Beyond Word2vec: An approach for urban functional region extraction and identification by combining Place2vec and POIs.
Computers, Environment and Urban Systems(2019)
摘要
The actual functions of a region may not reflect the intent of the original zoning scheme from planners. To identify the actual urban functional regions, numerous methods have been proposed with computational advancement. Specifically, remote sensing by image recognition, geodemographic classification, social sensing with big data and geo-text mining techniques have been widely applied. Points-of-interest (POIs) are one of the most common open-access data type used to extract information pertaining to functional zones. However, previous works have either lost sight or did not make full use of the spatial interactions that can be extracted from POIs due to model limitations in the context of geographical space. In this research, we introduced an approach that detects functional regions at the scale of a neighborhood area (NA) by combining POI data and a simplified Place2vec model, which is theorized from the first law of geography. First, the POI-based spatial context is constructed by using the nearest neighbor approach. Then, we can increase the number of training tuples (tcenter,tcontext) based on the weight derived from the distance between the POI tcenter and POI tcontext. Next, high-dimensional characteristic vectors of the POIs are extracted by using the skip-gram training framework. By summarizing the POI vectors at the NA level, we employ a K-means clustering model to cluster the functional regions. Compared with other probabilistic topic models (PTMs) and Word2vec, the Place2vec-based approach obtained the highest mean reciprocal rank value (MRR-SWP=0.356, MRR-SLC=0.401, MRR-SJC=0.433, and MRR-SLin=0.421) in terms of similarity capturing performance and functional region identification accuracy (OA=0.7424). The research has important implications to urban planning and governance.
更多查看译文
关键词
Urban Functional Regions,Place2vec,POIs,Geo-Text Mining,Machine Learning
AI 理解论文
溯源树
样例
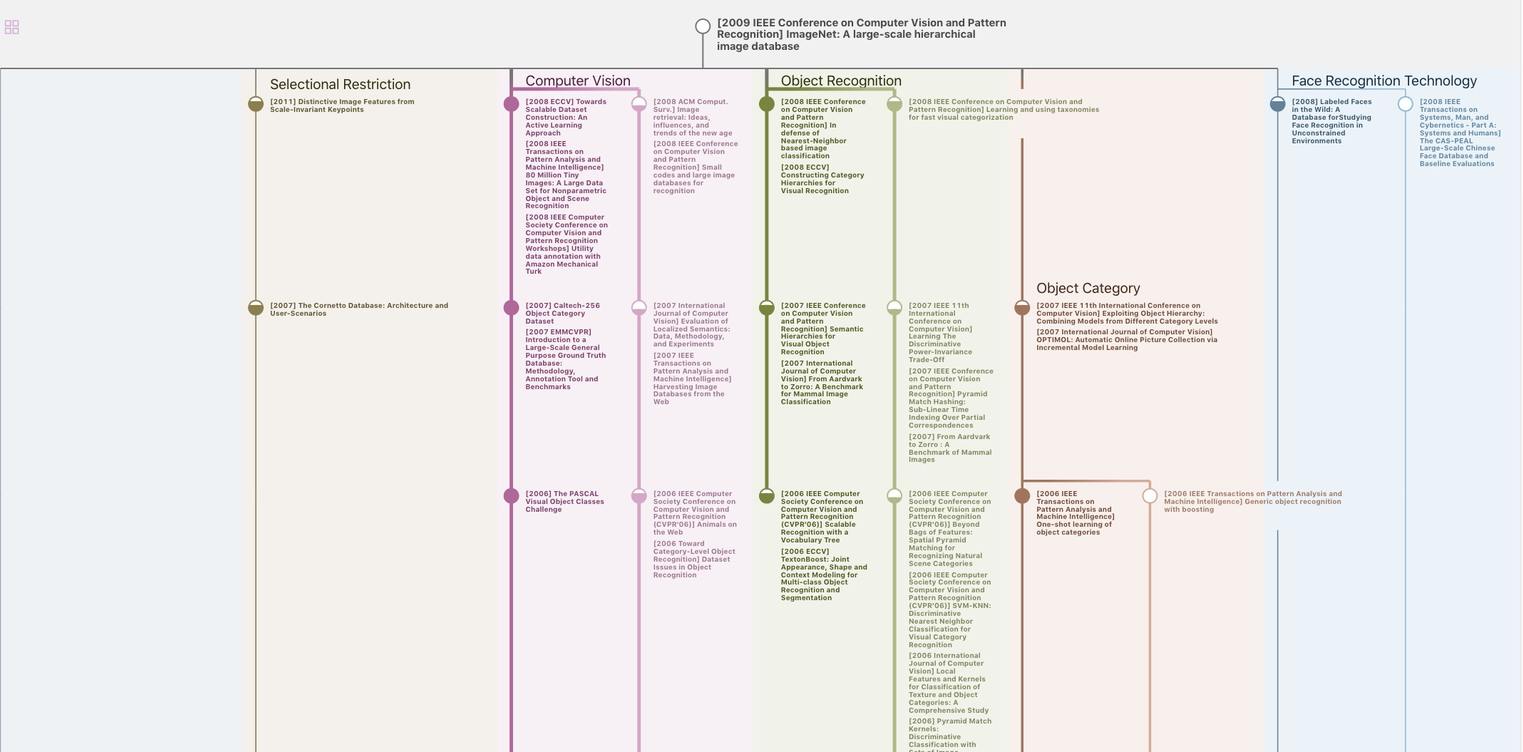
生成溯源树,研究论文发展脉络
Chat Paper
正在生成论文摘要