Popup: reconstructing 3D video using particle filtering to aggregate crowd responses
Proceedings of the 24th International Conference on Intelligent User Interfaces(2019)
摘要
Collecting a sufficient amount of 3D training data for autonomous vehicles to handle rare, but critical, traffic events (e.g., collisions) may take decades of deployment. Abundant video data of such events from municipal traffic cameras and video sharing sites (e.g., YouTube) could provide a potential alternative, but generating realistic training data in the form of 3D video reconstructions is a challenging task beyond the current capabilities of computer vision. Crowdsourcing the annotation of necessary information could bridge this gap, but the level of accuracy required to obtain usable reconstructions makes this task nearly impossible for non-experts. In this paper, we propose a novel hybrid intelligence method that combines annotations from workers viewing different instances (video frames) of the same target (3D object), and uses particle filtering to aggregate responses. Our approach can leveraging temporal dependencies between video frames, enabling higher quality through more aggressive filtering. The proposed method results in a 33% reduction in the relative error of position estimation compared to a state-of-the-art baseline. Moreover, our method enables skipping (self-filtering) challenging annotations, reducing the total annotation time for hard-to-annotate frames by 16%. Our approach provides a generalizable means of aggregating more accurate crowd responses in settings where annotation is especially challenging or error-prone.
更多查看译文
关键词
3D reconstruction, answer aggregation, autonomous vehicle, crowdsourcing, human computation, particle filter
AI 理解论文
溯源树
样例
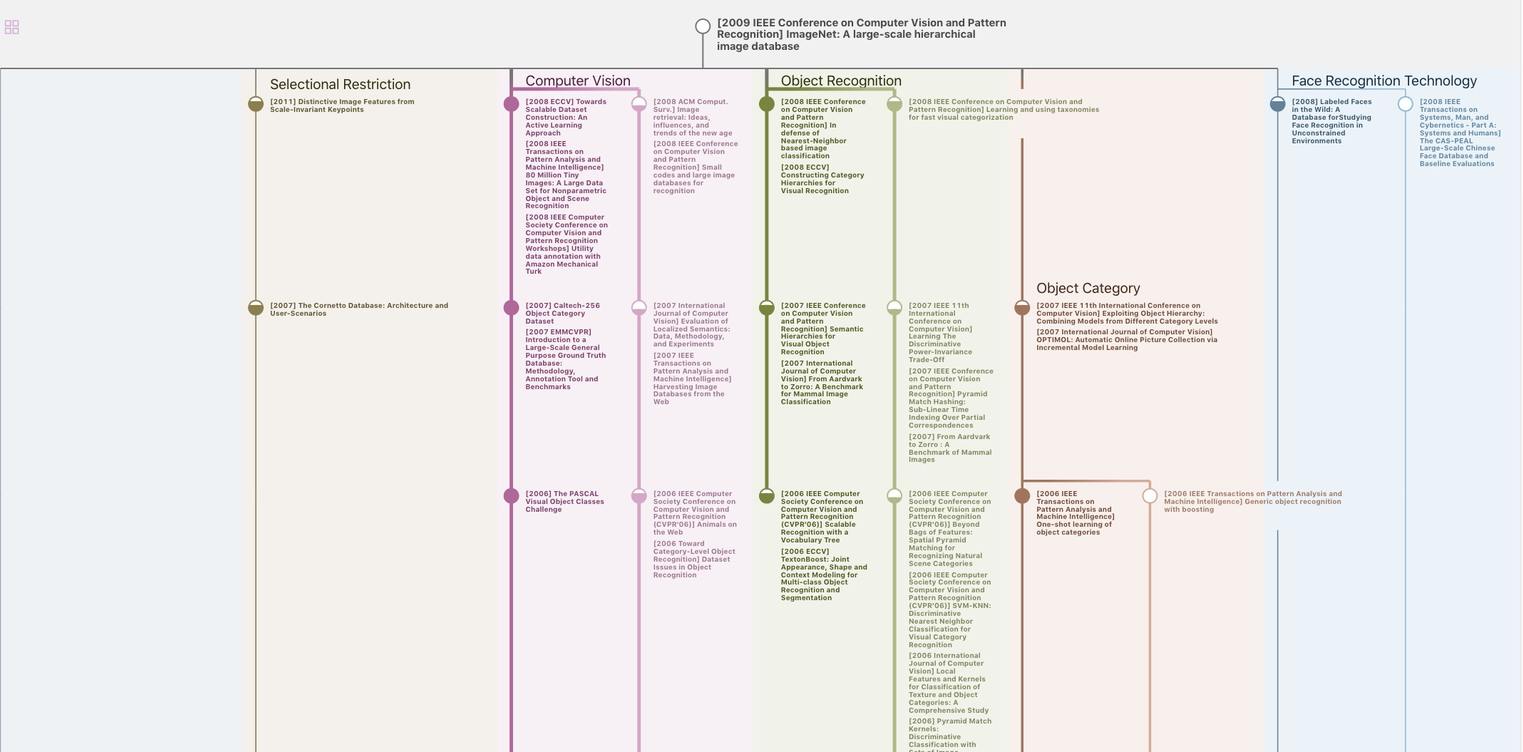
生成溯源树,研究论文发展脉络
Chat Paper
正在生成论文摘要