ARSAC: Efficient model estimation via adaptively ranked sample consensus.
Neurocomputing(2019)
摘要
RANSAC is a popular robust model estimation algorithm in various computer vision applications. However, the speed of RANSAC declines dramatically as the inlier rate of the measurements decreases. In this paper, a novel Adaptively Ranked Sample Consensus(ARSAC) algorithm is presented to boost the speed and robustness of RANSAC. The algorithm adopts non-uniform sampling based on the ranked measurements to speed up the sampling process. Instead of a fixed measurement ranking, we design an adaptive scheme which updates the ranking of the measurements, to incorporate high quality measurements into sample at high priority. At the same time, a geometric constraint is proposed during sampling process to select measurements with scattered distribution in images, which could alleviate degenerate cases in epipolar geometry estimation. Experiments on both synthetic and real-world data demonstrate the superiority in efficiency and robustness of the proposed algorithm compared to the state-of-the-art methods.
更多查看译文
关键词
RANSAC,Robust model estimation,Efficiency,Adaptively ranked measurements,Non-uniform sampling,Geometric constraint
AI 理解论文
溯源树
样例
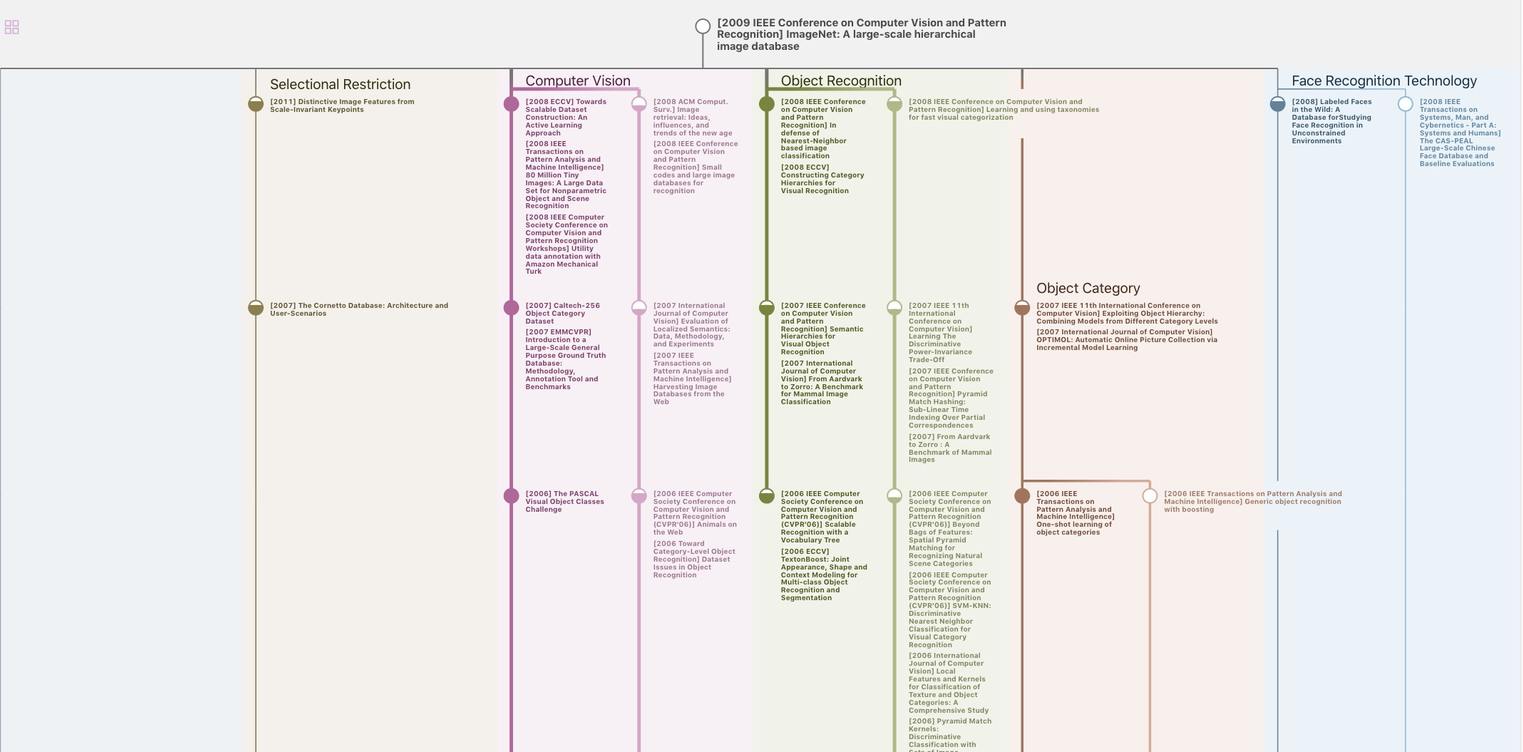
生成溯源树,研究论文发展脉络
Chat Paper
正在生成论文摘要