De Novo Molecular Design by Combining Deep Autoencoder Recurrent Neural Networks with Generative Topographic Mapping.
JOURNAL OF CHEMICAL INFORMATION AND MODELING(2019)
摘要
Here we show that Generative Topographic Mapping (GTM) can be used to explore the latent space of the SMILES-based autoencoders and generate focused molecular libraries of interest. We have built a sequence-to-sequence neural network with Bidirectional Long Short-Term Memory layers and trained it on the SMILES strings from ChEMBL23. Very high reconstruction rates of the test set molecules were achieved (>98%), which are comparable to the ones reported in related publications. Using GTM, we have visualized the autoencoder latent space on the two-dimensional topographic map. Targeted map zones can be used for generating novel molecular structures by sampling associated latent space points and decoding them to SMILES. The sampling method based on a genetic algorithm was introduced to optimize compound properties "on the fly". The generated focused molecular libraries were shown to contain original and a priori feasible compounds which, pending actual synthesis and testing, showed encouraging behavior in independent structure-based affinity estimation procedures (pharmacophore matching, docking).
更多查看译文
关键词
generative topographic mapping,molecular
AI 理解论文
溯源树
样例
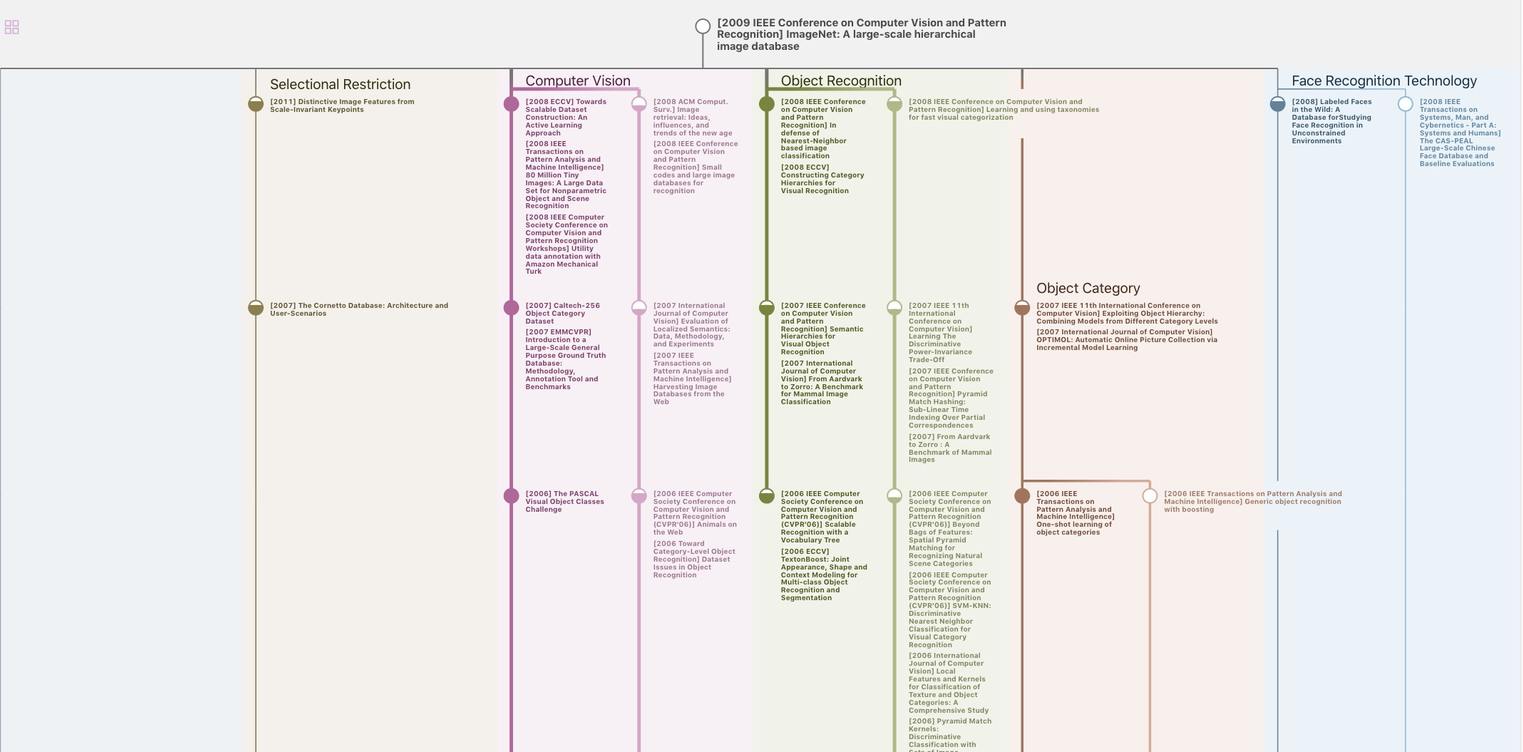
生成溯源树,研究论文发展脉络
Chat Paper
正在生成论文摘要