"Stream loss": ConvNet learning for face verification using unlabeled videos in the wild.
Neurocomputing(2019)
摘要
Face recognition tasks have seen a significantly improved performance due to ConvNets. However, less attention has been given to face verification from videos. This paper makes two contributions along these lines. First, we propose a method, called stream loss, for learning ConvNets using unlabeled videos in the wild. Second, we present an approach for generating a face verification dataset from videos in which the labeled streams can be created automatically without human annotation intervention. Using this approach, we have assembled a widely scalable dataset, FaceSequence, which includes 1.5M streams capturing ∼ 500K individuals. Using this dataset, we trained our network to minimize the stream loss. The network achieves accuracy comparable to the state-of-the-art on the LFW and YTF datasets with much smaller model complexity. We also fine-tuned the network using the IJB-A dataset. The validation results show competitive accuracy compared with the best previous video face verification results.
更多查看译文
关键词
Convolutional neural network,Face verification,Loss learning,Video stream
AI 理解论文
溯源树
样例
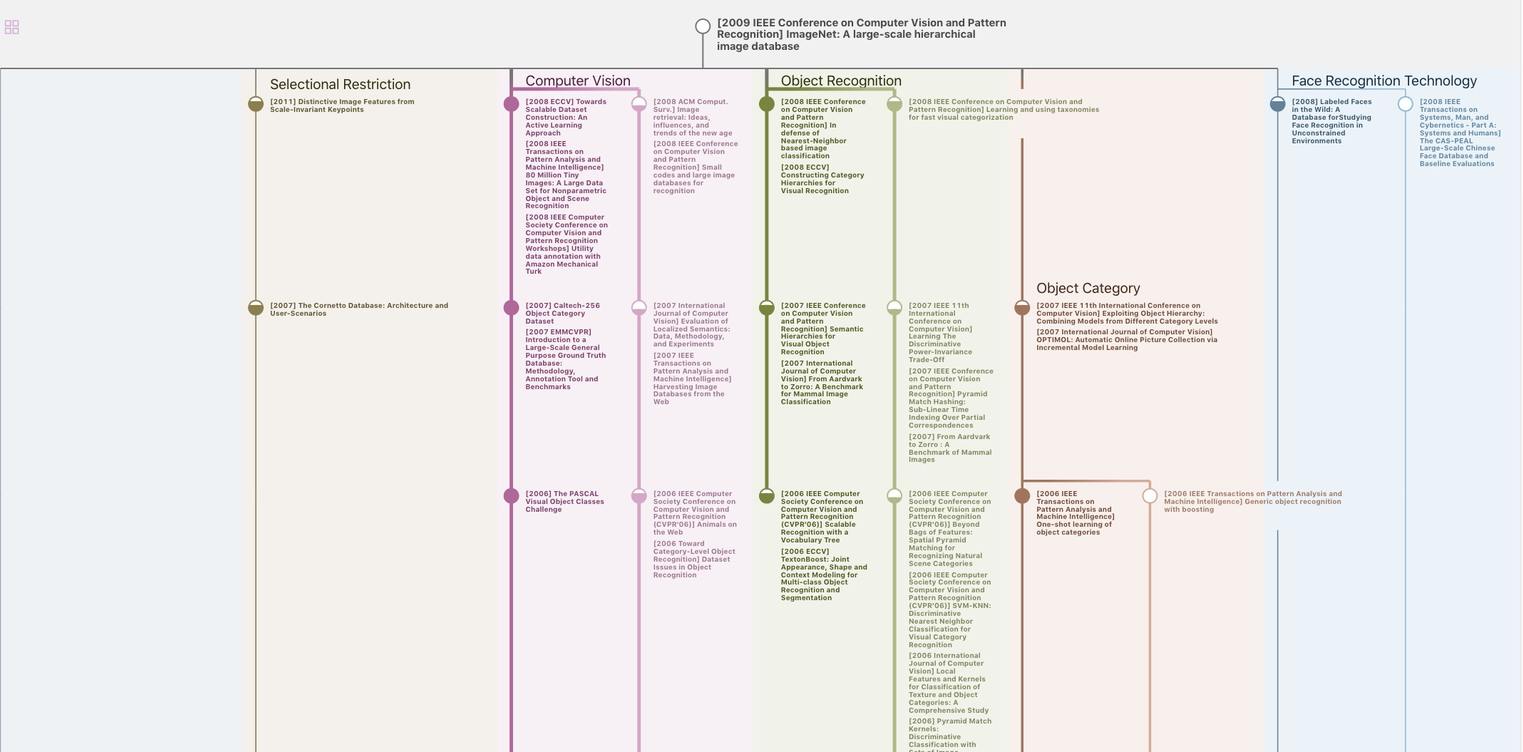
生成溯源树,研究论文发展脉络
Chat Paper
正在生成论文摘要