Automated classification of hand movements using tunable-Q wavelet transform based filter-bank with surface electromyogram signals.
Future Generation Computer Systems(2019)
摘要
To perform basic hand movements, a hand amputee person needs an exoskeleton prosthetic hand (EPH). The EPH can be controlled through electroencephalogram (EEG) or electromyogram (EMG) signals. The EMG signals are preferred as they are acquired from surface of forearm and termed as surface EMG (sEMG). It is very challenging to design the control section for EPH. It should be able to classify different hand movements accurately based on the acquired sEMG signals. Also the sEMG signals must be acquired from minimum number of electrodes to make EPH cost-effective. In this paper, we have proposed a novel technique to classify the basic hand movements. The method proposed in this paper applies tunable-Q wavelet transform (TQWT) based filter-bank (TQWT-FB) for decomposition of cross-covariance of sEMG (csEMG) signals. Then, Kraskov entropy (KRE) features are extracted and ranked. The proposed method is tested on the data obtained from five subjects and achieved the average classification accuracy (CA) of 98.55% using k-nearest neighbour (k-NN) classifier. Therefore, our developed prototype is available for further validation using larger diverse data.
更多查看译文
关键词
Hand amputee,Exoskeleton prosthetic hand,Surface electromyogram signals,Tunable-Q wavelet transform,k-nearest neighbours
AI 理解论文
溯源树
样例
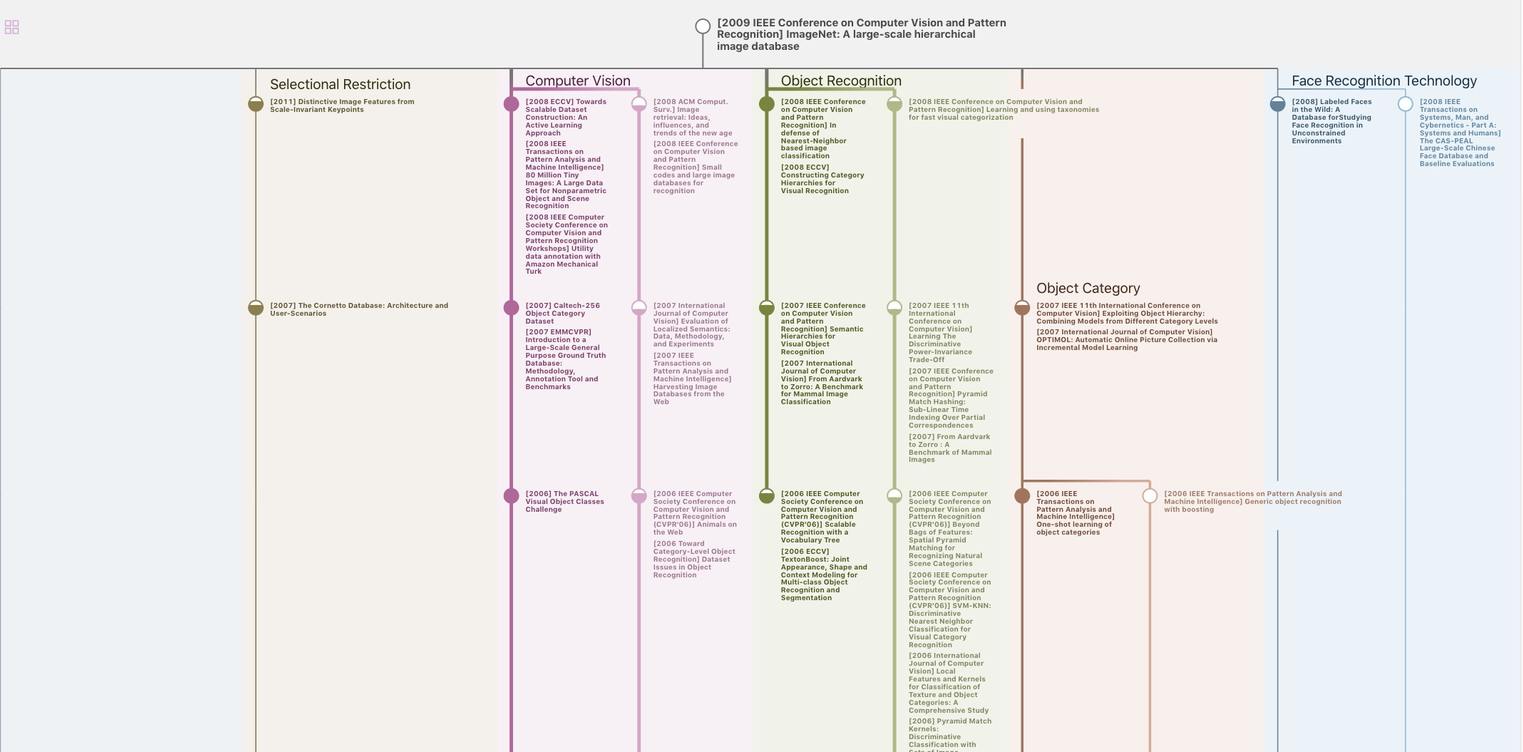
生成溯源树,研究论文发展脉络
Chat Paper
正在生成论文摘要