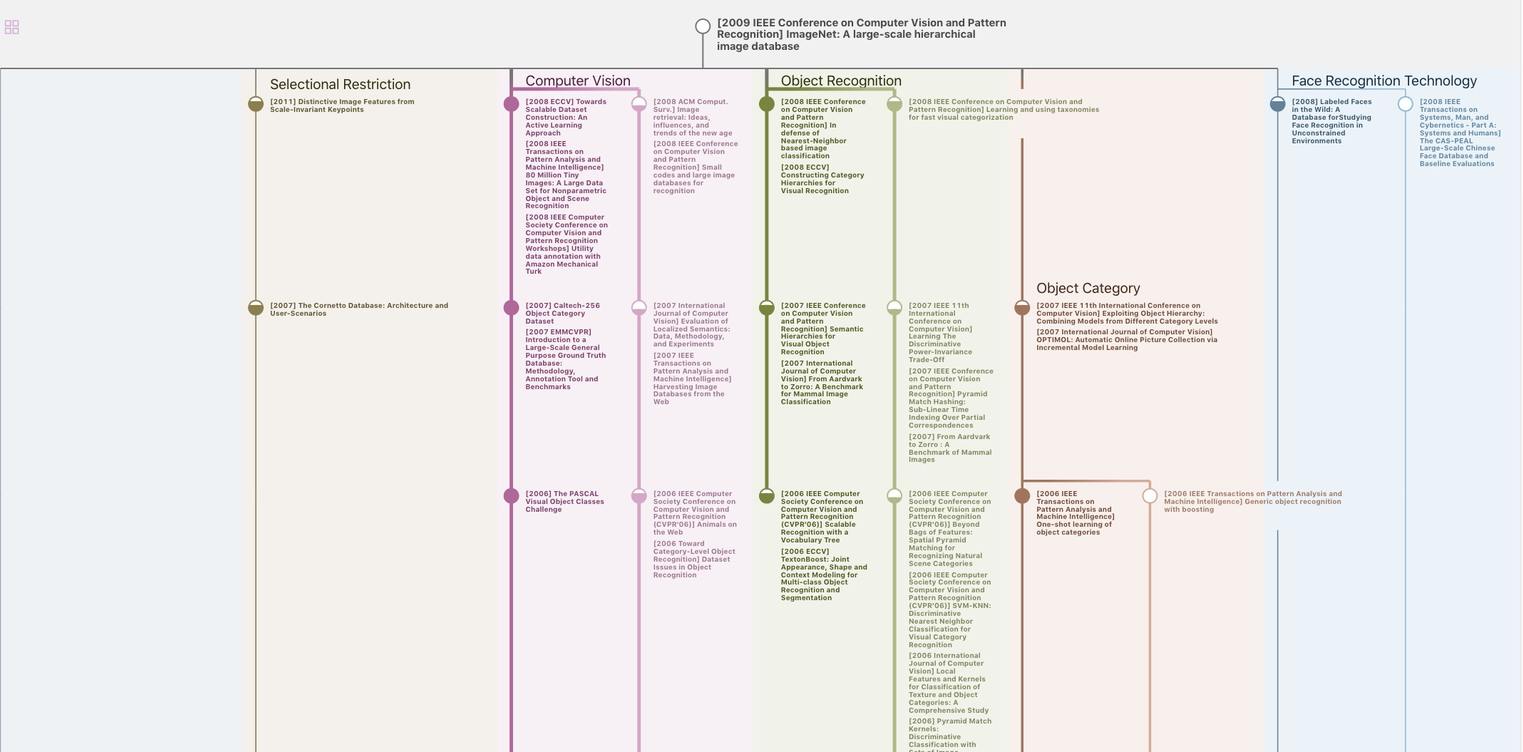
生成溯源树,研究论文发展脉络
Chat Paper
正在生成论文摘要