A Deep Learning Model For Automated Sleep Stages Classification Using Psg Signals
INTERNATIONAL JOURNAL OF ENVIRONMENTAL RESEARCH AND PUBLIC HEALTH(2019)
摘要
Sleep disorder is a symptom of many neurological diseases that may significantly affect the quality of daily life. Traditional methods are time-consuming and involve the manual scoring of polysomnogram (PSG) signals obtained in a laboratory environment. However, the automated monitoring of sleep stages can help detect neurological disorders accurately as well. In this study, a flexible deep learning model is proposed using raw PSG signals. A one-dimensional convolutional neural network (1D-CNN) is developed using electroencephalogram (EEG) and electrooculogram (EOG) signals for the classification of sleep stages. The performance of the system is evaluated using two public databases (sleep-edf and sleep-edfx). The developed model yielded the highest accuracies of 98.06%, 94.64%, 92.36%, 91.22%, and 91.00% for two to six sleep classes, respectively, using the sleep-edf database. Further, the proposed model obtained the highest accuracies of 97.62%, 94.34%, 92.33%, 90.98%, and 89.54%, respectively for the same two to six sleep classes using the sleep-edfx dataset. The developed deep learning model is ready for clinical usage, and can be tested with big PSG data.
更多查看译文
关键词
sleep stages, classification, deep learning, CNNs, polysomnography (PSG)
AI 理解论文
溯源树
样例
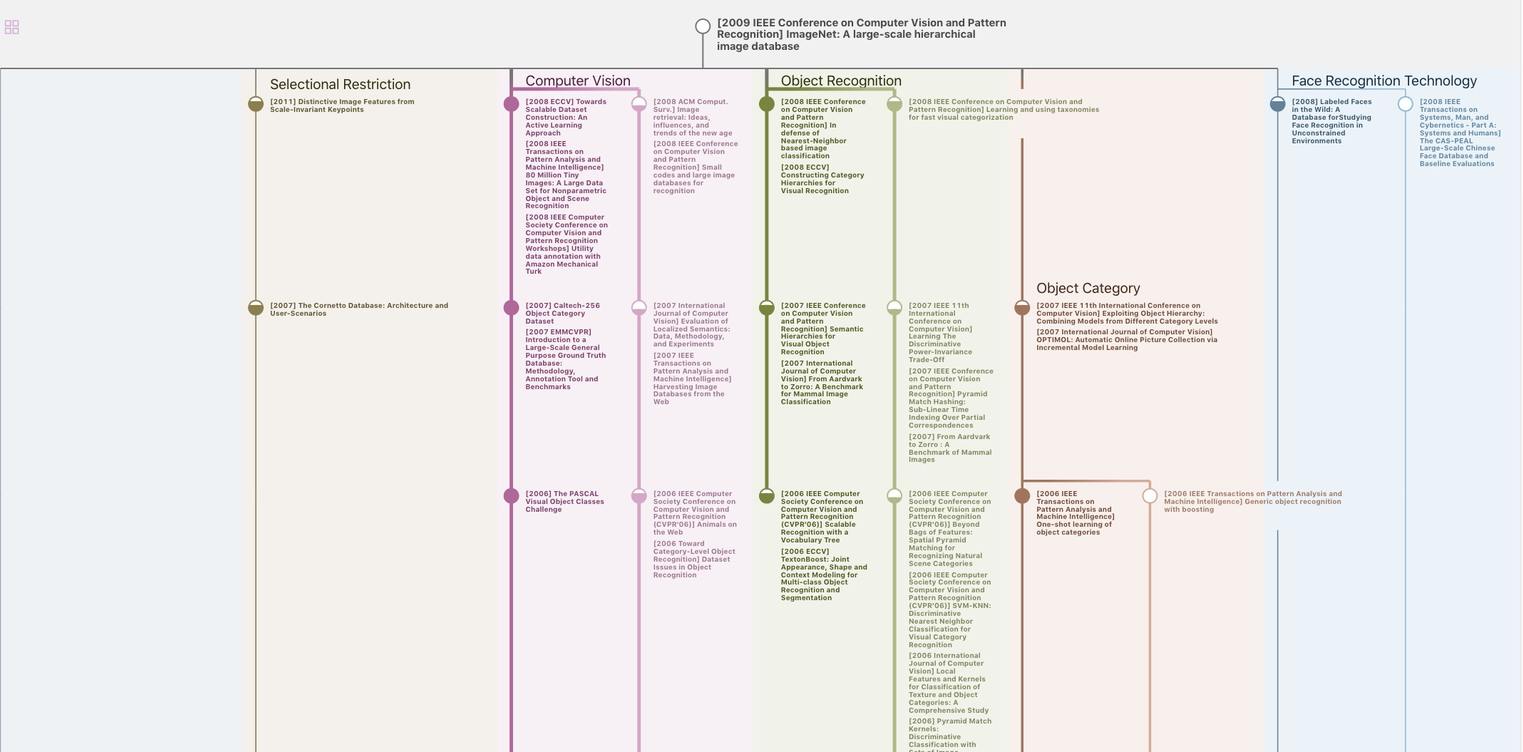
生成溯源树,研究论文发展脉络
Chat Paper
正在生成论文摘要