A Hybrid Deep Learning And Handcrafted Feature Approach For Cervical Cancer Digital Histology Image Classification
INTERNATIONAL JOURNAL OF HEALTHCARE INFORMATION SYSTEMS AND INFORMATICS(2019)
摘要
Cervical cancer is the second most common cancer affecting women worldwide but is curable if diagnosed early. Routinely, expert pathologists visually examine histology slides for assessing cervix tissue abnormalities. A localized, fusion-based, hybrid imaging and deep learning approach is explored to classify squamous epithelium into cervical intraepithelial neoplasia (CIN) grades for a dataset of 83 digitized histology images. Partitioning the epithelium region into 10 vertical segments, 27 handcrafted image features and rectangular patch, sliding window-based convolutional neural network features are computed for each segment. The imaging and deep learning patch features are combined and used as inputs to a secondary classifier for individual segment and whole epithelium classification. The hybrid method achieved a 15.51% and 11.66% improvement over the deep learning and imaging approaches alone, respectively, with a 80.72% whole epithelium CIN classification accuracy, showing the enhanced epithelium CIN classification potential of fusing image and deep learning features.
更多查看译文
关键词
Cervical Cancer, Clinical Decision Support Systems, Convolutional Neural Networks, Data Fusion, Deep Learning, Feature Extraction, Image Classification
AI 理解论文
溯源树
样例
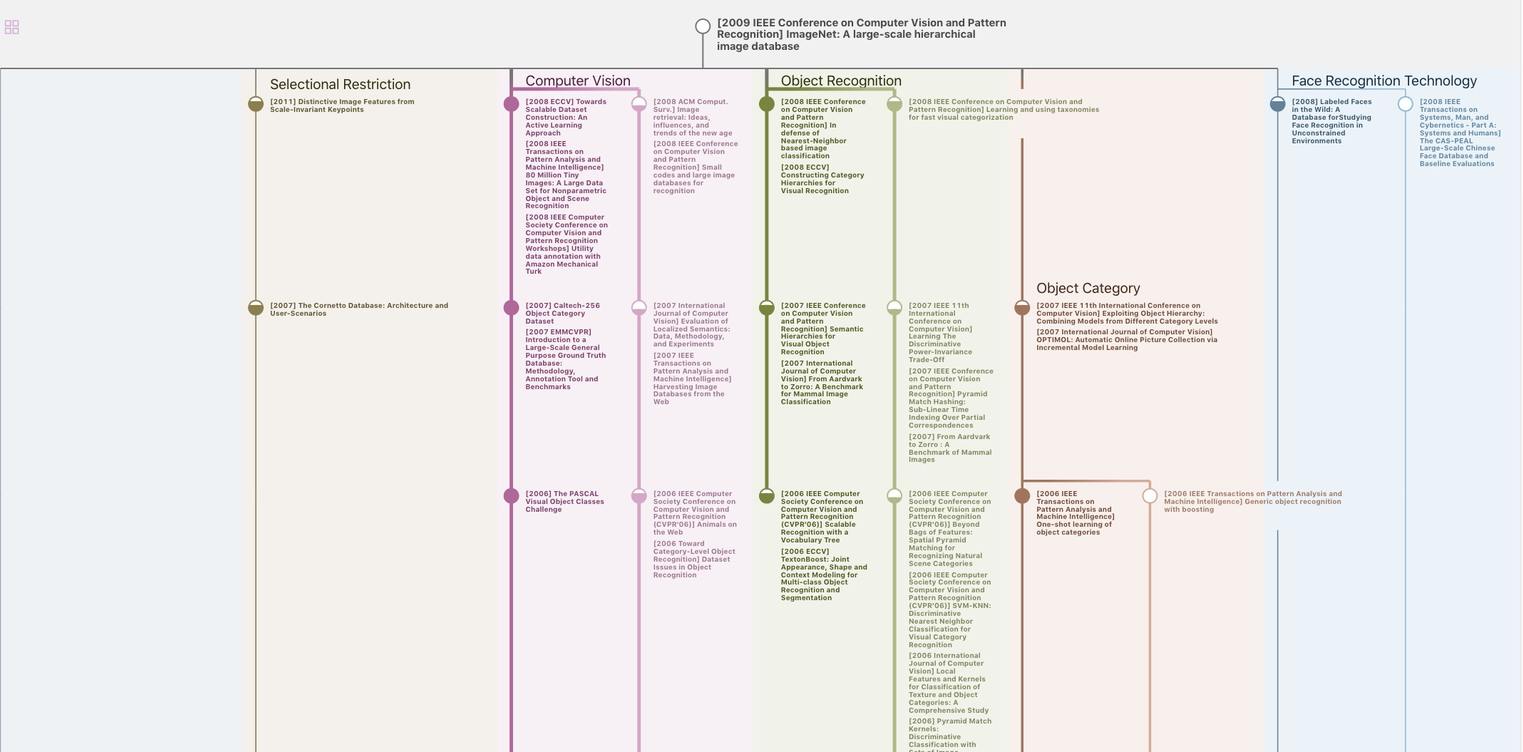
生成溯源树,研究论文发展脉络
Chat Paper
正在生成论文摘要